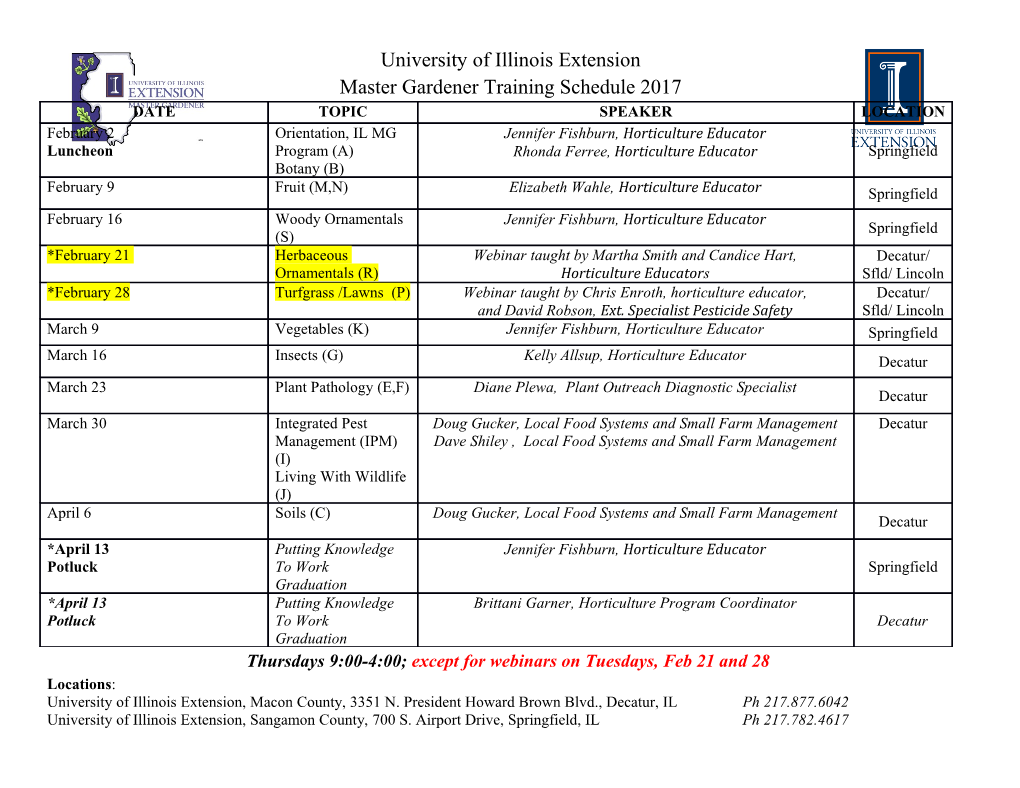
Computer Vision Stochastic Grammars for Scene Parsing Song-Chun Zhu Ying Nian Wu August 18, 2020 Contents 0.1 This Book in the Series . ix Part I Stochastic Grammars in Vision 1 Introduction 3 1.1 Vision as Joint Parsing of Objects, Scenes, and Events . .3 1.2 Unified Representation for Models, Algorithms, and Attributes . .6 1.2.1 Three Families of Probabilistic Models . .6 1.2.2 Dynamics of Three Inferential Channels . .7 1.2.3 Three Attributes associated with each Node . .7 1.3 Missing Themes in Popular Data-Driven Approaches . .8 1.3.1 Top-Down Inference in Space and Time . .8 1.3.2 Vision is to be a Continuous Computational Process . .9 1.3.3 Resolving Ambiguities and Preserving Distinct Solutions . 11 1.3.4 Vision is Driven by a Large Number of Tasks . 12 1.4 Scope of Book: Compositional Patterns in the Middle Entropy Regime . 13 1.4.1 Information Scaling and Three Entropy Regimes . 14 1.4.2 Organization of Book . 14 2 Overview of Stochastic Grammar 17 2.1 Grammar as a Universal Representation of Intelligence . 17 2.2 An Empiricist’s View of Grammars . 18 2.3 The Formalism of Grammars . 20 2.4 The Mathematical Structure of Grammars . 21 2.5 Stochastic Grammar . 23 2.6 Ambiguity and Overlapping Reusable Parts . 24 2.7 Stochastic Grammar with Context . 26 3 Spatial And-Or Graph 29 3.1 Three New Issues in Image Grammars in Contrast to Language . 29 3.2 Visual Vocabulary . 31 3.2.1 The Hierarchical Visual Vocabulary – the "Lego Land" . 31 3.2.2 Image Primitives . 32 3.2.3 Basic Geometric Groupings . 34 3.2.4 Parts and Objects . 35 3.3 Relations and configurations . 36 i 3.3.1 Relations . 37 3.3.2 Configurations . 39 3.3.3 The Reconfigurable Graphs . 40 3.4 Parse Graph for Objects and Scenes . 42 3.5 Knowledge Representation with And-Or Graph . 44 3.5.1 And-Or graph . 44 3.5.2 Stochastic Models on the And-Or graph . 47 3.6 Examples in Related Work . 49 3.6.1 Probabilistic Geometric Grammars . 49 3.6.2 Mixture of Deformable Part-based Models and Object Detection Grammars . 49 3.6.3 Probabilistic Program Induction . 50 3.6.4 Recursive Cortical Networks . 51 4 Learning the And-Or Graph 55 4.1 Overview of the Learning Problem . 55 4.1.1 Learning Parameters in Stochastic Context-Free Grammar . 57 4.1.2 Probability Model for AOG . 58 4.2 Learning Parameters in AOG . 59 4.2.1 Maximum Likelihood Learning of Θ ......................... 60 4.2.2 Learning and Pursuing the Relation Set . 61 4.2.3 Examples of Sampling and Synthesis from AOG . 61 4.2.4 Summary of the Parameter Learning . 62 4.3 Structure Learning:Block Pursuit and Graph Compression . 63 4.3.1 Hybrid Image Templates (HIT) as Terminal Nodes . 69 4.3.2 AOT: Reconfigurable Object Templates . 73 4.3.3 Learning AOT from Images . 75 4.3.4 Inference on AOTs . 80 4.3.5 Example: The Synthesized 1D Text AOT Learning . 83 4.4 Structure Learning: Unsupervised Structure Learning . 86 4.4.1 Algorithm Framework . 86 4.4.2 And-Or Fragments . 87 4.5 Structure Learning:Pruning from Full Graph . 89 4.5.1 General Framework . 91 4.5.2 Example: Learning Image Tangram Model . 91 5 Parsing Algorithms for Inference in And-Or Graphs 101 5.1 Classic Search and Parsing Algorithms . 101 5.1.1 Heuristic Search in And-Graph, Or-Graph and And-Or-Graph . 101 5.1.2 Bottom-Up Chart Parsing . 120 5.1.3 Top-Down Earley Parser and Generalization . 128 5.1.4 Inside-Outside Algorithm for Parsing and Learning . 131 5.1.5 Figure of Merit Parsing . 135 5.2 Scheduling Top-down and Bottom-up Processes for Object Parsing . 147 5.2.1 Integrating α-β-γ Processes in Inference . 149 5.2.2 Learning the α, β and γ Processes . 159 5.3 Example I: Integrating the α, β and γ for Image Parsing. 168 ii 5.3.1 Experiment I: Evaluating Information Contributions of the α, β and γ Processes Individually . 169 5.3.2 Experiment II: Object Parsing in a Greedy Pursuit Manner by Integrating the α, β and γ Processes . 176 5.4 Example II: Recognition on Object Categories . 176 6 Attributed And-Or Graph 179 6.1 Introduction of Attribute Grammar . 179 6.2 Attributed Graph Grammar Model . 180 6.3 Example I: Parsing the Perspective Man-made World . 181 6.4 Example II: Single-View 3D Scene Reconstruction and Parsing . 184 6.4.1 Attribute Hierarchy . 185 6.4.2 Attribute Scene Grammar . 189 6.4.3 Probabilistic Formulation for 3D Scene Parsing . 189 6.4.4 Inference . 194 6.5 Example III: Human-Centric Indoor Scene Synthesis Using Stochastic Grammar . 195 6.5.1 Representation . 196 6.5.2 Probabilistic Formulation . 196 6.5.3 Synthesizing Scene Configurations . 199 6.6 Example IV: Joint Parsing of Human Attributes, Parts and Pose . 200 6.7 Summarization . 204 7 Temporal And-Or Graph 205 7.1 Introduction . 205 7.2 Atomic Action Models . 206 7.2.1 2D HOI in Time - A Simplified Atomic Action Model . 207 7.2.2 Modeling Human Object Interaction in 3D and Time . 208 7.2.3 Part-Level 3DHOI . 213 7.2.4 Hand Object Interaction . 214 7.2.5 Concurrent HOI’s and hoi’s in STC-AOG . 215 7.3 Event representation by T-AOG . 216 7.3.1 The T-AOG for Events . 216 7.3.2 Parse Graph . 217 7.3.3 Example I: Synthesizing New Events by T-AoG . 218 7.3.4 Example II: Group Activity Parsing by ST-AoG . 218 7.4 Parsing with Event Grammars . 225 7.4.1 Formulation of Event Parsing . 225 7.4.2 Generating Parse Graphs of Single Events . 226 7.4.3 Runtime Incremental Parsing . 227 7.4.4 Generalized Earley Parser . 227 7.4.5 Multi-agent Event Parsing . 234 7.5 Learning the T-AoG . ..
Details
-
File Typepdf
-
Upload Time-
-
Content LanguagesEnglish
-
Upload UserAnonymous/Not logged-in
-
File Pages316 Page
-
File Size-