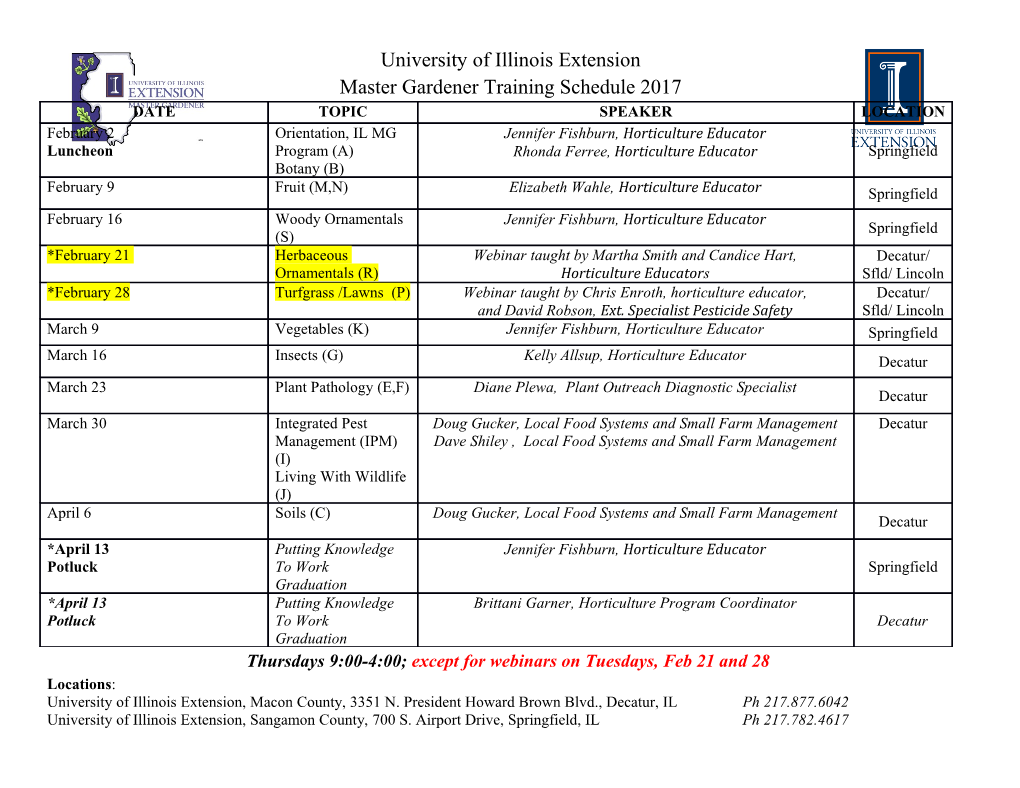
International Journal of Advanced Science and Technology Vol. 29, No. 3, (2020), pp. 10733 - 10739 ` Identification of Dialects: Survey S. Shivaprasad 1 Dr. M Sadanandam2 1 Research Scholar , Department of CSE, Kakatiya University ,Warangal & Assistant Professor Department of CSE ,VFSTR University, GUNTUR. 2Assistant Professor,Department of CSE , Kakatiya University ,Warangal 1 [email protected] ABSTRACT Automatic Dialect Identification plays a crucial role for constructing an Automatic speech recognition system in an appreciable manner in signal processing. We can mention dialect as property of a language that varies from standard version of that language depending upon the region. Dialect can be identified from speaker’s vocabulary, articulation, grammar and some other aspects like loudness, tonality and nasality. Identifying dialect exactly and properly will help in making some applications and services to work in an efficient manner such as e-learning and many such fields that is more helpful for homebound, aged ones. Dealing with dialect identification is very difficult due to factors like insufficient databases, subtle to regional boundaries, variations in languages. It is a tedious analysis procedure. Due to this factor dialect identification became crucial among research topics. Through this paper, we explain the process that is performed in identification various dialects and also the work done up to now, thereby providing information on what might be expected in coming years. Keywords: Dialects, Automatic Dialect Identification (ADI), Speech recognition Systems (SRS), automatic speech recognition ( ASR) 1. INTRODUCTION Language acts a medium of communication for humans. In speech recognition systems we use same language for various purposes. Dialect of language is considered as one of the problem for automatic speech recognition systems. Some speech recognition systems directly deal with the input audio and others deals the transformed audio signal. There is a need for finding the features of the audio signal to classify the audio. Recognition of audio signal based on dialects is next level for categorizing the speech recognition system. As of now many researchers has been working towards dialect recognition system in various languages. Dialect is defined as the language that has been habituated by the people of certain area. ISSN: 2005-4238 IJAST Copyright ⓒ 2020 SERSC 10733 International Journal of Advanced Science and Technology Vol. 29, No. 3, (2020), pp. 10733 - 10739 ` Automated dialect recognition systems are used to identify the dialect of a particular language. The training of the dialect recognition system is done by means of audio samples collected from a particular region. Automatic speech recognition systems has gained their importance in both academics and in industries [1]. It has a high impact on the society. Now-a-days speech recognition systems are given more importance by people rather than text formats because of having faster query processing as its advantage [3] [18]. Speech recognition systems are present now-a-days in electronic gadgets and there is a need for dialect recognition system for its improvement [19]. Dialect recognition systems are useful for people who are old and homebound by providing good e-health, telecommunication services. Day-by-day there has been an improvement in automated speech recognition systems. Dialect recognition systems will have an impact on speech recognition systems because it is adding an important feature for identifying the speaker [20]. In speech dialect is present in different segmental levels they are segmental, supra-segmental and sub-segmental. Developing a a good dialect recognition system may cause Improvements in applications that work with human interaction. Securing the communications that involve remote accessing. Refining the searches in electronic gadgets which work with the help of speech recognition systems. Dialect recognition system is considered as a more complex problem because there exists more likeness between vernaculars of same language [21]. Language tongues are utilized by the individuals to post status, speak with companions and other important aspects in social media. There is a requirement for new models to identify what is present in internet as well as news articles. Therefore, inorder to understand all these things dialect recognition system is considered as very important. 2. LITERATURE SURVEY George [1] conducted surveys in 1877 to identify the dialect. Barly [2] worked on Midland dialect whether it is presnt or not. He identified that the dialect will not deepends upon people prounciation of similar community region. From this, work on dialect is started. Imène GUELLIL, Faiçal AZOUAOU [3] worked on Arabic dialects identification and they identified new way about dialect identification. Thy applied techniques and algorithm that used in Algerian dialect. For this they had built and developed some dictionary of words that provides transfiguration between Algerian dialect and French with 25086 words. After that they proposed a calculation to cut down the messages in online networking into expressions and attempted to perceive every single expression by utilizing the words that were at that point developed in the dictionary. ISSN: 2005-4238 IJAST Copyright ⓒ 2020 SERSC 10734 International Journal of Advanced Science and Technology Vol. 29, No. 3, (2020), pp. 10733 - 10739 ` They considered mainly three techniques for identification. They are total, partial and by using Improved Levenshtein distance in which the terms in the constructed dictionary are identified but with differences of few letters. It included the length of the word thus differs from classical distance of Levenshtein. In order to apply their approach they relied on a corpus (collection) that was extracted using Facebook API from January 2015.They had considered a small corpus (with 100 messages in order to analyze their algorithm by focusing on the time taken to process such a huge collection [22] [23]. The outcomes of that algorithm were good enough and gave rating exceeding 60% for all three above mentioned types of identification with a distance less than or equal to 0.3, consequently with small differences in letters). It is to extend the work to EGY, TUN, Iraqi and other. To extend the dialect lexicon that is used by using an API on the net in order to collect the words from Algerian dialect. So, they startedwith a larger lexicon and enriched it [24]. Sreeraj V V Rajeev Rajan [4] had proposed new model consists feature-level fusion of MFCC (Mel Frequency Cepstral Coefficient) and TEO (teager energy operator). In classification phase a classifier based upon Support Vector Machine (SVM) is used. The systematic evaluation of the system which was proposed is executed upon a database containing the Malayalam dialect that was developed in studio environment. The database will have 4 dialects and each dialect will have 300 speech samples. The system based upon MFCC reported 65% of accuracy, system based upon TEO gave 73.33% of accuracy. The combined system exhibited some development with 78% of total accuracy. In [14], Dialect Identification of Assamese Language was proposed. Thy applied different methods like GMM and GMM-UBM. For the identidication purpose they created 13 hours and 30 minutes data of spontaneous speech. Even some sort of similarities is present between Goalparia,Kamrupi dialects and Assamese language, GMM-UBM provides good accuracy ISSN: 2005-4238 IJAST Copyright ⓒ 2020 SERSC 10735 International Journal of Advanced Science and Technology Vol. 29, No. 3, (2020), pp. 10733 - 10739 ` with 98.3% compare with GMM accuracy of 85.7%. So that thy conclude GMM-UBM is best modelling technique but they are not applied prosodic features and they does not check accuracy is incresed or decreased if database size is increased. Tanvira Ismail, Gaurab Krishnan Deka,[15] they are identifid Kamrupi Dialect with data set used is 10 hrs and 32 mins of spontaneous speech. These are applied GMM and GMM-UBM to identify the dialects. They identified GMM-UBM provides 99.5% compare to GMM with accuracy of 98.6% . Hence, they identified that GMM-UBM is better compared with GMM. However, they are not worked on the prosodic features . In [6],Mahnoosh Mehrabani proposed an analysis for identifying dialect/language sets automatically, which is based ontheprimitive differences between them. The differences were explored on the basis of the data that is available.. Initially, a method to measure the spectral acoustic differences between dialects was proposed which is on the basis of the volume space analysis within a 3D model. It uses MFCC derived log likelihood score distributions and Gaussian Mixture Models(GMM) [25]. Later, for studying the excitation structure differences among the dialects, energy and pitch contour primitives based text-independent prosody features were put forward. Dialect proximity measures were also proposed which were evaluated on Arabic dialects and on South languages of India. The measures presented by them are shown to be consistent and repeatable. However, they did not consider the lexical contrasts like word selection, grammatical structure or wider supra segmental differences for the evolution of specific dialects [26]. In [17], a user-friendly prototype was developed which can be installed on NAO robots to command them using speech. HMM-GMM was primarily adopted in this. The outcome from the experiments showed that a high accuracy
Details
-
File Typepdf
-
Upload Time-
-
Content LanguagesEnglish
-
Upload UserAnonymous/Not logged-in
-
File Pages7 Page
-
File Size-