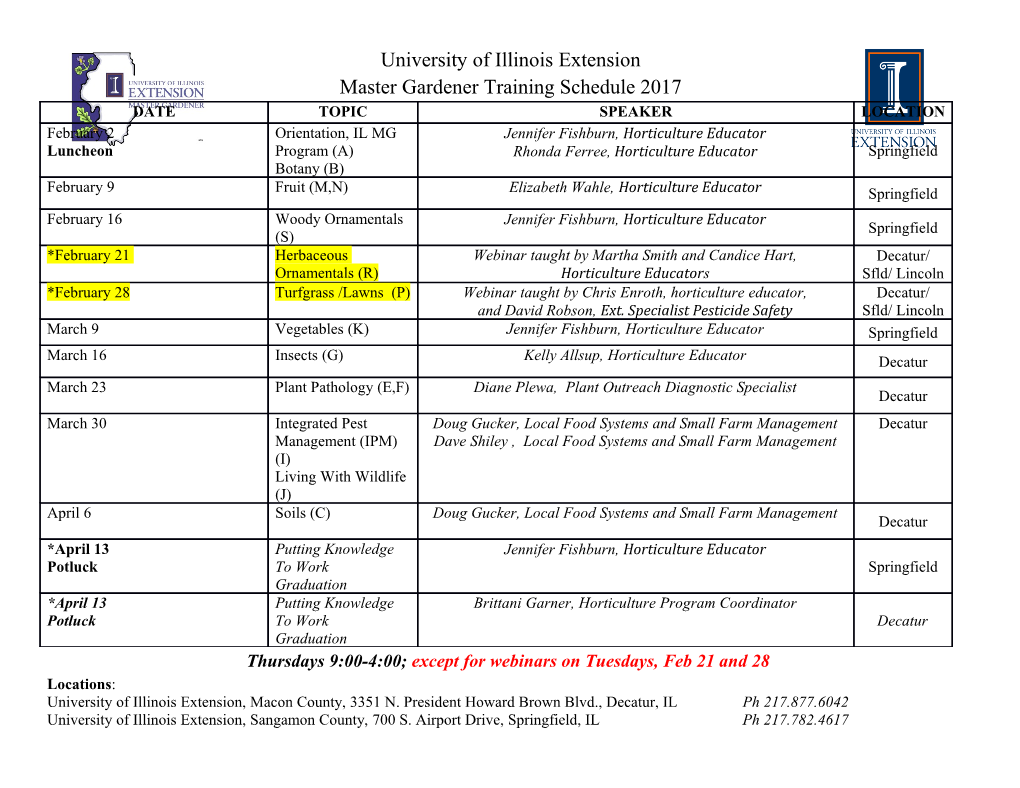
RESERVATION FOR OTHER BACKWARD CLASSES IN INDIAN CENTRAL GOVERNMENT INSTITUTIONS LIKE IITs, IIMs AND AIIMS – A STUDY OF THE ROLE OF MEDIA USING FUZZY SUPER FRM MODELS W. B. Vasantha Kandasamy Florentin Smarandache K. Kandasamy 2009 RESERVATION FOR OTHER BACKWARD CLASSES IN INDIAN CENTRAL GOVERNMENT INSTITUTIONS LIKE IITs, IIMs AND AIIMS – A STUDY OF THE ROLE OF MEDIA USING FUZZY SUPER FRM MODELS W. B. Vasantha Kandasamy e-mail: [email protected] web: http://mat.iitm.ac.in/~wbv www.vasantha.in Florentin Smarandache e-mail: [email protected] K. Kandasamy e-mail: [email protected] 2009 2 CONTENTS Dedication 5 Preface 6 Chapter One INTRODUCTION TO NEW SUPER FUZZY MODELS 7 1.1 Supermatrices and Fuzzy Supermatrices 7 1.2 Super Fuzzy Relational Maps 45 Chapter Two ANALYSIS OF THE ROLE OF MEDIA ON RESERVATION FOR OBC USING SUPER FUZZY MODELS 57 2.1 Brief Description of the Attributes Given by the Experts 58 2.2 Super Row FRM Model to Study the Role of Media on OBC Reservation 77 2.3 Super Fuzzy Mixed FRM Model to Study the Role of Media in Falsely Blaming the Government and Supporting Dr. Venugopal 88 2.4 Use of Super Column FRM Model to Study the Interrelation between the Government and Public 102 2.5 Analysis of Role of Media on Reservation for OBC using Fuzzy Cognitive Map 117 2.6 Observations based on this Analysis by Students and Experts through Seminars and Discussions 121 3 Chapter Three EXCERPT OF NEWS FROM PRINT MEDIA AND SUGGESTIONS AND COMMENTS BY THE EXPERTS 145 Chapter Four ANALYSIS SUGGESTIONS AND CONCLUSIONS BASED ON DISCUSSIONS, QUESTIONNAIRE, INTERVIEWS AND MATHEMATICAL MODELS 357 4.1 A View and Analysis by Group of Educationalists about Role of Media on OBC Reservation 357 4.1.1 Students Protests in Anti Reservation as covered by Media 359 4.1.2 Pro Reservation Protests of Students as Reported by the Media 364 4.1.3 Coverage Given by the Media about Other Anti Reservation Protests 367 4.1.4 The Pro Reservation Protests … Given by Media 370 4.1.5 Essays or Articles against Reservation in Print Media 372 4.2 The Present Functioning of the Media as Described by Experts 374 4.3 Analysis by Socio Scientists on Media and OBC Reservations 381 4.4 Origin of Reservations in India and Analysis by the Experts 404 4.5 Suggestions, Comments and Views 409 FURTHER READING 423 INDEX 453 ABOUT THE AUTHORS 455 4 DEDICATION We dedicate this book to Thanthai Periyar’s foremost follower and five-time Tamil Nadu Chief Minister Hon’ble Dr. Kalaignar for his uncompromising struggle to ensure social justice through 27% reservation for the Other Backward Classes (OBC) in Central Government-run higher educational institutions like the IITs, IIMs and AIIMS. 5 PREFACE The new notions of super column FRM model, super row FRM model and mixed super FRM model are introduced in this book. These three models are introduced specially to analyze the biased role of the print media on 27 percent reservation for the Other Backward Classes (OBCs) in educational institutions run by the Indian Central Government. This book has four chapters. In chapter one the authors introduce the three types of super FRM models. Chapter two uses these three new super fuzzy models to study the role of media which feverishly argued against 27 percent reservation for OBCs in Central Government-run institutions in India. The experts we consulted were divided into 19 groups depending on their profession. These groups of experts gave their opinion and comments on the news-items that appeared about reservations in dailies and weekly magazines, and the gist of these lengthy discussions form the third chapter of this book. The fourth chapter gives the conclusions based on our study. Our study was conducted from April 2006 to March 2007, at which point of time the Supreme Court of India stayed the 27 percent reservation for OBCs in the IITs, IIMs and AIIMS. After the aforesaid injunction from the Supreme Court, the experts did not wish to give their opinion since the matter was sub-judice. The authors deeply acknowledge the service of each and every expert who contributed their opinion and thus made this book a possibility. We have analyzed the data using the opinion of the experts who formed a heterogeneous group consisting of administrators, lawyers, OBC/SC/ST students, upper caste students and Brahmin students, educationalists, university vice-chancellors, directors, professors, teachers, retired Judges, principals of colleges, parents, journalists, members of the public, politicians, doctors, engineers, NGOs and government staff. The authors deeply acknowledge the unflinching support of Kama and Meena. W.B.VASANTHA KANDASAMY FLORENTIN SMARANDACHE K.KANDASAMY 6 Chapter One INTRODUCTION TO NEW SUPER FUZZY MODELS In this chapter the authors introduce some new fuzzy models using supermatrices. These new fuzzy models are used in chapter two to analyze the role of media in the context of OBC (Other Backward Castes/Classes) reservation in the institutions run by the central government. In the first section we recall the definition of supermatrices and then we define the notion of fuzzy supermatrices. Section two defines the new notion of fuzzy super models and shows how they function. 1.1 Supermatrices and Fuzzy Supermatrices Here we just recall the notion of supermatrices and define the new notion of fuzzy supermatrices. DEFINITION 1.1.1: Let V = (V1 V2 …Vn) where each Vi is a row vector vii... v , 1≤ i≤∈ n , v i Q or R, then V is denoted by V = ()1t1 j 11 1 11 1 n n n i (V1 … Vn) = vv"""" v||| vv v v v v where v ∈ Q {()12 t12 12 t 1 2 t n} j or R, i=1, 2, …, n and i≤ j ≤ ti. If t1, t2, …, tn are distinct then we call V to be a mixed super row vector. If t1 = t2 = … = tn we then call V to be a super row vector. We just illustrate this by the following example. 7 Example 1.1.1: Let V= (1 2 3 0 | 5 7 –3 1 | 6 1 2 7 | 0 0 –1 4); V is called the super row vector and V = (V1 V2 V3 V4) where each Vi is a 1 × 4 row vector. DEFINITION 1.1.2: Let V = (V1 V2 …Vn) be a super row vector, if the entries of each Vi is from [0, 1], the unit interval; i=1, 2,…, n then we call V to be a simple fuzzy super row vector. Let V= (V1 V2 …Vn) be the mixed super row vector, where each Vi is a 1 × ti row vector, i =1, 2, …, n and ti ≠ tj; if i ≠ j for atleast one i and j: 1 ≤ i , j ≤ n ; and if the entries of each of the row vector Vi is from the unit interval [0, 1]; 1 ≤ i ≤ n ; then we call V to be the mixed simple fuzzy super row vector. We illustrate the above definition by the following examples. Example 1.1.2: Let V = (10.7 0.8 | 0.9 0.2 0.3 0.4 | 0.6 1 0.5 0.2 1 0.8 0). V is a mixed simple fuzzy super row vector, the entry of each Vi is from [0, 1] where V = (V1 V2 V3); i = 1, 2, 3. Example 1.1.3: Let A = (1 0 1 0 | 0 1 1 1 | 1 1 1 1 | 1 0 0 1 | 1 1 1 0) = (A1 A2 A3 A4 A5). A is a simple fuzzy super row vector for entries of each Ai is from the set {0, 1} ⊆ [0, 1]. Now we proceed on to define simple super column vector and mixed simple super column vector. DEFINITION 1.1.3: Let ⎡M1 ⎤ ⎢M ⎥ M = ⎢ 2 ⎥ ⎢ # ⎥ ⎢ ⎥ ⎣M s ⎦ where Mi is a ti × 1 column vector with entries from Q or R, 1 ≤ i ≤ s; then we call M to be a simple mixed super column vector if ti ≠ tj for at least one i ≠ j. If t1 = t2 = … = ts then we call M to be a simple super column vector. 8 We illustrate this by following examples. Example 1.1.4: Let ⎡⎤3 ⎢⎥7 ⎢⎥ ⎡M1 ⎤ M = ⎢⎥1 = ⎢ ⎥ . ⎢⎥ ⎣M2 ⎦ ⎢⎥5 ⎣⎦⎢⎥2 M is a simple mixed super column vector. Example 1.1.5: Consider ⎡ 3 ⎤ ⎢ ⎥ ⎢ 1 ⎥ ⎢ 1 ⎥ ⎢ ⎥ ⎢ 2 ⎥ ⎡⎤M1 ⎢ 0 ⎥ ⎢⎥ M ⎢ 5 ⎥ M = ⎢⎥2 = ⎢ ⎥ . ⎢⎥M3 ⎢ 7 ⎥ ⎢⎥ M ⎢ 2 ⎥ ⎣⎦4 ⎢ ⎥ ⎢ 1 ⎥ ⎢−3⎥ ⎢ ⎥ ⎢ 1 ⎥ ⎢ ⎥ ⎣ 2 ⎦ M is a simple super column vector. Now we define simple fuzzy super column vector and mixed simple fuzzy super column vector. Let ⎡M1 ⎤ ⎢M ⎥ M = ⎢ 2 ⎥ ⎢ ⎥ ⎢ ⎥ ⎣Ms ⎦ 9 be a mixed simple super column vector, if each of the entries are from the unit interval [0, 1] then we call M to be mixed simple fuzzy super column vector. ⎡M1 ⎤ ⎢M ⎥ M = ⎢ 2 ⎥ ⎢ ⎥ ⎢ ⎥ ⎣Mt ⎦ be a simple super column vector where each Mi is a r × 1 column vector i =1, 2, …, t and each entry of Mi is from the unit interval [0, 1] then we call M to be a fuzzy super column vector. Example 1.1.6: Let ⎡ 1 ⎤ ⎢ ⎥ ⎢ 0 ⎥ ⎢ 1 ⎥ ⎢ ⎥ ⎢ 1 ⎥ ⎢0.2⎥ ⎢ ⎥ ⎢0.7 ⎥ ⎢ 0 ⎥ ⎢ ⎥ M = ⎢ 1 ⎥ . ⎢ 1 ⎥ ⎢ ⎥ ⎢0.5⎥ ⎢0.2⎥ ⎢ ⎥ ⎢0.7 ⎥ ⎢0.5⎥ ⎢ ⎥ ⎢ 1 ⎥ ⎢ ⎥ ⎣0.9⎦ M is a fuzzy super column vector. Next we proceed on to define the notion of super row matrix and mixed super row matrix. DEFINITION 1.1.4: Let M = [M1 M2 … Mj], where Mt is a s × pt matrix with s rows and pt columns, t = 1, 2, …, j ; where 10 elements of each Mt is from Q or R; 1 ≤ t ≤ j.
Details
-
File Typepdf
-
Upload Time-
-
Content LanguagesEnglish
-
Upload UserAnonymous/Not logged-in
-
File Pages455 Page
-
File Size-