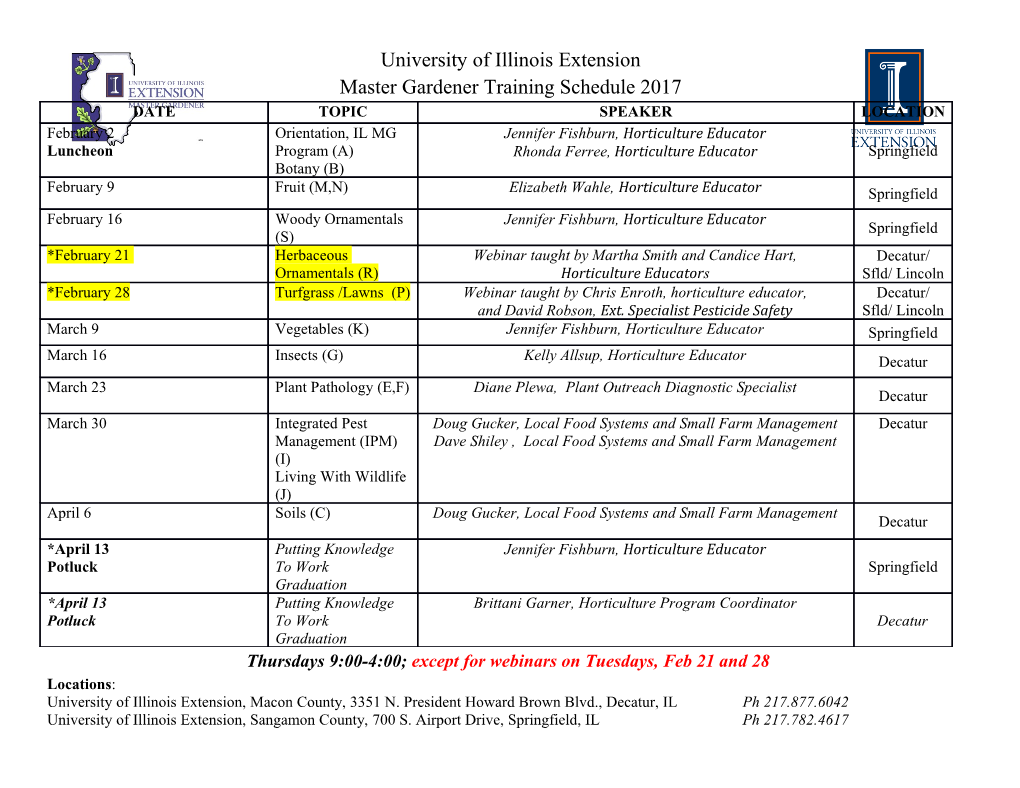
1 1 Systemic Risk Cycle: Evidence from ASEAN-5 2 3 Febrio Kacaribu1 4 Denny Irawan2 5 6 Abstract 7 8 We examine the cyclicality of systemic risk under the influence of business cycle dynamics by 9 using quarterly data of 73 listed banks in ASEAN-5 countries. The data spans from 2001Q1 to 10 2017Q2. We use Systemic Risk Index (SRISK) by Brownlees and Engle (2017), which represents 11 the capital shortfall of a bank in the time of a crisis, as the measure of systemic risk. We find 12 evidence for a countercyclical relationship between SRISK and the business cycle. There is a 13 strong evidence that the leverage ratio has a negative impact on the systemic risk dynamics. 14 15 Key words: Business Cycle, Banks Leverage, Macroprudential Policy 16 17 JEL classification numbers: E32, G21, E58, G28 1 Department of Economics, Universitas Indonesia 2 Arndt-Corden Department of Economics, The Australian National University 2 18 1. Introduction 19 The importance of systemic risk in the financial system has attracted a lot of attention since 20 the financial crisis of 2008. In the sphere of the macro-finance strand of literature, the crisis 21 has stimulated many studies to explain the new center of attention in the banking risk, the 22 systemic risk. By definition, systemic risk can be inferred as a potential of a financial institution 23 to be undercapitalized once the financial system is in the crisis (Engle et al, 2014). 24 Series of studies have been conducted to formulate measurement to quantify the 25 systemic risk. Up to recently, there are three most popular systemic measurements in the 26 literatures, they are: (i) Marginal Expected Shortfall (MES) by Acharya et al (2016), which is 27 based on Acharya et al (2012), (ii) Systemic Risk Index (SRISK) Measure by Brownlees and 28 Engle (2017), which is based on MES, and (iii) CoVaR or Conditional Value at Risk developed 29 by Adrian and Brunnermeier (2016). In addition to that, Lopez-Espinosa et al (2012) proposed 30 an extended version of CoVaR to be able to capture asymmetric response of the banks toward 31 shocks. Zedda and Cannas (2017) attempted to develop a systemic risk measurement with 32 Leave-One-Out (LOO) approach, which measure how system-wide risk would change if one 33 financial institution is excluded. Meanwhile, Varotto and Zhao (2018) developed a hybrid 34 systemic risk measurement called as “rSYR”. These studies, however, only focus on how to 35 define and measure systemic risk. Meanwhile, the world financial system is hardly to be free 36 from the imminent threat of the systemic risk. Thus, along the process of refining ways to 37 define and measure systemic risk, it is also important to put effort to explain – and then 38 control – the behavior of systemic risk. This condition motivates our study to explain the main 39 determinant of the systemic risk. In this study, we focus our analysis on the ASEAN-5 market. 40 We borrow a theoretical framework from Adrian and Shin (2013). The framework has 41 provided insight on two most important determinants of the probability of default, which are 42 leverage and the business cycle. Leverage is an aspect which can be controlled by banks. 43 Although leveraging is an inherent part of banking activities, many financial crises have shown 44 that excessive leverage by the banks could deteriorate the banks’ financial soundness and, 45 thus, put the financial system in danger. BASEL III provides clear guideline for bank supervisors 46 and regulators across the globe to ensure the banks’ leveraging activities to be at the utmost 47 prudence (BIS, 2017). The business cycle is impossible to be controlled by the banks. It is an 48 inherent macroeconomic feature of modern economy. This fact thus provides a strong ground 3 49 for this study to put attention toward how systemic risk could be influenced by the fluctuation 50 of the business cycle. 51 The business cycle is defined as the fluctuation of real aggregate economic activity 52 (Burns and Mitchell (1946); in Jacobs (1998)). At practical level, the business cycle is 53 represented in many literatures as the fluctuation of the gross domestic product (GDP). 54 However, as the GDP is measured quarterly, many studies also implement the Industrial 55 Production Index (IPI) as an alternative representation of the business cycle. Departing from 56 the definition of the business cycle and systemic risk, we define cyclicality of systemic risk as 57 the behavior of systemic risk along the business cycle fluctuation. This definition has specific 58 concern on how systemic risk may build up as compounded by the dynamics of the business 59 cycle. 60 This study attempts to examine the cyclicality of systemic risk toward the business and 61 financial cycle fluctuation. Several works have been done to address this problem. Liu (2016) 62 examines the dynamics of CoVaR of US Bank-Holding-Company (BHCs) using regime switching 63 approach. The result shows that CoVaR is pro-cyclical toward macroeconomic variables. In 64 line with that, Lenoci (2017) also finds that SRISK is pro-cyclical by examining US BHC data. 65 Tasca and Battiston (2011) also exhibit procyclicality phenomenon by simulating the 66 cyclicality of Value-at-Risk in hypothetical setting. 67 Several studies outline the role of deposit insurance in explaining the dynamics of 68 systemic risk. Calomiris and Jaremski (2016) point out the role of deposit insurance in 69 increasing banks’ risk-taking tendency and thus positively contribute toward increased 70 systemic risk. In support for this study, Acharya et al (2011) argued that deposit insurance 71 premium setting should be carefully considered so it could play role as a control tool to reduce 72 the banks’ moral hazard. Liu and Staum (2010) strongly argue that systemic risk components 73 of a bank should be considered when calculating the deposit insurance premium. An empirical 74 study by Kusairi et al (2018) exhibits that implementation deposit insurance scheme in ASEAN 75 countries induces banks to take more risk. 76 Our study offers three contributions. First, the base measurement employed in this 77 study is based on market information, i.e. the nature of volatility of the stock market through 78 the different economic cycle. Second, in the context of macroprudential management, this 79 study addresses one of the most important questions on how to make the financial system 80 more resilient. By examining the cyclicality of systemic risk, we can understand its dynamics 4 81 over-time and find the determinants of its cyclicality. Third, this study explores the role, if 82 any, of the deposit insurance scheme in managing the systemic risk. Most ASEAN countries in 83 our dataset have only implemented deposit insurance scheme after 2005, except for The 84 Philippines which have implemented such scheme since 1963. 85 This study is also one of the very few to address systemic risk issue in developing 86 countries, especially in South-East Asia. Most studies observe systemic risk development in 87 the developed countries, which are mostly characterized by relatively deep and mature 88 financial market. The South-East Asian financial market is, with some exception for Singapore, 89 characterized as developing and shallow financial market. 90 On the choice of the proxy for the systemic risk we choose SRISK, which is based on 91 LRMES. SRISK is defined as capital shortfall that a bank will be expected to lose on the 92 condition that the market is in crisis. 93 This paper is organized as follows. Section 1 provides introduction explaining the 94 contextual ground of the study. Section 2 provides brief of the literature ground related to 95 the study. Section 3 gives overview of ASEAN-5 banking and financial system. Section 4 96 explains our basic theoretical framework of how the business and financial cycles may 97 influence the systemic risk in the banks. Section 5 explains our dataset and empirical 98 estimation setting. Section 6 articulates the results of our study and discussion of some 99 impact toward the literature development and the practice the macroprudential 100 management. Section 7 provides conclusion and recommendation. 101 102 2. Literature Review 103 The systemic risk indexes that are involved here, LRMES and SRISK, are based on market 104 information, especially the fluctuation of the company price in term of its market value. The 105 spirit comes from the fact that recent financial crises are mostly caused by the sudden fall of 106 asset price in such a short period. The deterioration of asset price, as standard business cycle 107 theory mentions, is a strong symptom that the economy is in the downturn phase. 108 The work of Schwert (1989) is one of initial attempts to relate macroeconomic 109 fundamentals with asset price. In his work, he models the stock price as the discounted 110 present value of expected future cash flow to stockholders. On the aggregate level, the value 111 of corporate equity depends on the health of the economy. Thus, the uncertainty of future 112 macroeconomic conditions would cause a proportional change in stock return volatility. This 5 113 framework is still in place until recently, as followed by Engle (2014), Subrahmanyam and 114 Titman (2013), and Hamilton and Lin (1996), David (1997) and Veronesi (1999). 115 In the same spirit, Bansal and Yaron (2004) develop Dynamic Capital Asset Pricing 116 Model (DCAPM) to relate macroeconomic condition with asset price volatility, based on the 117 dynamic consumption preference model developed by Epstein and Zin (1989). The model 118 incorporates changes in the conditional volatility of future growth rates to allow time-varying 119 risk premia.
Details
-
File Typepdf
-
Upload Time-
-
Content LanguagesEnglish
-
Upload UserAnonymous/Not logged-in
-
File Pages38 Page
-
File Size-