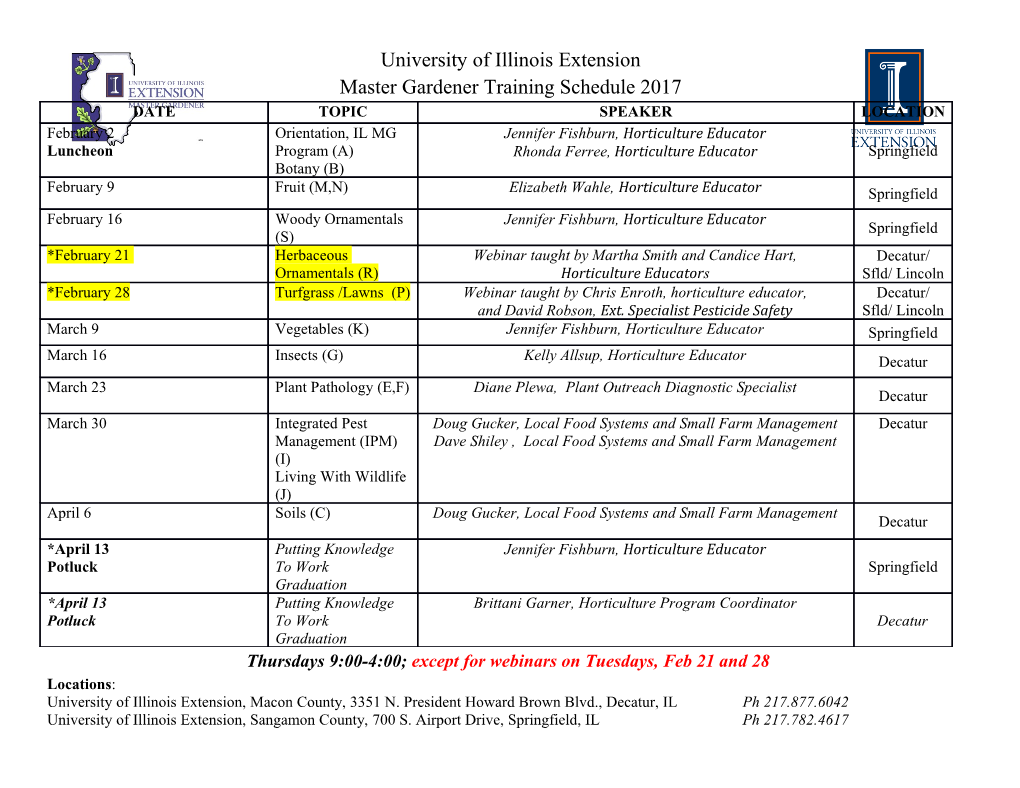
A Long Short-Term Memory Recurrent Neural Network Framework for Network Traffic Matrix Prediction Abdelhadi Azzouni and Guy Pujolle LIP6 / UPMC; Paris, France fabdelhadi.azzouni,[email protected] Abstract—Network Traffic Matrix (TM) prediction is defined clusters in near real-time. Another example is, upon congestion as the problem of estimating future network traffic from the occurrence in the network, traditional routing protocols cannot previous and achieved network traffic data. It is widely used in react immediately to adjust traffic distribution, resulting in network planning, resource management and network security. Long Short-Term Memory (LSTM) is a specific recurrent neural high delay, packet loss and jitter. Thanks to the early warning, network (RNN) architecture that is well-suited to learn from a proactive prediction-based approach will be faster, in terms experience to classify, process and predict time series with time of congestion identification and elimination, than reactive lags of unknown size. LSTMs have been shown to model temporal methods which detect congestion through measurements, only sequences and their long-range dependencies more accurately after it has significantly influenced the network operation. than conventional RNNs. In this paper, we propose a LSTM RNN framework for predicting Traffic Matrix (TM) in large networks. Several methods have been proposed in the literature for By validating our framework on real-world data from GEANT´ network traffic forecasting. These can be classified into two network, we show that our LSTM models converge quickly and categories: linear prediction and nonlinear prediction. The give state of the art TM prediction performance for relatively most widely used traditional linear prediction methods are: a) small sized models. the ARMA/ARIMA model [3], [6], [7] and b) the HoltWinters keywords - Traffic Matrix, Prediction, Neural Networks, algorithm [3]. The most common nonlinear forecasting meth- Long Short-Term Mermory ods involve neural networks (NN) [3], [8], [9]. The experi- mental results from [13] show that nonlinear traffic prediction I. INTRODUCTION based on NNs outperforms linear forecasting models (e.g. Most of the decisions that network operators make depend ARMA, ARAR, HW) which cannot meet the accuracy re- on how the traffic flows in their network. However, although it quirements. Choosing a specific forecasting technique is based is very important to accurately estimate traffic parameters, cur- on a compromise between the complexity of the solution, rent routers and network devices do not provide the possibility characteristics of the data and the desired prediction accuracy. for real-time monitoring, hence network operators cannot react [13] suggests if we take into account both precision and effectively to the traffic changes. To cope with this problem, complexity, the best results are obtained by the Feed Forward prediction techniques have been applied to predict network NN predictor with multiresolution learning approach. parameters and therefore be able to react to network changes Unlike feed forward neural networks (FFNN), Recurrent in near real-time. Neural Network (RNNs) have cyclic connections over time. The predictability of network traffic parameters is mainly The activations from each time step are stored in the internal determined by their statistical characteristics and the fact state of the network to provide a temporal memory. This that they present a strong correlation between chronolog- capability makes RNNs better suited for sequence modeling arXiv:1705.05690v3 [cs.NI] 8 Jun 2017 ically ordered values. Network traffic is characterized by: tasks such as time series prediction and sequence labeling self-similarity, multiscalarity, long-range dependence and a tasks. highly nonlinear nature (insufficiently modeled by Poisson and Long Short-Term Memory (LSTM) is a RNN architecture Gaussian models) [2]. that was designed by Hochreiter and Schmidhuber [15] to A network TM presents the traffic volume between all pairs address the vanishing and exploding gradient problems of of origin and destination (OD) nodes of the network at a conventional RNNs. RNNs and LSTMs have been successfully certain time t. The nodes in a traffic matrix can be Points- used for handwriting recognition [1], language modeling, of-Presence (PoPs), routers or links. phonetic labeling of acoustic frames [10]. Having an accurate and timely network TM is essential In this paper, we present a LSTM based RNN framework for most network operation/management tasks such as traffic which makes more effective use of model parameters to train accounting, short-time traffic scheduling or re-routing, network prediction models for large scale TM prediction. We train and design, long-term capacity planning, and network anomaly compare our LSTM models at various numbers of parameters detection. For example, to detect DDoS attacks in their early and configurations. We show that LSTM models converge stage, it is necessary to be able to detect high-volume traffic quickly and give state of the art TM prediction performance for relatively small sized models. Note that we do not address The autoregressive model fitted to the mean-corrected series the problem of TM estimation in this paper and we suppose Xt = St − S, t = k + 1; n, where S represents the sample that historical TM data is already accurately obtained. mean for Sk+1; :::; Sn , is given by φ(B)Xt = Zt , where l1 l2 l3 The remainder of this paper is organized as follows: Section φ(B) = 1 − φ1B − φl1 B − φl2 B − φl3 B ; fZtg ≈ 2 2 II summarizes time-series prediction techniques. LSTM RNN WN(0; σ ), while the coefficients φj and the variance σ are architecture and equations are detailed in section III. We detail calculated using the YuleWalker equations described in [20]. the process of feeding our LSTM architecture and predicting We obtain the relationship: TM in section IV. The prediction evaluation and results are presented in section V. Related work is presented in section ξ(B)Yt = φ(1)S + Zt (4) VI and the paper is concluded by section VII. k+l3 where ξ(B)Yt = (B)'(B) = 1 + ξ1B + ::: + ξk+l3 B II. TIME SERIES PREDICTION From the following recursion relation we can determine the linear predictors In this section, we give a brief summary of various linear k+l predictors based on traditional statistical techniques, such as X3 ARMA (Autoregressive Moving Average), ARIMA (Autore- PnYn+h = − ξPnYn+h−j + φ(1)S h ≥ 1 (5) gressive Integrated Moving Average), ARAR (Autoregressive j=1 Autoregressive) and HW (HoltWinters) algorithm. And non- with the initial condition PnYn+h = Yn+h for h ≤ 0. linear time series prediction with neural networks. 1) Linear Prediction: d) HoltWinters algorithm: The HoltWinters forecasting a) ARMA model: The time series fXtg is called an algorithm is an exponential smoothing method that uses recur- ARMA(p, q) process if fXtg is stationary (i.e. its statistical sions to predict the future value of series containing a trend. properties do not change over time) and If the time series has a trend, then the forecast function is: Xt −φ1Xt−1 −:::−φpXt−p = Zt +θ1Zt−1 +:::+θqZt−q (1) Ybn+h = PnYn+h = an + bnh (6) 2 b where fZtg ≈ WN(0; σ ) is white noise with zero mean 2 and variance σ and the polynomials φ(z) = 1 − φ1z − ::: − where ban and bn are the estimates of the level of the trend p q φpz and θ(z) = 1+θ1z +:::+θqz have no common factors. function and the slope respectively. These are calculated using The identification of a zero-mean ARMA model which the following recursive equations: describes a specific dataset involves the following steps [20]: ( a) order selection (p, q); b) estimation of the mean value of the an+1 = αYn+1 + (1 − α)(an + bn) b b (7) series in order to subtract it from the data; c) determination of bn+1 = β(ban+1 − ban) + (1 − β)bn the coefficients fφi; i = 1; pg and fθi; i = 1; qg; d) estimation 2 of the noise variance σ . Predictions can be made recursively Where Ybn+1 = PnYn+1 = ban + bn represents the one- using: step forecast. The initial conditions are: ba2 = Y2 and b2 = 8Pn θnj(Xn+1−j − Xbn+1−j) if1 ≤ n ≤ m) Y2 − Y1. The smoothing parameters α and β can be chosen <> j=1 Pq either randomly (between 0 and 1), or by minimizing the sum Xbn+1 = j=1 θnj(Xn+1−j − Xbn+1−j) of squared one-step errors Pn (Y − P Y )2 [20]. :>+φ X + :: + φ X ifn ≥ m i=3 i i−1 i 1 n p n+1−p 2) Neural Networks for Time Series Prediction: Neural where m = max(p; q) and θ is determined using the nj Networks (NN) are widely used for modeling and predicting innovations algorithm. network traffic because they can learn complex non-linear b) ARIMA model: A ARIMA(p, q, d) process is de- patterns thanks to their strong self-learning and self- adaptive scribed by: capabilities. NNs are able to estimate almost any linear or non-linear function in an efficient and stable manner, when φ(B)(1 − B)dX = θ(B)Z (2) t t the underlying data relationships are unknown. The NN model where φ and θ are polynomials of degree p and q respec- is a nonlinear, adaptive modeling approach which, unlike the tively, (1−B) represents the differencing operator, d indicates techniques presented above, relies on the observed data rather the level of differencing and B is the backward-shift operator, than on an analytical model. The architecture and the param- j i.e. B Xt = Xt−j eters of the NN are determined solely by the dataset. NNs are c) ARAR algorithm: The ARAR algorithm applies characterized by their generalization ability, robustness, fault memory-shortening transformations, followed by modeling the tolerance, adaptability, parallel processing ability, etc [14].
Details
-
File Typepdf
-
Upload Time-
-
Content LanguagesEnglish
-
Upload UserAnonymous/Not logged-in
-
File Pages6 Page
-
File Size-