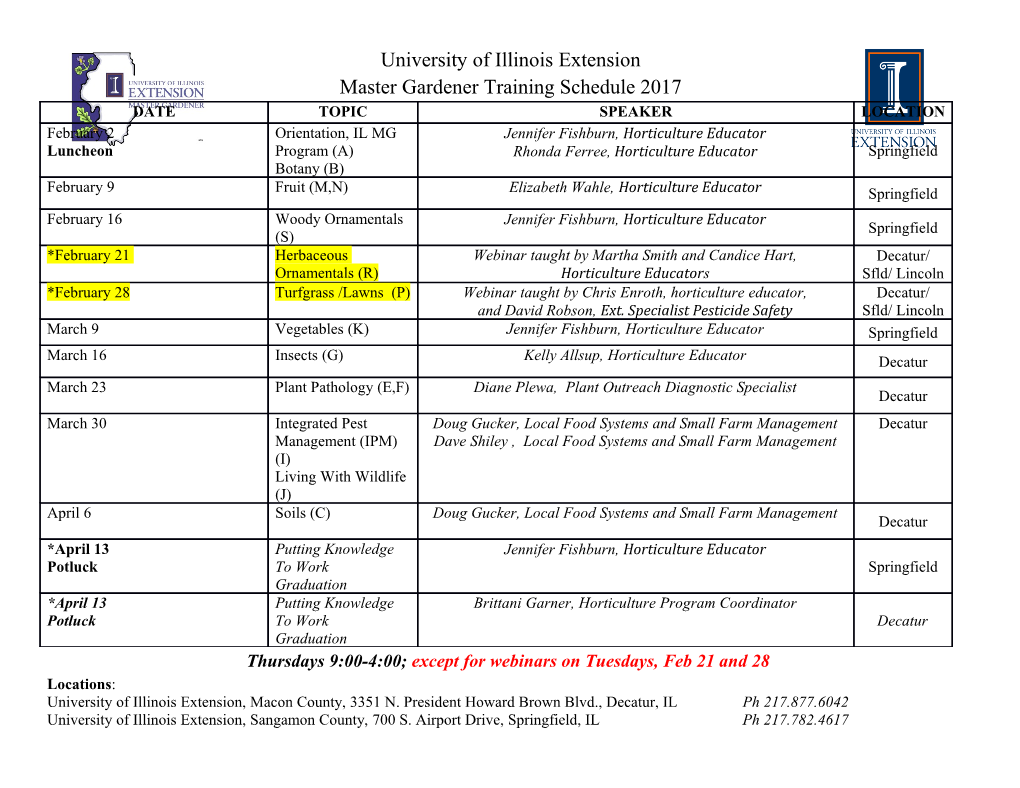
Random Cluster Dynamics for the Ising model is Rapidly Mixing Heng Guo (Joint work with Mark Jerrum) Durham Jul 29 2017 Queen Mary, University of London 1 The Model The random cluster model (Fortuin, Kasteleyn 69) Parameters 0 6 p 6 1 (edge weight), q > 0 (cluster weight). Given graph G = (V, E), the measure on subgraph r ⊆ E is defined as jrj jEnrj κ(r) πRC(r) / p (1 - p) q , where κ(r) is the number of connected components in (V, r). (1 - p)4q4 p2(1 - p)2q2 p4q 2 The random cluster model (Fortuin, Kasteleyn 69) The partition function (normalizing factor): X jrj jEnrj κ(r) ZRC(p, q) = p (1 - p) q . r⊆E Equivalent to the Tutte polynomial ZTutte(x, y): 1 q = (x - 1)(y - 1) p = 1 - y 3 The random cluster model (Fortuin, Kasteleyn 69) jrj jEnrj κ(r) πRC(r) / p (1 - p) q The motivation is to unify: • Ising model q = 2 • Potts model q > 2, integer • Bond percolation q = 1 (On Kn, Erdős-Rényi random graph) ! ! q ! • Electrical network q 0 (Spanning trees if p 0 and p 0) 4 The random cluster model (Fortuin, Kasteleyn 69) jrj jEnrj κ(r) πRC(r) / p (1 - p) q The motivation is to unify: • Ising model q = 2 • Potts model q > 2, integer • Bond percolation q = 1 (On Kn, Erdős-Rényi random graph) ! ! q ! • Electrical network q 0 (Spanning trees if p 0 and p 0) 4 The random cluster model (Fortuin, Kasteleyn 69) jrj jEnrj κ(r) πRC(r) / p (1 - p) q The motivation is to unify: • Ising model q = 2 • Potts model q > 2, integer • Bond percolation q = 1 (On Kn, Erdős-Rényi random graph) ! ! q ! • Electrical network q 0 (Spanning trees if p 0 and p 0) 4 The random cluster model (Fortuin, Kasteleyn 69) jrj jEnrj κ(r) πRC(r) / p (1 - p) q The motivation is to unify: • Ising model q = 2 • Potts model q > 2, integer • Bond percolation q = 1 (On Kn, Erdős-Rényi random graph) ! ! q ! • Electrical network q 0 (Spanning trees if p 0 and p 0) 4 The random cluster model (Fortuin, Kasteleyn 69) jrj jEnrj κ(r) πRC(r) / p (1 - p) q The motivation is to unify: • Ising model q = 2 • Potts model q > 2, integer • Bond percolation q = 1 (On Kn, Erdős-Rényi random graph) ! ! q ! • Electrical network q 0 (Spanning trees if p 0 and p 0) 4 The random cluster model (Fortuin, Kasteleyn 69) jrj jEnrj κ(r) πRC(r) / p (1 - p) q The motivation is to unify: • Ising model q = 2 • Potts model q > 2, integer • Bond percolation q = 1 (On Kn, Erdős-Rényi random graph) ! ! q ! • Electrical network q 0 (Spanning trees if p 0 and p 0) 4 Glauber dynamics Glauber dynamics (single edge update) PRC (Metropolis): Current state x ⊆ E 1. With prob. 1=2 do nothing. (Lazy) 2. Otherwise, choose an edge e u.a.r. { } 3. Move to y = x ⊕ feg with prob. min 1, πRC(y) . πRC(x) Detailed balance: π(x)P(x, y) = π(y)P(y, x) = minfπ(x), π(y)g 5 Glauber dynamics Glauber dynamics (single edge update) PRC (Metropolis): 8 { } > 1 min 1, πRC(y) if jx ⊕ yj = 1; < 2m πRC(x) { } P ⊕f g P (x, y) = 1 min , πRC(x e ) ; RC >1 - 2m e2E 1 π (x) if x = y :> RC 0 otherwise. We are interested in: { } jj t · jj 6 Tmix(PRC) = min t : PRC(x0, )- π TV ϵ , 1 Trel(PRC) = . 1 - λ2(PRC) 6 A simple example Let p < 1=2. { } π (x [ feg) min 1, RC 8 πRC(x) < p if e is not a cut edge = 1-p : p q(1-p) if e is a cut edge 7 A simple example Let p < 1=2. { } π (x [ feg) min 1, RC 8 πRC(x) < p if e is not a cut edge = 1-p : p q(1-p) if e is a cut edge 7 A simple example Let p < 1=2. { } π (x [ feg) min 1, RC 8 πRC(x) < p if e is not a cut edge = 1-p : p q(1-p) if e is a cut edge 7 A simple example Let p < 1=2. { } π (x [ feg) min 1, RC 8 πRC(x) < p if e is not a cut edge = 1-p : p q(1-p) if e is a cut edge 7 A simple example Let p < 1=2. { } π (x [ feg) min 1, RC 8 πRC(x) < p if e is not a cut edge = 1-p : p q(1-p) if e is a cut edge 7 Previous results Previous results focus on special graphs. • On the complete graph (mean-field): [Gore, Jerrum 99] [Blanca, Sinclair 15] • On the 2D lattice Z2: [Borgs et al. 99] [Blanca, Sinclair 16] [Gheissari, Lubetzky 16] q > 2: Slow mixing for the complete graph. 0 6 q 6 2: No known fast mixing bound for general graphs. 8 Main theorem Theorem For the random cluster model with parameters 0 < p < 1 and q = 2, 6 4 2 Trel(PRC) 8n m , 6 4 2 -1 -1 Tmix(PRC) 8n m (ln πRC(x0) + ln ϵ ). (n = #vertices, m = #edges.) • For q > 2, there exists p such that Tmix(PRC) is exponential on complete graphs. [Gore, Jerrum 99] [Blanca, Sinclair 15] [Gheis- sari, Lubetzky, Peres 17] • For q > 2 and 0 < p < 1, it is #BIS-hard to approximate ZRC(p, q). [Goldberg, Jerrum 12] • For 0 6 q < 2, there is no known obstacle. 9 Main theorem Theorem For the random cluster model with parameters 0 < p < 1 and q = 2, 6 4 2 Trel(PRC) 8n m , 6 4 2 -1 -1 Tmix(PRC) 8n m (ln πRC(x0) + ln ϵ ). (n = #vertices, m = #edges.) • For q > 2, there exists p such that Tmix(PRC) is exponential on complete graphs. [Gore, Jerrum 99] [Blanca, Sinclair 15] [Gheis- sari, Lubetzky, Peres 17] • For q > 2 and 0 < p < 1, it is #BIS-hard to approximate ZRC(p, q). [Goldberg, Jerrum 12] • For 0 6 q < 2, there is no known obstacle. 9 Main theorem Theorem For the random cluster model with parameters 0 < p < 1 and q = 2, 6 4 2 Trel(PRC) 8n m , 6 4 2 -1 -1 Tmix(PRC) 8n m (ln πRC(x0) + ln ϵ ). (n = #vertices, m = #edges.) • For q > 2, there exists p such that Tmix(PRC) is exponential on complete graphs. [Gore, Jerrum 99] [Blanca, Sinclair 15] [Gheis- sari, Lubetzky, Peres 17] • For q > 2 and 0 < p < 1, it is #BIS-hard to approximate ZRC(p, q). [Goldberg, Jerrum 12] • For 0 6 q < 2, there is no known obstacle. 9 Main theorem Theorem For the random cluster model with parameters 0 < p < 1 and q = 2, 6 4 2 Trel(PRC) 8n m , 6 4 2 -1 -1 Tmix(PRC) 8n m (ln πRC(x0) + ln ϵ ). (n = #vertices, m = #edges.) • For q > 2, there exists p such that Tmix(PRC) is exponential on complete graphs. [Gore, Jerrum 99] [Blanca, Sinclair 15] [Gheis- sari, Lubetzky, Peres 17] • For q > 2 and 0 < p < 1, it is #BIS-hard to approximate ZRC(p, q). [Goldberg, Jerrum 12] • For 0 6 q < 2, there is no known obstacle. 9 Swandsen-Wang algorithm Ferromagnetic Ising model (Ising, Lenz 25) A configuration σ : V ! f , g. Parameter β > 1. j j w(σ) = β mono(σ) Gibbs distribution: π(σ) ∼ w(σ). P Partition function: ZIsing(β) = σ w(σ). β4 β2 β0 2 C Exact evaluation of ZIsing is #P-hard even for β unless β = 0, 1, i. FPRAS for ZIsing for β > 1 [Jerrum, Sinclair 93] Efficient sampling [Randall, Wilson 99] 10 Ferromagnetic Ising model (Ising, Lenz 25) A configuration σ : V ! f , g. Parameter β > 1. j j w(σ) = β mono(σ) Gibbs distribution: π(σ) ∼ w(σ). P Partition function: ZIsing(β) = σ w(σ). β4 β2 β0 2 C Exact evaluation of ZIsing is #P-hard even for β unless β = 0, 1, i. FPRAS for ZIsing for β > 1 [Jerrum, Sinclair 93] Efficient sampling [Randall, Wilson 99] 10 Ferromagnetic Ising model (Ising, Lenz 25) A configuration σ : V ! f , g. Parameter β > 1. j j w(σ) = β mono(σ) Gibbs distribution: π(σ) ∼ w(σ). P Partition function: ZIsing(β) = σ w(σ). β4 β2 β0 2 C Exact evaluation of ZIsing is #P-hard even for β unless β = 0, 1, i. FPRAS for ZIsing for β > 1 [Jerrum, Sinclair 93] Efficient sampling [Randall, Wilson 99] 10 Equivalence at q = 2 1 Let β = 1-p . jEj ZIsing(β) = β ZRC (p, 2) Joint distribution on vertices and edges [Edwards, Sokal 88]: vertex colors assigned uniformly, edges chosen with prob. p, conditioned on no chosen edge is bichromatic. Marginal on vertices ) Ising model. Marginal on edges ) random cluster model. 11 Equivalence at q = 2 1 Let β = 1-p . jEj ZIsing(β) = β ZRC (p, 2) Joint distribution on vertices and edges [Edwards, Sokal 88]: vertex colors assigned uniformly, edges chosen with prob. p, conditioned on no chosen edge is bichromatic. Marginal on vertices ) Ising model. Marginal on edges ) random cluster model. 11 Equivalence at q = 2 1 Let β = 1-p . jEj ZIsing(β) = β ZRC (p, 2) Joint distribution on vertices and edges [Edwards, Sokal 88]: vertex colors assigned uniformly, edges chosen with prob. p, conditioned on no chosen edge is bichromatic. Marginal on vertices ) Ising model. Marginal on edges ) random cluster model.
Details
-
File Typepdf
-
Upload Time-
-
Content LanguagesEnglish
-
Upload UserAnonymous/Not logged-in
-
File Pages76 Page
-
File Size-