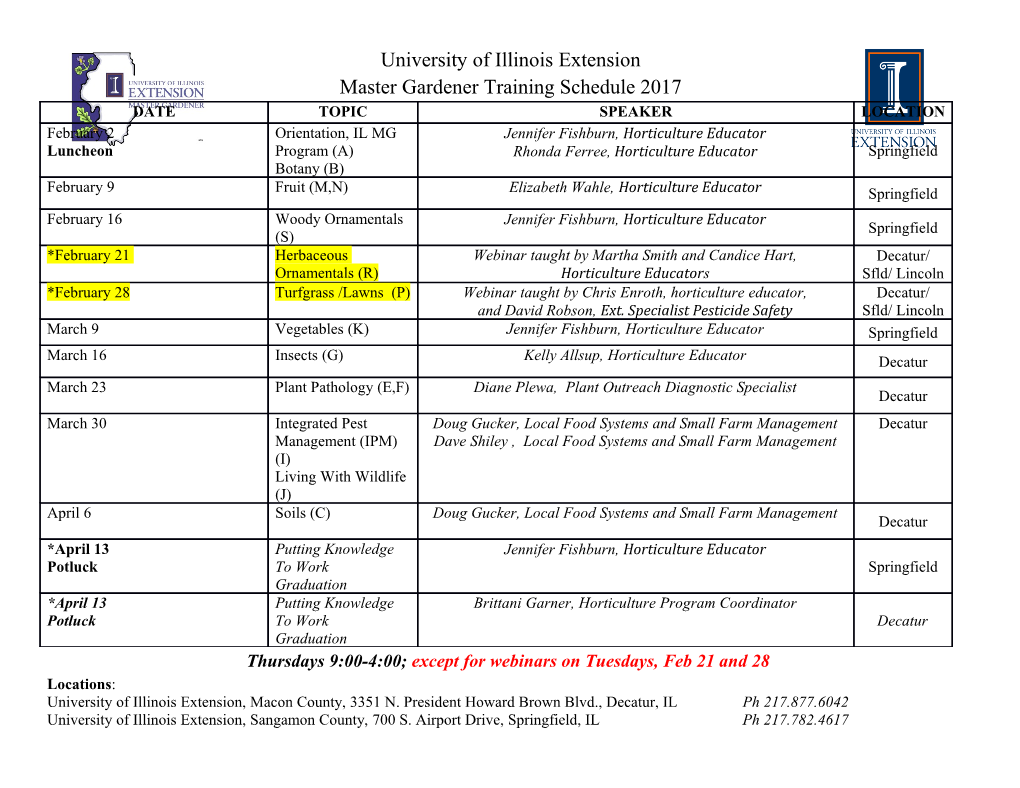
Journal of Risk and Financial Management Article The Burr X Pareto Distribution: Properties, Applications and VaR Estimation Mustafa Ç. Korkmaz 1,* ID , Emrah Altun 2, Haitham M. Yousof 3, Ahmed Z. Afify 3 ID and Saralees Nadarajah 4 1 Department of Measurement and Evaluation, Artvin Çoruh University, Artvin 08000, Turkey 2 Department of Statistics, Hacettepe University, Ankara 06800, Turkey; [email protected] 3 Department of Statistics, Mathematics and Insurance, Benha University, Benha 13511, Egypt; [email protected] (H.M.Y.); [email protected] (A.Z.A.) 4 School of Mathematics, University of Manchester, Manchester M13 9PL, UK; [email protected] * Correspondence: [email protected] Received: 31 October 2017; Accepted: 18 December 2017; Published: 21 December 2017 Abstract: In this paper, a new three-parameter Pareto distribution is introduced and studied. We discuss various mathematical and statistical properties of the new model. Some estimation methods of the model parameters are performed. Moreover, the peaks-over-threshold method is used to estimate Value-at-Risk (VaR) by means of the proposed distribution. We compare the distribution with a few other models to show its versatility in modelling data with heavy tails. VaR estimation with the Burr X Pareto distribution is presented using time series data, and the new model could be considered as an alternative VaR model against the generalized Pareto model for financial institutions. Keywords: Burr X distribution; Pareto distribution; maximum likelihood estimation; heavy tail distribution; value-at-risk 1. Introduction The Pareto (P) distribution is very versatile, and a variety of uncertainties can be usefully modelled by it. It has several applications in actuarial science, economics, finance, life testing, survival analysis and telecommunications because of its heavy tail properties. The probability density function (pdf) and cumulative distribution function (cdf) of the P distribution are given (for x > b) by: a x −a x −a g(x; a, b) = and G(x; a, b) = 1 − , x b b where b > 0 is a scale parameter and a > 0 is a shape parameter. This distribution is a special form of the Pearson Type VI distribution. Since the P distribution has a reversed-J pdf shape and a decreasing hazard rate function (hrf), it may sometimes be insufficient to model data. Generally, practical problems require a wider range of possibilities for the medium risk, for example when the lifetime data present a bathtub-shaped hrf, such as human mortality and machine life cycles. For this reason, researchers developed various extensions and modified forms of the P distribution to obtain a more flexible model with different numbers of parameters. Some of them can be cited as follows: Exponentiated P (EP) (Stoppa 1990; Gupta et al. 1998), Beta P (BP) (Akinsete et al. 2008), Kumaraswamy P (KwP) (Bourguignon et al. 2013), Kumaraswamy generalized P (Nadarajah and Eljabri 2013), P ArcTan (PAT) (Gómez-Déniz and Calderín-Ojeda 2015), exponentiated Weibull P (Afify et al. 2016) and Weibull P J. Risk Financial Manag. 2018, 11, 1; doi:10.3390/jrfm11010001 www.mdpi.com/journal/jrfm J. Risk Financial Manag. 2018, 11, 1 2 of 16 (WP) distributions (Tahir et al. 2016). On the other hand, Yousof et al.(2016) defined the cdf of the Burr X-G(BX-G) family (for x 2 <) by: ( )!d G(x; x) 2 F(x; d, x) = 1 − exp − , (1) G(x; x) where d > 0 is the shape parameter and x = xk = ( x1, x2, ... ) is a parameter vector. The BX-G density function becomes: ( ) ( )!d−1 2dg(x; x)G(x; x) G(x; x) 2 G(x; x) 2 f (x; d, x) = exp − 1 − exp − . (2) G(x; x)3 G(x; x) G(x; x) This generator can supply the flexibility of pdf and hrf to any baseline distribution model (Yousof et al. 2016). In this paper, we introduce a new extended P distribution, called the Burr X Pareto (BXP) model, based on the BX-G family. With this idea, we construct the new BXP distribution as more flexible than the P distribution and provide a comprehensive description of some of its mathematical properties. We prove empirically that the BXP model provides better fits than some extensions and generalizations of the P, some of which have one extra model parameter, and the others have the same number of parameters, by means of two applications to real data. We hope that the new distribution will attract wider applications in reliability, engineering and other areas of research. The rest of the paper is organized as follows. In Section2, we define the BXP model. In Section3, we provide a useful mixture representation for its pdf. In Section4, we derive some of its general mathematical properties. Some estimation methods of the model parameters are performed in Section5. In Section6, simulation results to assess the performance of the proposed maximum likelihood estimation procedure are discussed. In Section7, we provide two applications to real data to illustrate the importance and flexibility of the new family. Value-at-Risk estimation with the BXP distribution is presented in Section8. Finally, some concluding remarks are presented in Section9. 2. The New Model In this section, we define the BXP model and provide some plots for its pdf and hrf. The BXP cdf is given by: ( )!d x a 2 F(x; d, a, b)= 1 − exp − − 1 , x > b > 0, a, d > 0. (3) b The pdf corresponding to (3) is given by: 2a −a a 2 a x x h x i f (x; d, a, b) = 2d x b 1 − b exp − b − 1 (4) a 2d−1 h x i × 1 − exp − b − 1 . Lemma1 provides random number generations from the BXP and some relations and of the BXP distribution with the well-known Burr X and uniform distributions. Lemma 1. (a) If a random variable Y follows the Burr X distribution with shape parameter d and scale parameter one, then the random variable X = b(1 + Y)(1/a) follows the BXP(d, a, b) distribution. (b) If a random variable Y follows the uniform distribution on [0,1], then the random variable: q 1/a X = b 1 + − log1 − Y1/d follows the BXP(d, a, b) distribution. J. Risk Financial Manag. 2018, 11, 1 3 of 16 Proof. The proofs of (a) and (b) are obtained by the transformation method. The hrf, reversed hazard rate function and cumulative hazard rate function of X are given, respectively, by: 2a −a a 2 a 2d−1 a x x h x i h x i 2d x b 1 − b exp − b − 1 1 − exp − b − 1 h(x; d, a, b) = , a 2d h x i 1 − 1 − exp − b − 1 2a −a a 2 a x x h x i 2d x b 1 − b exp − b − 1 r(x; d, a, b) = a 2 h x i 1 − exp − b − 1 and: 2 ( )!d3 x a 2 H(x; d, a, b) = −4log 1 − exp − − 1 5. b In Figure1, we sketched the possible pdf and hrf shapes of the BXP distribution for some selected parameter values. Figure1 shows that the BXP distribution has various pdf and hrf shapes. δ=2,α=2.5,β=0.5 δ=2,α=1.9,β=0.5 δ=2,α=1.7,β=0.5 δ=2,α=1.5,β=0.5 δ=2,α=1.3,β=0.5 δ=0.5,α=2,β=0.5 δ=0.4,α=2,β=0.5 δ=0.3,α=2,β=0.5 δ=0.9,α=0.9,β=0.5 δ=0.2,α=2,β=0.5 δ=0.9,α=0.8,β=0.5 δ=0.1,α=2,β=0.5 δ=0.9,α=0.7,β=0.5 δ=0.9,α=0.6,β=0.5 f(x) f(x) f(x) δ=0.9,α=0.5,β=0.5 0 2 4 6 8 10 0 2 4 6 8 0.0 0.5 1.0 1.5 0.5 0.6 0.7 0.8 0.9 1.0 0.6 0.8 1.0 1.2 1.4 1 2 3 4 5 x x x δ=0.5,α=0.1,β=0.5 δ=3,α=2.5,β=0.5 δ=0.4,α=0.1,β=0.5 δ=4,α=2.5,β=0.5 δ=0.5,α=0.1,β=0.5 δ=5,α=2.5,β=0.5 δ=0.2,α=0.1,β=0.5 δ=6,α=2.5,β=0.5 δ=0.1,α=0.1,β=0.5 δ=7α=2.5,β=0.5 h(x) h(x) h(x) δ=1.3,α=0.15,β=0.5 δ=1.4,α=0.15,β=0.5 δ=1.5,α=0.15,β=0.5 δ=1.6,α=0.15,β=0.5 δ=1.7,α=0.15,β=0.5 0 2 4 6 8 0.0 0.1 0.2 0.3 0.4 0.000 0.005 0.010 0.015 0.020 0.025 1 2 3 4 5 0 5 10 15 0.50 0.55 0.60 0.65 0.70 x x x Figure 1. Plots of the Burr XPareto (BXP) pdf (top) and plots of the BXP hazard rate function (hrf) (bottom). J. Risk Financial Manag. 2018, 11, 1 4 of 16 3. Expansions of pdf and cdf In this section, we provide a very useful linear representation for the BXP density function.
Details
-
File Typepdf
-
Upload Time-
-
Content LanguagesEnglish
-
Upload UserAnonymous/Not logged-in
-
File Pages16 Page
-
File Size-