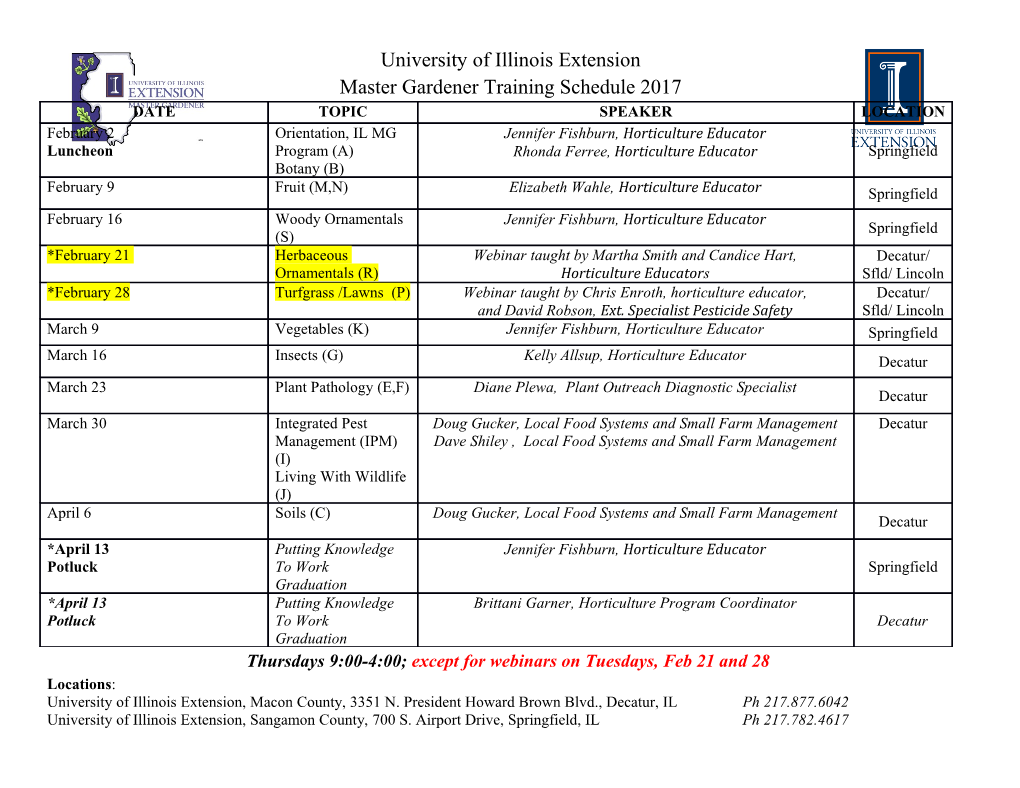
Word Transduction for Addressing the OOV Problem in Machine Translation for Similar Resource-Scarce Languages Shashikant Sharma and Anil Kumar Singh IIT (BHU), Varanasi, India fshashikant.sharma.cse12, [email protected] Abstract different communities1. Most of these languages are resource-scarce and therefore very little or no Similar languages have a large number work has been done on these languages. Lan- of cognate words which can be exploited guage is one of the major factors responsible for to deal with Out-Of-Vocabulary (OOV) digital divide between urban and rural areas due words problem. This problem is especially to prevalence of information technology in urban severe for resource-scarce languages. We areas (Dubey and Devanand, 2013). Therefore, propose a method for ‘word transduction’ removing this language barrier is crucial to the for addressing this problem. We take ad- growth of the society as well as for bridging the vantage of the fact that, although it is dif- digital divide. ficult to prepare sentence aligned parallel Hindi has several ‘dialects’ (often called sub- corpus for such languages, it is much eas- languages) spread over the entire Hindi speak- ier to prepare ‘parallel’ list of word pairs ing region commonly known as the Hindi-Belt. which are cognates and have similar pro- Bhojpuri is one of the seven Hindi sub-languages nunciations. We can try to learn pronunci- (other six include Awadhi, Braj, Haryanvi, Bun- ations (or orthographic representations) of deli, Bagheli and Kannauji) (Mishra and Bali, OOV words from such a parallel list. This 2011). Although the designation of Bhojpuri as a could be done by using phrase-based ma- language or simply as a dialect of Hindi is a topic chine translation (PBMT). We show that, of debate2, it is closely related to Hindi and bor- for small amount of data, a model based rows many words from Hindi, either directly or on weighted rewrite rules for phoneme with some phonological (and thus, orthographic) chunks outperforms a PBMT-based ap- changes. Besides, both use the Devanagari script proach. An additional point that we make for all official purposes, which (like major In- is that word transduction can also be used dian languages, Indo-Aryan and Dravidian) has to borrow words from another similar lan- evolved from the ancient Brahmi script (Sproat, guage and adapt them to the phonology of 2002; Sproat, 2003). In spite of having more the target language. than 33 million speakers3, Bhojpuri is a resource- 1 Introduction scarce language due to lack of resources such as machine readable dictionaries, WordNet and any Current research in the field of Automatic Speech standard parallel corpus, which makes the devel- Recognition (ASR) and Machine Translation opment of machine translation (MT) systems very (MT) tends to focus on the language pairs that challenging. For the same reason, Statistical Ma- have a large amount of data available. This is be- chine Translation is not feasible as it requires a cause the quality of these systems is dependent 1 on the amount and quality of the training data http://www.censusindia.gov.in/Census_ Data_2001/Census_Data_Online/Language/ used. As a result, many such systems between gen_note.html (relatively) resource-rich languages, such as En- 2http://ncictt.com/index.php/articles/ glish, Hindi, and Urdu are available. However 42-bhojpuri-a-dialect-of-hindi 3http://www.censusindia.gov.in/Census_ in a country like India, there are 122 major lan- Data_2001/Census_Data_Online/Language/ guages and over 1599 other languages spoken by Statement1.aspx 56 Proceedings of the 13th International Conference on Finite State Methods and Natural Language Processing, pages 56–63, Umea,˚ Sweden, 4–6 September 2017. c 2017 Association for Computational Linguistics https://doi.org/10.18653/v1/W17-4007 large parallel corpus. Alternatively, direct MT is The representation of speech using a sequence more suitable for closely related languages (Hajicˇ of phonetic symbols is defined as transcription. et al., 2000). Hindi has a phonetic writing system, i.e., there is Therefore, preparation of sufficient amount of very little distinction between its transcription and data for NLP tools like MT systems seems very pronunciation. Therefore, it is reasonable to as- difficult in the near future. Any such system, due sume that words in Hindi and Bhojpuri are spelled to lack of resources, faces low-coverage issues due or transcribed in the same way as they are pro- to the presence of unknown (Out-Of-Vocabulary nounced (Choudhury, 2003). This property makes or OOV) words. the mapping of Devanagari letters to International Phonetic Alphabet (IPA) symbols very easy. IPA To address this issue, we propose a ‘word trans- is organized in such a way that each symbol on a duction’ approach, which can show noticeable im- chart can be visualized as a hierarchical structure provement in inter-dialectal translation by trans- of features (Peter Ladefoged, 1988). It is possi- ducing OOV words. We define the term word ble to decomposes letters in the IPA representation transduction as the conversion of words from one into the building block of sounds (features). We source language to another closely related target have used the IPA representation of both source language such that pronunciation and meaning are (Hindi) and target (Bhojpuri) language. For in- similar. This can have two aspects. One is cognate stance, k is a single Devnagari alphabet but is generation, while the other is adapting borrowed equivalent to k^ + a, i.e., k has the inherent vowel words from the source language to the target lan- a which is easily reflected in its IPA representa- guage such that it matches the phonology of the tion(/k@/). target language. In other words, word transduc- The major contributions of this paper are: tion can be seen as transliteration (Denoual and Lepage, 2006; Finch and Sumita, 2009) in the • We propose a phoneme chunk based method same script to incorporate phonological changes for word transduction which transduces between a pair of closely related languages. words of Hindi to its closely related language Since the problem of machine transliteration Bhojpuri. Using the method described in this can also be viewed as the process of machine paper, we try to predict Bhojpuri pronuncia- translation at the character level, we have used tion from its corresponding Hindi word. This the popular phrase-based SMT (Statistical Ma- method is adapted from extensively reported chine Translation) system Moses between Hindi- earlier work on similar problems. Bhojpuri word pairs as the baseline system. SMT • We also show that proposed phoneme chunk requires a bilingual parallel training data, a lan- based method for word transduction performs guage model (LM), a translation model (TM) and better than the standard Statistical Machine a decoder. This method uses mapping of small text Translation as well as factored Statistical Ma- chunks (called ‘phrases’) without the utilization of chine Translation (Koehn and Hoang, 2007) any explicit linguistic information (such as mor- when applied on the same dataset using the phological, syntactic, or semantic). That is, it con- Moses decoder (Koehn et al., 2007). siders only the surface form of words to create a phrase table. Such additional information can be 2 Related Work incorporated in the form of ‘factors’, along with the words or characters (in case of transliteration) In a parallel corpus of a language and its dialect to improve the accuracy of standard SMT. (or closely related language), words can be cate- gorized into two categories based on their pronun- Surface form, along with these factors, creates ciation or orthographic form: factored representation of each word (Koehn et al., 2007; Koehn and Hoang, 2007). For factored • Word pairs having entirely different pronun- SMT, we augmented each letter of Devanagari ciations (and hence orthographic forms) in with their phonetic features (described in the next the two varieties. For example, ruaA (rauaa) section) to create its factored representation. This in Bhojpuri means aAp (aap, you-honorific) factored Statistical Machine Translation at charac- in Hindi. This type of word pairs share al- ter level performed better than our baseline SMT most no or very little phonetic and ortho- system. graphic similarity. Since our model utilizes 57 phonetic transition between two closely re- were used as a substitute for the proto-language. lated languages, this type of word-pairs are AFE, having more symbols than the normal not suitable for our model. set of phonemes (as in PBF), was applied to produce Finnish, Estonian and PBF directly and • Words having similar pronunciations (and unambiguously. hence their orthographic forms). A phonemic Singh (2006) formulated a phonetic model to study of Hindi and Bundeli (Acharya, 2015), represent relations between the sounds of In- mainly focusing on the prosodic features and dian languages and the letters or ‘akshars’ (or- the syllabic patterns of these two languages thographic syllables). It included phonetic fea- concluded that the borrowing of words from tures (Clements, 1985) mapped to each letter as Hindi to Bundeli generally follows certain well as a computational model to calculate the or- rules. For instance if a word in Hindi starts thographic and phonetic distance between given with y [ya], it is replaced by j [ja] in its Bun- pair of akshars, letters, words or strings. The deli equivalent as yjmAn [yajamaan] be- phonetic features described were mainly the ones comes jjmAn [jajamaan], ym nA [yamunaa] considered in modern phonetics, as well as some becomes jm nA [jamunaa] etc. This cate- orthographic features specific to Indian language gory of word-pairs is our main motivation be- scripts. The distance measure was based on the hind the work described in this paper. Our fact that phonetic features differentiate two sounds goal was to build a system which takes a (or akshars representing them) in a cascaded or hi- word as input in one language and returns its erarchical way.
Details
-
File Typepdf
-
Upload Time-
-
Content LanguagesEnglish
-
Upload UserAnonymous/Not logged-in
-
File Pages8 Page
-
File Size-