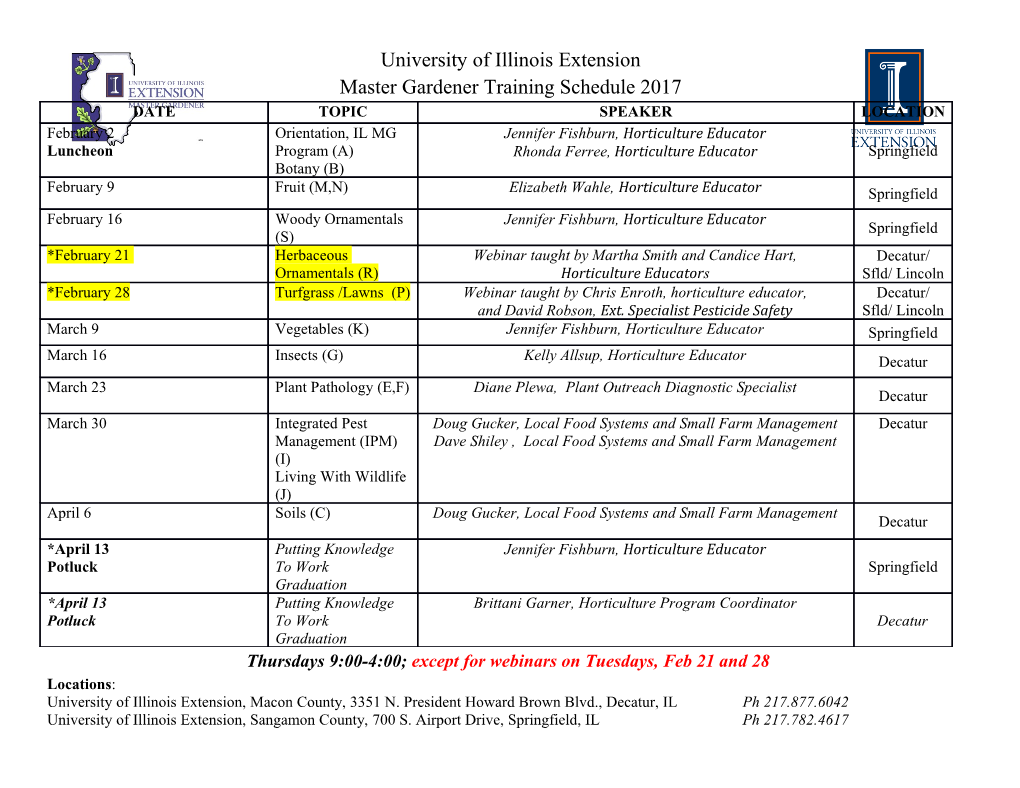
1 2 Summer Workshop on Distribution Theory & its Summability Perspective 50 45 40 35 30 25 Frequencies 20 15 10 5 0 | 16 17 18 18.9 20 21 22 Center of mass Amount of Drink Mix (in ounces) M. Kazım Khan Kent State University (USA) Place: Ankara University, Department of Mathematics Dates: 16 May - 27 May 2011 Supported by: The Scientific and Technical Research Council of Turkey (TUBITAK)¨ 4 Preface This is a collection of lecture notes I gave at Ankara University, department of math- ematics, during the last two weeks of May, 2011. I am greatful to Professor Cihan Orhan and the Scientific and Technical Research Council of Turkey (TUBITAK)¨ for the invitation and support. The primary focus of the lectures was to introduce the basic components of distribution theory and bring out how summability theory plays out its role in it. I did not assume any prior knowledge of probability theory on the part of the participants. Therefore, the first few lectures were completely devoted to building the language of probability and distribution theory. These are then used freely in the rest of the lectures. To save some time, I did not prove most of these results. Then a few lectures deal with Fourier inversion theory specifically from the summability perspective. The next batch consists of convergence concepts, where I introduce the weak and the strong laws of large numbers. Again long proofs were omitted. A noteable exception deals with the results that involve the uniformly in- tegrable sequence spaces. Since this is a new concept from summability perspective, I have tried to sketch some of the proofs. I must acknowledge the legendary Turkish hospitality of all the people I came to meet. As always, it was a pleasure visiting Turkey and I hope to have the chance to visit again. Mohammad Kazım Khan, Kent State University Kent, Ohio, USA. 6 List of Participants 1- AYDIN, Didem Ankara Universitesi¨ 2- AYGAR, Yelda Ankara Universitesi¨ 3- AYKOL, Canay Ankara Universitesi¨ 4- BAS¸CANBAZ TUNCA, G¨ulen Ankara Universitesi¨ 5- CAN, C¸a˘gla Ankara Universitesi¨ 6- CEBESOY, S¸erifenur Ankara Universitesi¨ 7- COS¸KUN, Cafer Ankara Universitesi¨ 8- C¸ETIN,˙ Nursel Ankara Universitesi¨ 9- DONE,¨ Ye¸sim Ankara Universitesi¨ 10- ERDAL, Ibrahim˙ Ankara Universitesi¨ 11- GUREL,¨ Ovg¨u¨ Ankara Universitesi¨ 12- IPEK,˙ Pembe, Ankara Universitesi¨ 13- KATAR, Deniz Ankara Universitesi¨ 14- ORHAN, Cihan Ankara Universitesi¨ 15- SAKAOGLU,˘ Ilknur˙ Ankara Universitesi¨ 16- SOYLU, Elis Ankara Universitesi¨ 17- S¸AHIN,˙ Nilay Ankara Universitesi¨ 18- TAS¸, Emre Ankara Universitesi¨ 19- UNVER,¨ Mehmet Ankara Universitesi¨ 20- YARDIMCI, S¸eyhmus Ankara Universitesi¨ 21- YILMAZ, Ba¸sar Ankara Universitesi¨ 22- YURDAKADIM,˙ Tu˘gba Ankara Universitesi¨ 8 CONTENTS 9 Ranks, Order Statistics & Records 75 10 Fourier Transforms 83 10.1Examples ................................. 83 11 Summability Assisted Inversion 89 Contents 12 General Inversion 97 12.1 Fourier&DirichletSeries . 99 13 Basic Limit Theorems 107 13.1 ConvergenceinDistribution . 108 13.2 ConvergenceinProbability&WLLN. 111 Preface 3 14AlmostSureConvergence&SLLN 117 List of Participants 5 15 The Lp Spaces & Uniform Integrability 127 Contents 5 15.1 UniformIntegrability. 132 List of Figures 9 16 Laws of Large Numbers 141 16.1 Subsequences & Kolmogorov Inequality . 142 1 Modeling Distributions 1 17 WLLN, SLLN & Uniform SLLN 151 1.1 Distributions ............................... 1 17.1 Glivenko-CantelliTheorem . 163 1.2 ProbabilitySpace&RandomVariables . 8 18 Random Series 169 2 ProbabilitySpaces&RandomVariables 11 18.1 Zero-OneLaws&RandomSeries . 169 3 Expectations 21 18.2 RefinementsofSLLN. 175 3.1 Properties of Lebesgue integral . 22 19 Kolmogorov’s Three Series Theorem 183 3.2 Covariance ................................ 23 20 The Law of Iterated Logarithms 189 4 Various Inequalities 27 4.1 Holder&Minkowski’sInequalities . 28 4.2 Jensen’sInequality ......... ......... ......... 30 5 Classification of Distributions 35 5.1 AbsoluteContinuity&Singularity . 41 6 Conditional Distributions 49 6.1 ConditionalExpectations . 52 7 ConditionalExpectations&Martingales 57 7.1 Properties of E(X Y ) .......................... 57 7.2 Martingales ................................| 59 8 Independence & Transformations 63 8.1 Transformations of Random Variables . 63 8.2 Sequences of Independent Random Variables . 68 8.3 GeneratingFunctions. 70 10 LIST OF FIGURES List of Figures 1.1 A Histogram for the Drink Mix Distribution. 4 1.2 Inverse Image of an Interval . 8 8.1 Inverse of a Distribution Function. 66 11.1 TriangularDensity . 95 12.1 Dirichlet Kernels for n = 5 and n =8. .................101 12.2 Fejer Kernels for T =5 and T =8....................103 12.3 Poisson Kernels for r = 0.8 and r = 0.9. ................105 14.1 Density of Random Harmonic Series . 124 2 Modeling Distributions (i) Distributions arising while measuring mass produced products. • (ii) Distributions arising in categorical populations. • (iii) Distribution of Stirling numbers. • (iv) Distribution of zeros of orthogonal polynomials. • (v) Distributional convergence of summability theory. Lecture 1 • (vi) Distributions of eigenvalues of Toeplitz matrices. • (vii) Maxwell’s law of ideal gas. • (viii) Distribution of primes. Modeling Distributions • (ix) The Fineman-Kac formula and partial differential equations. • Of course, this is just a tiny sample of topics from an enormous field. One obvious omission being the field of Schwartz’s distributions. This is purely because there A phenomenon when repeatedly observed gives rise to a distribution. In other are excellent books on the subject.1 We will, however, briefly visit this branch while words, a distribution is our way of capturing the variability in the phenomenon. discussing summability assisted Fourier inversion theory. Such distributions arise in almost all fields of endeavor. In social sciences they are used to keep tabs on social indicators, in finance they are used to study and qunatify Example - 1.1.1 - (Measurement distributions — accuracy of automatic the financial health of corporations and pricing various assets and derived securities filling machines) Kountry Times makes 20 ounce cans of lemonade drink mix. such as options and bonds. Data distributions appear in statistics. In mathematics Due to unknown random fluctuations, the actual fill weight of each can is rarely distributions of zeros of orthogonal polynomials appear and the distribution of equal to 20 oz. Here is a collection of fill weights of 200 randomly chosen cans. primes are fundamental entities. In natural sciences about one and a half centure ago Maxwell conjoured up a distribution to describe the speed of molecules in 18.3 19.4 18.8 19.6 19.8 17.7 18.2 20.1 17.2 18.8 19.0 18.6 18.0 18.9 19.1 17.2 17.3 19.4 18.6 20.5 20.8 19.9 ideal gas, which was later observed to be quite accurate. The genetic diversity 18.7 16.7 19.2 18.8 18.3 18.3 18.3 17.9 18.2 17.5 17.6 and its quantification is still in its infency in terms of discovering the underlying 19.7 20.5 19.5 18.6 19.9 19.3 18.5 19.9 18.7 20.3 19.2 distributions that it hides. 18.9 18.6 19.4 18.7 18.5 19.2 17.3 18.0 17.7 19.2 19.1 In this chapter we will collect the tools that are quite effective in studying 18.8 18.3 21.0 18.0 18.9 19.9 21.4 18.8 19.0 18.9 18.7 distributions. We will present the following basic notions. 18.9 19.2 17.6 20.0 19.5 19.4 18.3 19.9 18.4 18.3 18.6 19.4 17.7 18.8 17.8 19.2 18.6 20.2 19.0 18.3 18.3 19.0 Some examples of distributions, • 18.4 19.4 19.4 17.9 19.2 18.5 17.7 19.3 19.0 16.7 18.3 A framework by which distributions can be modeled, • 19.7 18.8 19.4 20.3 18.3 18.6 19.4 18.4 18.6 19.1 18.0 Transforms of distributions, such as moment generating functions and char- 18.8 18.3 18.7 19.1 17.8 17.5 17.0 19.4 19.2 19.8 18.6 • acteristic functions, 17.7 17.9 19.1 18.2 19.5 19.6 20.4 20.7 19.8 18.9 19.2 Conditional probabilities and conditional expectations. 17.8 21.0 17.5 17.9 18.5 21.1 19.8 18.3 20.2 17.4 18.8 • 18.5 19.7 19.0 18.3 19.3 18.8 18.1 17.8 19.1 20.1 19.9 These results will be used in the remainder of the book. 21.0 17.9 18.3 17.1 18.7 18.5 19.1 17.6 20.4 19.2 19.2 20.2 17.4 18.4 18.9 18.4 18.8 18.3 19.8 18.7 19.1 20.4 18.7 18.9 18.0 20.7 20.8 19.9 20.6 19.2 18.4 18.5 18.5 1.1 Distributions 18.4 19.9 17.9 19.4 19.2 20.4 19.7 17.5 19.0 17.9 18.4 19.7 19.1 Any characteristic, when repeatedly measured, yields a collection of measured/collected responses. The word “variable” is used for the characteristic that is being measured, In this example, the feature being measured is the fill weight (measured in ounces). since it may vary from measurement to measurement. The collection of all the mea- We see unexpectedly large amount of variability. The issue is: sured responses is called the “distribution” of the variable. Sometimes, the word “Does the distribution say anything about whether the advertised av- data is also used to refer to the distribution of the variable.
Details
-
File Typepdf
-
Upload Time-
-
Content LanguagesEnglish
-
Upload UserAnonymous/Not logged-in
-
File Pages103 Page
-
File Size-