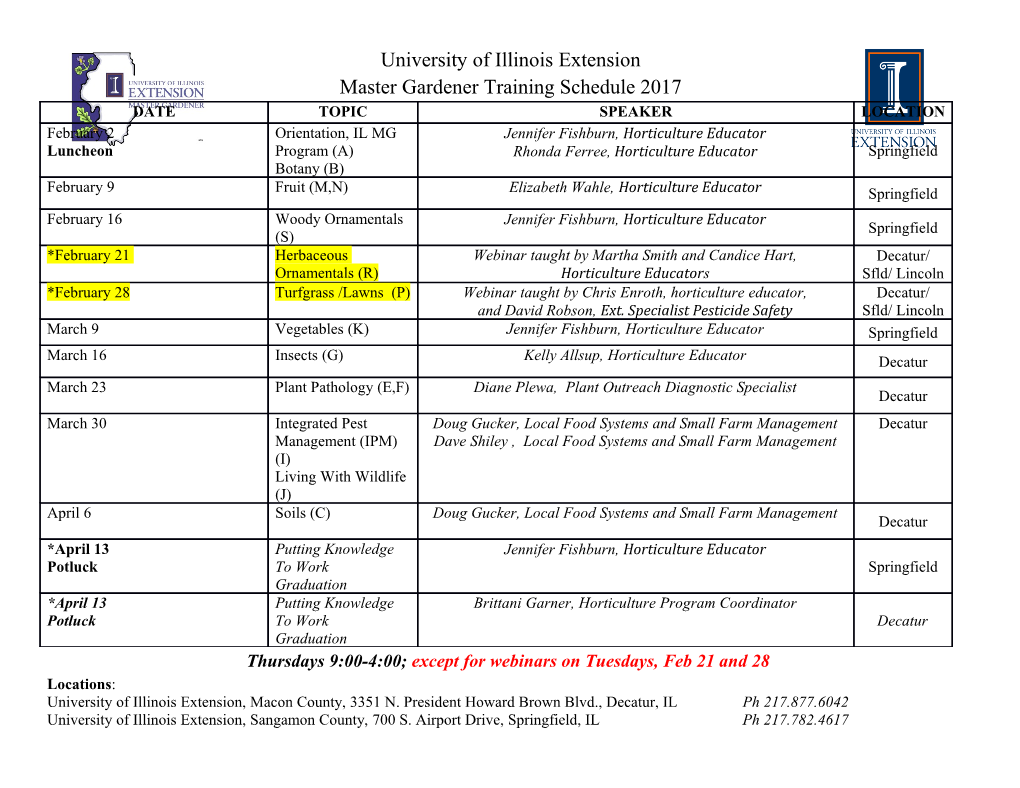
How to interpret and report the results from multivariable analyses Neus Valveny and Stephen Gilliver analyses the participating variables can be classified into: TFS Develop, Medical ● Dependent (or outcome or predicted) Writing Unit, Barcelona, variables and Spain / Lund, Sweden ● Independent (or predictor or explanatory) variables, which in some models can be further classified into factors and covariates (or confounding factors). In a bivariate analysis (sometimes Correspondence to: referred to as univariate – see Box 1 below) Neus Valveny there is only one independent and one TFS Develop dependent variable. Consell de Cent, 334-336, 4th floor ES-08009 Barcelona, Spain + 34 617 414 124 [email protected] Abstract Multivariable analyses are some of the mean, percentage). With central statistical methods of clinical univariate analyses we can trials, and yet some medical writers may only answer “descriptive be unsure as to what they are and how questions” in a single arm or best to interpret and report the results. In cohort, such as “What is the rate of this article we provide an overview of responders to drug X?” or “What is the multivariable analyses, introducing some mean survival time in patients treated of the core models biostatisticians use to with drug Y?” analyse trial data. We focus on odds But what about situations where we ratios, hazard ratios, and β coefficients as wish to analyse more than one variable at a key parameters and provide guidance on time? The purpose of bivariate and multi - important considerations when reporting variable analyses is to probe the relationships them. between two (bivariate) or more than two (multivariable) variables. These types of analyses allow us to test a previously defined What is a multivariable hypothesis (e.g. the primary efficacy analysis In a multivariable analysis there are: analysis? of a confirmatory study) or to explore the ● One dependent variable and Univariate analyses – analyses involving only existing relation ships between the collected ● Two or more independent variables. a single variable – are descriptive by nature. variables (e.g. between-arm analyses, sub - They allow us to describe the distribution of group analyses, exploratory analyses). With BOX 1: Bivariate analyses that analyse the a variable in a sample of n individuals or n bivariate and multivariable analyses we can relationship between one independent tumour biopsies, for example. In univariate answer “analytical questions” in one or more variable and one dependent variable are analyses we commonly use parameters such cohorts, such as “What is the overall survival often referred to as “univariate” analyses as the median, mean, and standard deviation with drug X compared with drug Y?”, “What to distinguish them from multivariable to describe quantitative (or continuous) is the efficacy of drug Z, based on the analyses, in which two or more variables and frequencies and percentages to reduction in cholesterol levels, compared independent variables are assessed in describe categorical variables. We can also with placebo?”, or “What is the relationship relation to a dependent outcome. In this estimate population parameters by calcul - between response rate to drug X and the context, the term “univariate” is correct ating 95% confidence intervals (CIs) for the level of biomarker Y?” and replaces the term “bivariate”. aforementioned summary statistics (median, In both bivariate and multivariable www.emwa.org Volume 25 Number 3 | Medical Writing September 2016 | 37 How to interpret and report the results from multivariable analyses – Valveny and Gilliver Multivariable analyses The dependent vari - we must also consider that some should not be con - able is the one that is independent variables may be entered in the fused with multivariate assessed with the model because they are confounding variables analyses, which are study. Sometimes it is (sometimes also denoted as covariates). used to assess the referred to as the Confounding variables are factors related to relationships of several endpoint. Usually this both the dependent and independent predictors with two or term is reserved for the variables. Unless we adjust our multivariable more dependent vari - combination of the out - analysis for confounding variables, we may ables or outcomes at the come plus the timepoint(s) end up with an inaccurate or incorrect same time. In this article we of assess ment (e.g. if the representation of the true relationships will not review multivariate outcome is “mortality”, the between the dependent and independent analyses. However, medical writers endpoint could be “mortality rate at 6 variables. For example, in many clinical trials should be aware that the terms multivariate months”). the baseline value for a quantitative and multivariable are often used inter - The independent variables define the outcome (e.g. baseline blood pressure in a changeably. Do not be surprised to see subgroups of patients in which the outcome hypertension trial) is a potential con - multivariable analyses described as will be compared (e.g. treatment arms). founding variable if it is not fully balanced multivariate. ● If the independent variable is categorical between the two treatment arms, despite To correctly interpret a multivariable (e.g. treatment arm, gender), the para - randomisation of the patients, because it is analysis it is highly recommendable to first meters of the multivariable models we also related to the outcome. For this reason, look at the bivariate analyses between the will review in later sections – the odds the primary efficacy analysis should always variables that were involved in the ratio (OR), hazard ratio (HR), and beta include the baseline value for the multivariable modelling. They show you: coefficient (β) – always estimate the quantitative outcome as a covariate. 1. the raw relationships between the depen - effect on the outcome of one or more dent and independent variables (which allow categories versus a reference category When to apply a multivariable the unadjusted associations to be quantified) (e.g. placebo or female gender), which analysis and 2. correlations or assoc iations between must be defined a priori. A multivariable analysis is needed in the independent variables (which, if present, ● If the independent variable is quan tit - following cases: may require changes to the model). ative (e.g. age), no subgroups are 1. If there is one main independent variable compared and the OR, β, and HR of interest (the other independent Variables: estimate the effect on the outcome of variables being secondary factors): Dependent vs independent / each 1-unit increase in the a. To evaluate the relationship between Quantitative vs categorical independent variable (e.g. “for each 1 the variable of interest and the out - It is very important to note that both the mg/dl increase in baseline cholesterol”). come after adjusting (or controlling) dependent and independent variables can It is very common for continuous for other independent variables that be either quantitative or categorical, and predictors to be transformed into categorical may also be related to the outcome correct identification of these statistical variables prior to the multivariable analysis (confounding factors or covariates). properties is essential for the medical using a previously defined cut-off point Examples: writer to correctly interpret and report the (from the literature). This is because the “Patients treated with drug A had results. parameters of the models are much easier significantly higher cholesterol levels at 6 Common quantitative outcomes include for physicians to interpret if they compare months compared to patients treated with cholesterol levels, blood pressure, and quest - one category to another than if they placebo, after adjustment for ionnaire scores and common categ orical inform about the risk associated baseline cholesterol.” outcomes are survival (yes/no), response to with a 1-unit increase in the “Higher biomarker X treatment, and presence/absence of a predictor. However, this leads to levels were significantly assoc - specific event (e.g. cardiovascular event, a loss of statistical power and to iated with a higher response relapse). the risk of not finding rate, independently of/after Common quantitative predictors include significant results. If the model adjusting for age and gender.” age, BMI, and baseline values for the includes the original con tinuous (Box 2 opposite) outcomes. Common categorical predictors predictor, the medical writer may 2. If there are two or more include treatment arm, gender, and baseline facilitate interpretation of the results main independent variables disease severity. by reporting the risk associated with, for of interest: Note that categorical variables with only example, a 10-unit increase in the predictor. a. To explore which of the independent two categories are ref erred to as dichotomous. In interpreting a multivariable analysis variables are independently associated 38 | September 2016 Medical Writing | Volume 25 Number 3 Valveny and Gilliver – How to interpret and report the results from multivariable analyses with the outcome, i.e. they keep a Please note that the words “independently potentially use multiple Cox regression. significant p-value in the model associated” and “independent factor/ Finally, multiple linear regression, despite the inclusion of other predictor” imply that a multi - multiple ANOVA, and ANCOVA are independent vari ables: variable
Details
-
File Typepdf
-
Upload Time-
-
Content LanguagesEnglish
-
Upload UserAnonymous/Not logged-in
-
File Pages6 Page
-
File Size-