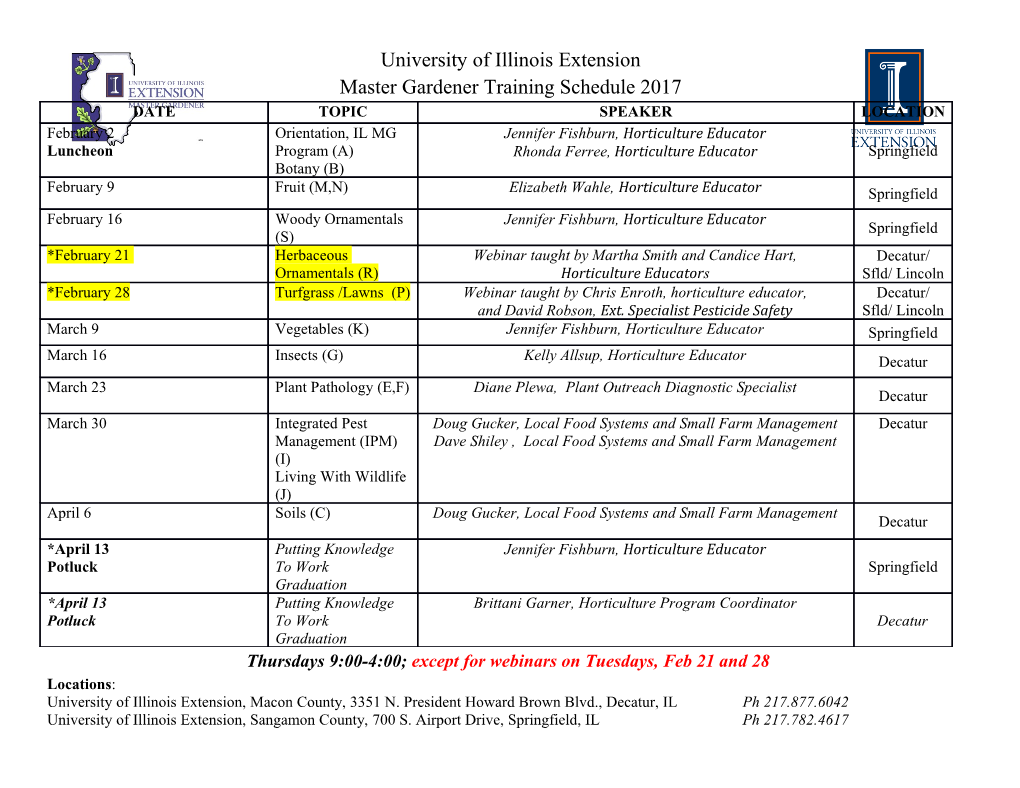
Robust Shrinkage Estimation of High-dimensional Covariance Matrices 1Yilun Chen, 2Ami Wiesel, 1Alfred O. Hero, III 1 Department of EECS, University of Michigan, Ann Arbor, USA 2 Department of CS, Hebrew University, Jerusalem, Israel fyilun,[email protected] [email protected] Abstract—We address high dimensional covariance estimation In this paper, we model the samples using the elliptical for elliptical distributed samples. Specifically we consider shrink- distribution; a flexible and popular alternative that encom- age methods that are suitable for high dimensional problems passes a large number of important non-Gaussian distributions with a small number of samples (large p small n). We start from a classical robust covariance estimator [Tyler(1987)], which in signal processing and related fields, e.g., [9], [13], [16]. is distribution-free within the family of elliptical distribution A well-studied covariance estimator in this setting is the ML but inapplicable when n < p. Using a shrinkage coefficient, we estimator based on normalized samples [7], [16]. The estimator regularize Tyler’s fixed point iteration. We derive the minimum is derived as the solution to a fixed point equation. It is mean-squared-error shrinkage coefficient in closed form. The distribution-free within the class of elliptical distributions and closed form expression is a function of the unknown true covariance and cannot be implemented in practice. Instead, its performance advantages are well known in the n p we propose a plug-in estimate to approximate it. Simulations regime. However, it is not suitable for the “large p small demonstrate that the proposed method achieves low estimation n” setting. Indeed, when n < p, the ML estimator does not error and is robust to heavy-tailed samples. even exist. To avoid this problem the authors of [10] propose an iterative regularized ML estimator that employs diagonal loading and use a heuristic for selecting the regularization I. INTRODUCTION parameter. They empirically demonstrated that their algorithm Estimating a covariance matrix (or a dispersion matrix) has superior performance in the context of a radar application. is a fundamental problem in statistical signal processing. Many techniques for detection and estimation rely on accurate estimation of the true covariance. In recent years, estimating a Our approach is similar to [10] but we propose a a system- high dimensional p × p covariance matrix under small sample atical choice of the regularization parameter. We consider a size n has attracted considerable attention. In these “large p shrinkage estimator that regularizes the fixed point iterations small n” problems, the classical sample covariance suffers of the ML estimator. Following Ledoit-Wolf [2], we provide from a systematically distorted eigen-structure, and improved a simple closed-form expression for the minimum mean- estimators are required. squared-error shrinkage coefficient. This clairvoyant coeffi- Many efforts have been devoted to high-dimensional co- cient is a function of the unknown true covariance and cannot variance estimation, which use Steinian shrinkage [1]–[3] or be implemented in practice. Instead, we develop a “plug-in” other types of regularized methods such as [4], [5]. However, estimate to approximate it. Simulation results demonstrate that most of the high-dimensional estimators assume Gaussian the our estimator achieves superior performance for samples distributed samples. This limits their usage in many important distributed within the elliptical family. Furthermore, for the applications involving non-Gaussian and heavy-tailed sam- case that the samples are truly Gaussian, we report very ples. One exception is the Ledoit-Wolf estimator [2], where little performance degradation with respect to the shrinkage the authors shrink the sample covariance towards a scaled estimators designed specifically for Gaussian samples [3]. identity matrix and proposed a shrinkage coefficient which is asymptotically optimal for any distribution. However, as the Ledoit-Wolf estimator operates on the sample covariance, it is The paper is organized as follows. Section II provides a inappropriate for heavy tailed non-Gaussian distributions. On brief review of elliptical distributions and Tyler’s covariance the other hand, traditional robust covariance estimators [6]–[8] estimation method. The regularized estimator is introduced and designed for non-Gaussian samples generally require n p derived in Section III. We provide simulations in Section IV and are not suitable for “large p small n” problems. Therefore, and conclude the paper in Section V. the goal of our work is to develop a covariance estimator for both problems that are high dimensional and non-Gaussian. Notations: In the following, we depict vectors in lowercase This work was partially supported by AFOSR, grant number FA9550-06- boldface letters and matrices in uppercase boldface letters. (·)T 1-0324. The work of A. Wiesel was supported by a Marie Curie Outgoing International Fellowship within the 7th European Community Framework denotes the transpose operator. Tr(·) and det(·) are the trace Programme. and the determinant of a matrix, respectively. II. ML COVARIANCE ESTIMATION FOR ELLIPTICAL final normalization step is needed, which ensures the iteration DISTRIBUTIONS limit Σb 1 satisfies Tr(Σb 1) = p. A. Elliptical distribution The ML estimate corresponds to the Huber-type M- estimator and has many good properties when n p, such x p×1 Let be a zero-mean random vector generated by the as asymptotic normality and strong consistency. Furthermore, following model it has been pointed out [7] that the ML estimate is the “most x = Ru; (1) robust” covariance estimator in the class of elliptical distri- where R is a positive random variable and u is a p × 1 zero- butions in the sense of minimizing the maximum asymptotic mean, jointly Gaussian random vector with positive definite variance. covariance Σ. We assume that R and u are statistically independent. The resulting random vector x is elliptically III. ROBUST SHRINKAGE COVARIANCE ESTIMATION distributed. Here we extend Tyler’s method to the high dimensional The elliptical family encompasses many useful distributions setting using shrinkage. It is easy to see that there is no in signal processing and related fields and includes: the solution to (5) when n < p (its left-hand-side is full rank Gaussian distribution itself, the K distribution, the Weibull whereas it right-hand-side of is rank deficient). This motivates distribution and many others. Elliptically distributed samples us to develop a regularized covariance estimator for elliptical are also referred to as Spherically Invariant Random Vectors samples. Following [2], [3], we propose to regularize the fixed (SIRV) or compound Gaussian vectors in signal processing point iterations as and have been used in various applications such as band- n T limited speech signal models, radar clutter echo models [9], p X si si Σb j+1 = (1 − ρ) + ρI (7) n T −1 and wireless fading channels [13]. i=1 si Σb j si Σb j+1 B. ML estimation Σb j+1 = ; (8) Tr(Σb j+1)=p Let fx gn be a set of n independent and identically i i=1 where ρ is the so-called shrinkage coefficient, which is a distributed (i.i.d.) samples drawn according to (1). The prob- constant between 0 and 1. When ρ = 0 the proposed shrinkage lem is to estimate the covariance (dispersion) matrix Σ from estimator reduces to Tyler’s unbiased method in (5) and when fx gn . To remove the scale ambiguity caused by R we i i=1 ρ = 1 the estimator reduces to the trivial estimator Σ = I. further constrain that Tr(Σ) = p. The term ρI ensures that Σb j+1 is always well-conditioned The commonly used sample covariance, defined as and thus allows continuation of the iterative process without n 1 X T restarts. Therefore, the proposed iteration can be applied Sb = xix ; (2) n i to high dimensional estimation problems. We note that the i=1 normalization (8) is important and necessary for convergence. is known to be a poor estimator of Σ, especially when the We now turn to the problem of choosing a good, data- samples are high-dimensional and/or heavy-tailed. dependent, shrinkage coefficient ρ. Following Ledoit-Wolf [2], Tyler’s method [7], [16] addressed this problem by working we begin by assuming we “know” the true covariance Σ. The with the normalized samples: optimal ρ that minimizes the minimum mean-squared error is x called the “oracle” coefficient and is s = i ; (3) i 2 kxik2 ρO = arg min E Σe (ρ) − Σ ; (9) ρ F for which the term R in (1) drops out. The pdf of si is given by [12] where Σe (ρ) is defined as −p=2 Γ(p=2) p −1 T −1 n T p(si; Σ) = · det(Σ ) · si Σ si : (4) p X sis 2πp=2 Σe (ρ) = (1 − ρ) i + ρI: (10) n sT Σ−1s n i=1 i i and the maximum likelihood estimator based on fsigi=1 is the solution to There is a closed-form solution to the problem (9) which is n T p X sis provided in the following theorem. Σ = · i : (5) n sT Σ−1s i=1 i i Theorem 1. For i.i.d. elliptical distributed samples, the solu- When n ≥ p, the ML estimator can be found using the tion to (9) is following fixed point iterations: Tr2(Σ) + (1 − 2=p)Tr(Σ2) ρO = : n T 2 2 2 p X sisi (1 − n=p − 2n=p )Tr (Σ) + (n + 1 + 2(n − 1)=p)Tr(Σ ) Σb j+1 = · ; (6) (11) n T −1 i=1 si Σb j si Proof: To ease the notation we define Ce as where the initial Σ is usually set to the identity matrix. It can b 0 n T p X sis be shown [7], [16] that the iteration process in (6) converges Ce = i : (12) n sT Σ−1s and does not depend on the initial setting of Σb 0.
Details
-
File Typepdf
-
Upload Time-
-
Content LanguagesEnglish
-
Upload UserAnonymous/Not logged-in
-
File Pages4 Page
-
File Size-