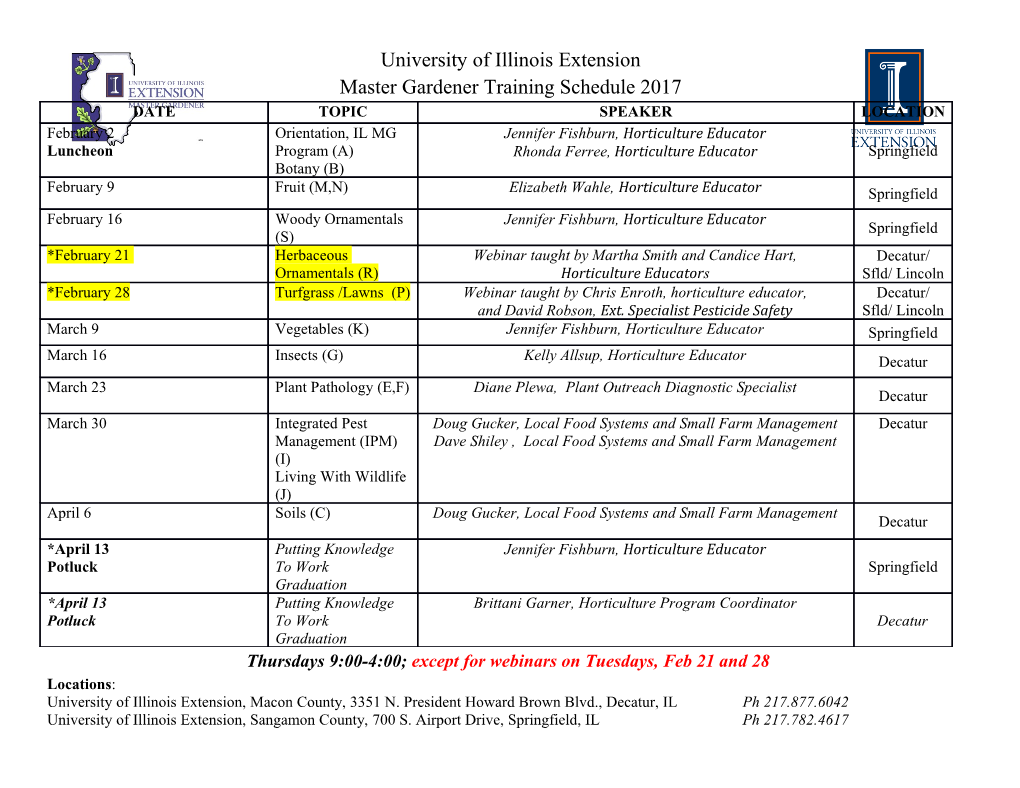
Choosing a Deep Learning Library There are a lot of them JesseBrizzi{.com,@gmail.com,@curalate.com} Who am I/What do I do? ● Research Engineer ○ Focus in Computer Vision and Machine learning ○ CS background ● Work on Image Intelligence Team @Curalate ○ E-Commerce SaaS ○ Platform to enable brands to find image based social media content to repurpose for e-commerce purposes. ○ Image Intelligence Team owns entire pipeline of researching new ML application to training, development, and then getting it into production. ○ Intelligent Product Tagging - technology that can analyze an image and use machine learning to identify specific products depicted within that image. Choosing a Deep Learning Library Choosing a Deep Learning Library at’s L’s a Neural Net? Choosing a Deep Learning Library at’s L’s a Neural Net? ● FCN - Fully Connected Network ○ Multilayer perceptron/fundamental neural net where each neuron is connect to all neurons in the previous layer of the network. ● CNN - Convolutional Neural Network ○ Neural net that uses convolutional layers, heavily used in Computer Vision applications. ● RNN - Recurrent Neural Network ○ Neural net that feeds its output back into itself to process the next input, heavily used in Natural Language Processing applications. ● LSTM - Long Short-Term Memory Recurrent Neural Net ○ Fancy RNNs that contain additional control over what output is passed to the next input. Choosing a Deep Learning Library Important Factors ● Academia vs Industry ○ Who is the target audience? ● Community support ○ Pretrained models? ○ Research paper repos? ○ How googleable are bugs and issues? ● Development speed/barriers for entry ○ Abstractions of low level concepts. ○ Documentation quality ○ Supported programming languages ○ The ability to Scale Choosing a Deep Learning Library Important Factors ● Codebase Quality ○ Is the code actively maintained? ●Performance ○ Benchmarks (oldish) https://arxiv.org/pdf/1608.07249.pdf ○ Performance does not scale very well on CPUs. 16 core CPUs are only slightly better than 4 or 8 core CPUs. ○ GPUs perform much better than many-core CPUs. ○ Scalability across multiple GPUs ○ Performance is also affected by the design of configuration files/implementation paradigm. Choosing a Deep Learning Library Important Factors ● Train to Production pipeline ○ Support for a fast to prototype language (python, R) and deployment in your production language (java/scala, c++, JS, whatever). ○ Train locally if you have the hardware vs training on pre-prepared, simplified cloud services. ○ Ability to run on different platforms ranging from mobile phones to massive server farms ○ Transfer your work to other libraries Choosing a Deep Learning Library Imperative vs Symbolic paradigms ● Dynamic Computation Graphing (Imperative Programming) ○ Are built at runtime which lets you use standard language statements. ○ At run time the system generation the graph structure. ○ Useful for when the graph structure needs to change at run time. ○ Makes debugging easy. ● Imperative programs tend to be more flexible ○ It’s easier to use native language features. ○ The graph can follow your programs logical control flow. Choosing a Deep Learning Library Imperative vs Symbolic paradigms ● Symbolic Programs Tend to be More Efficient ○ Both in terms of memory and speed. ○ Can safely reuse the memory for in-place computation. ○ Can also operation folding optimizations. ● Static Computation Graphing (Symbolic Paradigm) ○ Define the computation graph once, execute graph many times. ○ Can optimized the graph at the start ○ Good for fixed size Net (feed-forward, CNN) ● Easier to manage in terms of loading and resources Choosing a Deep Learning Library Libraries That People Should Know About Caffe ● IMO the first mainstream production ready lib. UC Berkeley ○ high performance and well tested C++ codebase. Watches: 2,241 Stars: 27,296 ● One of the first, and largest, model zoos. Forks: 16,454 ● Large community of open source research projects. Avg Issue Resolution: 3 Days Open issues: 13% ● Able to train a net from your data without writing any code. Symbolic Paradigm ● Good for feedforward networks, image processing, and for fine-tuning pretrained nets Research Citations (2014): 10,159 ● Main advantage was being first to market. Model zoo ● Can convert models to almost any other relevant lib. Choosing a Deep Learning Library Caffe ● Has bad design choices that are inherited from its original use case: UC Berkeley conventional CNN applications. Watches: 2,241 Stars: 27,296 ● Not good for recurrent networks Forks: 16,454 ● Does not support Auto differentiation Avg Issue Resolution: 3 Days Open issues: 13% ● Very verbose in layer and network definitions Symbolic Paradigm ○ the graph is treated as a collection of layers, as opposed to nodes of single tensor operations Research Citations (2014): 10,159 Model zoo Choosing a Deep Learning Library Keras ● A library that sits on top of other DL libs and provides a single, easy to use, high level interface. ● Very modular, minimal, readable, object oriented code. Keras ● Great for beginners, with great documentation Watches: 1,982 | Stars: 38,796 ● Lacks in optimizations Forks: 14,799 ● Supported backends Avg Issue Resolution: 23 Days Open issues: 24% ○ Tensorflow, Theano, CNTK, MXNet Symbolic Paradigm ● Can export your trained models into the backends format. ● Fork included in TensorFlow’s Python library. Model zoo ● Not as customizable Choosing a Deep Learning Library Tensorflow ● The current most popular option. Google ○ Largest active community Watches: 8,606 Stars: 121,864 ○ More open source projects and models. Forks: 72,545 Avg Issue Resolution: 8 Days ● Google’s attempt to build a single deep learning framework for Open issues: 16% everything deep learning related. Symbolic/Dynamic Paradigm ○ Built with massive distributed computing in mind (powers G-apps). Research Citations (2016): 6233 ○ Has mobile capabilities in the form of TensorFlow Mobile and Model zoo TensorFlow Light. ● TensorBoard is amazing for debugging and training. CNN Example Code (Keras R) ● TensorFlow Serving for prod deployments (python) CNN Example Code (Keras Py) ● A lot of documentation (official and 3rd party) CNN Example Code Choosing a Deep Learning Library Tensorflow ● Deep Google Cloud integration. Google ● Pretty low level (Keras and Sonnet help solve this) Watches: 8,606 Stars: 121,864 ● Most things outside of the core c/python library are “experimental” Forks: 72,545 Avg Issue Resolution: 8 Days ○ All of the APIs outside of the Python API are not covered by Open issues: 16% their API stability promises. Symbolic/Dynamic Paradigm ● Biggest issue with library is performance. Research Citations (2016): 6233 ○ TensorFlow is just slower and more of a resource hog when Model zoo compared to the other libraries. CNN Example Code (Keras R) ○ Other libs can perform twice as fast on typical deep net tasks. ○ Avoid for performant RNNs or LSTMs networks. CNN Example Code (Keras Py) ○ Worst at scaling efficiency. CNN Example Code Choosing a Deep Learning Library Torch/PyTorch ● Torch was one of the original academic Deepmind, NYU, IDIAP Facebook focused libs. ● Many maintainers went to work at Watches: 665 | Stars: 8,218 Watches: 1,197 | Stars: 25,450 Forks: 2,340 Forks: 6,044 Facebook and created PyTorch. Avg Issue Resolution: 69 Days Avg Issue Resolution: 6 Days Open issues: 34% Open issues: 24% ● They use the same underlying C lib. ○ Provide similar performance. Symbolic Paradigm Symbolic/Dynamic Paradigm ● They differ in Research Citations: 1,246 Research Citations: 879 ○ Interface (Lua vs Python) Model zoo Model zoo ○ Auto diff capabilities CNN Example Code ○ Paradigms Choosing a Deep Learning Library PyTorch ● PyTorch was made with the goal of fixing or modernizing Torch. Facebook ● Hybrid fronted for switching between paradigms. ● PyTorch also has its own visualization dashboard called Visdom. Watches: 1,197 | Stars: 25,450 Forks: 6,044 ● Probably should avoid if want to deploy into production. Avg Issue Resolution: 6 Days Open issues: 24% ○ Facebook maintains a separate lib targeted at developers, Caffe2. Symbolic/Dynamic Paradigm ○ Making changes to make PyTorch production ready. Research Citations: 879 ○ Caffe2 recently merged into PyTorch Model zoo ● Researchers tend to prefer PyTorch over Tensorflow CNN Example Code ○ Makes prototyping easy Choosing a Deep Learning Library MXNet ● Newer and growing option. Apache, Amazon ● Largest officially supported API selection. Watches: 1,180 | Stars: 16,450 ○ High compatibility and consistency. Forks: 5,889 Avg Issue Resolution: 40 Days ● Direct competitor to TensorFlow across all applications. Open issues: 13% ○ It can run on everything from a web browser, a mobile Symbolic/Dynamic Paradigm phone, to a massive distributed server farm. Research Citations: 712 ○ Amazon has found that you can get up to an 85% scaling Model zoo efficiency with MXNet. CNN Example Python Code ● Has its own serving framework and deep integration with AWS. CNN Example Code (Gluon) ● Also has its own Tensorboard forks. Choosing a Deep Learning Library MXNet Gluon ● Collaboration between AWS and Microsoft. ● Provides a clear, concise, and simple API for deep learning. ○ Full set of plug-and-play neural network building blocks. ■ predefined layers, optimizers, and initializers ○ Built in model zoo. ● Hybridization is awesome ○ Hybrid Symbolic/Dynamic graph functionality. ○ Offers benefits of both. ○ Can make Gluon 3x faster than PyTorch ● Great documentation for absolute beginners.
Details
-
File Typepdf
-
Upload Time-
-
Content LanguagesEnglish
-
Upload UserAnonymous/Not logged-in
-
File Pages44 Page
-
File Size-