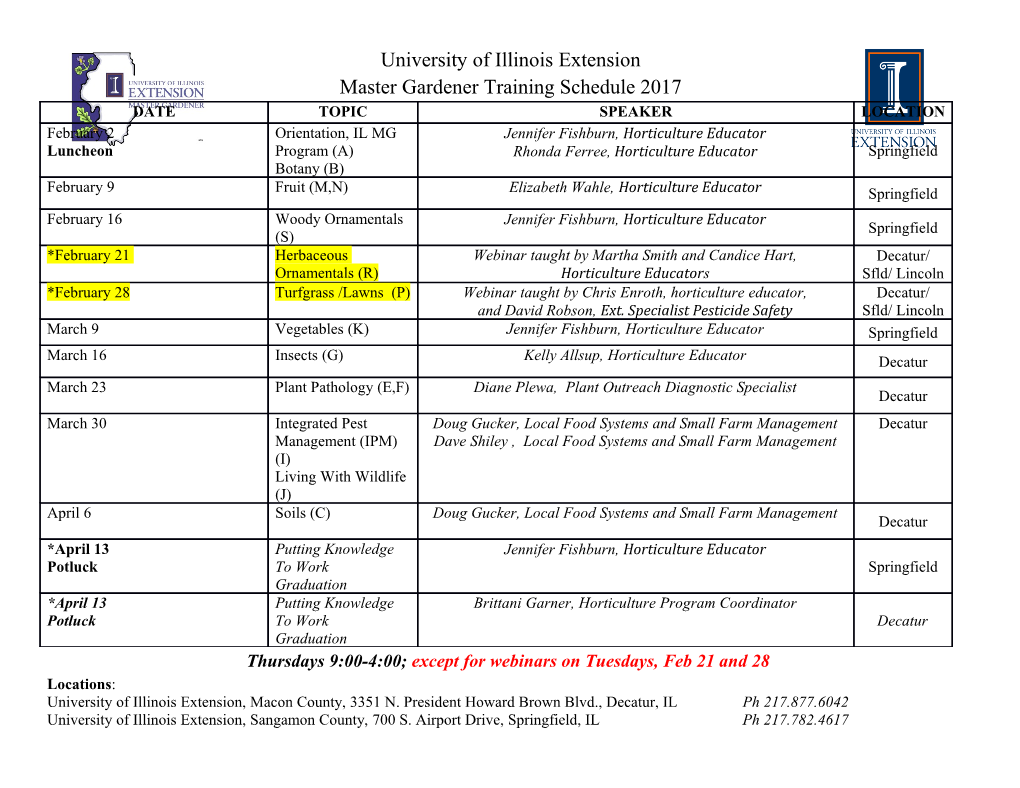
Copyright by Yuqing Lin 2020 The Dissertation Committee for Yuqing Lin certifies that this is the approved version of the following dissertation: Non-unique topological sofic entropy and a von Neumann algebra multiplicative ergodic theorem Committee: Lewis Bowen, Supervisor David Kerr Joseph Neeman Lorenzo Sadun Non-unique topological sofic entropy and a von Neumann algebra multiplicative ergodic theorem by Yuqing Lin Dissertation Presented to the Faculty of the Graduate School of The University of Texas at Austin in Partial Fulfillment of the Requirements for the Degree of Doctor of Philosophy The University of Texas at Austin May 2020 Acknowledgments I could not have come this far without the guidance and support of many people. I would like to thank my advisor Lewis Bowen for all the time, effort, and support he put into mentoring me, working with me, encouraging me, and being patient with me throughout my studies. I would like to thank the UT department of mathematics along with all the pro- fessors, fellow graduate students, and staff for instruction, encouragement, conversations, community, and administrative help. I would like to thank the wider mathematical community for allowing me to participate in conferences and mathematical activities, and especially those who also encouraged and welcomed me. I would like to thank my family, personal friends, mentors, and church community for their love, encouragement, guidance, and community. I would also like to thank everyone who has ever made me laugh or smile, even for a brief moment. Yuqing Lin The University of Texas at Austin May 2020 iv Abstract Non-unique topological sofic entropy and a von Neumann algebra multiplicative ergodic theorem Yuqing Lin, Ph.D. The University of Texas at Austin, 2020 Supervisor: Lewis Bowen A sofic approximation to a countable group is a sequence of partial actions on finite sets that asymptotically approximates the action of the group on itself by left-translations. A group is sofic if it admits a sofic approximation. Sofic entropy theory is a generalization of classical entropy theory in dynamics to actions by sofic groups. However, the sofic entropy of an action may depend on a choice of sofic approximation. All previously known examples showing this dependence rely on degenerate behavior. In joint work with D. Airey and L. Bowen an explicit example is exhibited of a mixing subshift of finite type with two different positive sofic entropies. The example is inspired by statistical physics literature on 2-colorings of random hyper-graphs. Also, in joint work with L. Bowen and B. Hayes, the classical Multiplicative Ergodic Theorem (MET) of Oseledets is generalized to cocycles taking values in a type II von Neumann algebra. This appears to be the first MET involving operators with continuous spectrum. v Table of Contents Chapter 1 Introduction 1 1.1 General introduction to \A topological dynamical system with two different positive sofic entropies" . 1 1.2 General introduction to \A The multiplicative ergodic theorem for von Neu- mann algebra valued cocycles" . 10 Chapter 2 A topological dynamical system with two different positive sofic entropies 16 2.1 Introduction . 16 2.1.1 Random sofic approximations . 18 2.1.2 Proper colorings of random hyper-graphs from a statistical physics viewpoint . 18 2.1.3 The action . 22 2.1.4 Sofic entropy of the shift action on proper colorings . 23 2.1.5 Random hyper-graph models . 24 2.1.6 The strategy and a key lemma . 25 2.2 Topological sofic entropy . 26 2.3 Reduction to the key lemma . 28 2.4 The first moment . 33 2.4.1 Almost proper 2-colorings . 34 2.4.2 Equitable colorings . 38 2.5 The second moment . 41 2.5.1 Analysis of 0 and the proof of Lemma 2.1.2 inequality (2.2) . 51 vi 2.5.2 Reducing Lemma 2.1.2 inequality (2.3) to estimating the local cluster 59 2.6 The local cluster . 63 2.7 A Markov process on the Cayley hyper-tree . 66 2.7.1 Local convergence . 67 2.7.2 The density of the rigid set . 70 2.8 Rigid vertices . 76 2.9 Appendix A: Topological sofic entropy notions . 83 2.10 Appendix B: Concentration for the planted model . 86 2.10.1 General considerations . 87 2.10.2 Specific considerations . 92 Chapter 3 A multiplicative ergodic theorem for von Neumann algebra valued cocycles 100 3.1 Introduction . 100 3.1.1 The finite dimensional MET . 100 3.1.2 Previous literature . 101 3.1.3 von Neumann algebras . 102 3.1.4 Example: the abelian case . 102 3.1.5 Main results . 103 3.1.6 The abelian case . 108 3.1.7 Powers of a single operator . 108 3.1.8 Proof overview . 109 3.2 The abelian case . 111 3.2.1 Theorem 3.1.1 from the pointwise ergodic . 111 3.2.2 Examples with continuous spectrum . 112 3.2.3 Almost uniform convergence and growth rates . 112 3.2.4 A counterexample . 114 3.3 Preliminaries . 116 3.3.1 Spectral measures . 116 3.3.2 Polar decomposition . 117 3.3.3 The regular representation . 118 vii 3.3.4 The algebra of affiliated operators . 118 3.4 The log-square integrable general linear group . 120 3.5 The geometry of positive definite operators . 124 3.5.1 The space P1(M; τ) of bounded positive operators . 124 3.5.2 The measure topology . 127 3.5.3 The space P(M; τ) of positive log-square integrable operators . 132 3.5.4 Continuity of the exponential map . 138 3.5.5 Semi-finite case . 142 3.6 Proofs of the main results . 150 3.6.1 The limit operator . 150 3.6.2 Determinants . 157 3.6.3 Growth rates . 158 3.6.4 Essentially dense subspaces . 162 3.6.5 Invariance . 165 Bibliography 169 viii Chapter 1 Introduction 1.1 General introduction to \A topological dynamical system with two different positive sofic entropies" Shannon entropy Suppose we have a random variable X taking values in a finite set A, and suppose that we seek a function giving a quantitative representation of how much information one would obtain, or how \surprised" one would be, to learn of a particular outcome in A. Suppose we want this function to depend only on the probability of an outcome. Naturally, the rarer the outcome the more surprising its occurrence would be. On the other hand if an outcome has probability 1, then its occurrence holds no information or surprise. Another reasonable assumption is that one learns the same amount of information from the simultaneous oc- currence of two independent events as from the separate occurrence of these events. This leads us to a function I : (0; 1] ! [0; 1) such that I is monotone decreasing, I(1) = 0, and I(xy) = I(x) + I(y). A natural candidate is then I(x) = − log(x). The above is a sim- plified account of the approach that Claude Shannon took when he introduced information entropy in [Sha48]. The motivation for Shannon's work was in data communication - he showed that entropy is a theoretical limit to how efficiently data can be coded without losing any information. This Shannon entropy H(X) is defined to be the expected value of the 1 information one learns from X, i.e. - X H(X) = − pa log pa a2A where pa is the probability that X has outcome a 2 A. By continuity we let 0 log 0 = 0. It can be deduced that H is maximized when all outcomes are equally likely. The history of entropy can be traced back to thermodynamics in 1854, when Rudolf Clausius ([Cla56]) gave the first mathematical formulation of entropy. In the 1870s Boltzman and Gibbs introduced a statistical mechanical view of entropy. We give a brief illustration found in Petersen ([Pet89]) of the connection to Shannon entropy. Suppose there are n molecules and states s1; :::; sk, and we have a system where pi proportion of the particles are in state i. Then the number of ways for the particles to be in such a distribution is n . p1n;p2n;:::;pkn nH(p) P Using Stirling's formula, this number is approximately e , where H(p) = − pi log pi as seen earlier. Thus higher entropy is related to a larger number of possibilities, which can be interpreted as higher uncertainty or randomness. This statistical mechanical view of counting the exponential growth rate of \microstates" turns out to be the approach taken in sofic entropy. Entropy rate Suppose now that we have a stationary stochastic process X = (X1;X2; :::) and we want to quantify the amount of information per unit of time that we learn from this process. 1 Let h(X) = limn!1 n H(X1; :::; Xn). This is the notion of entropy rate. A stochastic process is stationary if the law of the shifted process (X2;X3; :::) is the same as the law of X. Assuming stationarity, all random variables in the sequence have the same law, and therefore the same Shannon entropy. The entropy rate of the process is in general less than or equal to the Shannon entropy of any single random variable Xi since knowledge of the past values allows one to make better predictions of the future. For example, Shannon ([Sha51]) tried to estimate the entropy of the English language. Using standard tables the Shannon entropy of a single letter, chosen uniformly at random from amongst pieces of typical English prose, assuming a twenty-seven letter alphabet (in- cluding spaces), is about 4.03 bits (i.e. if log base 2 is used in the formula for entropy).
Details
-
File Typepdf
-
Upload Time-
-
Content LanguagesEnglish
-
Upload UserAnonymous/Not logged-in
-
File Pages183 Page
-
File Size-