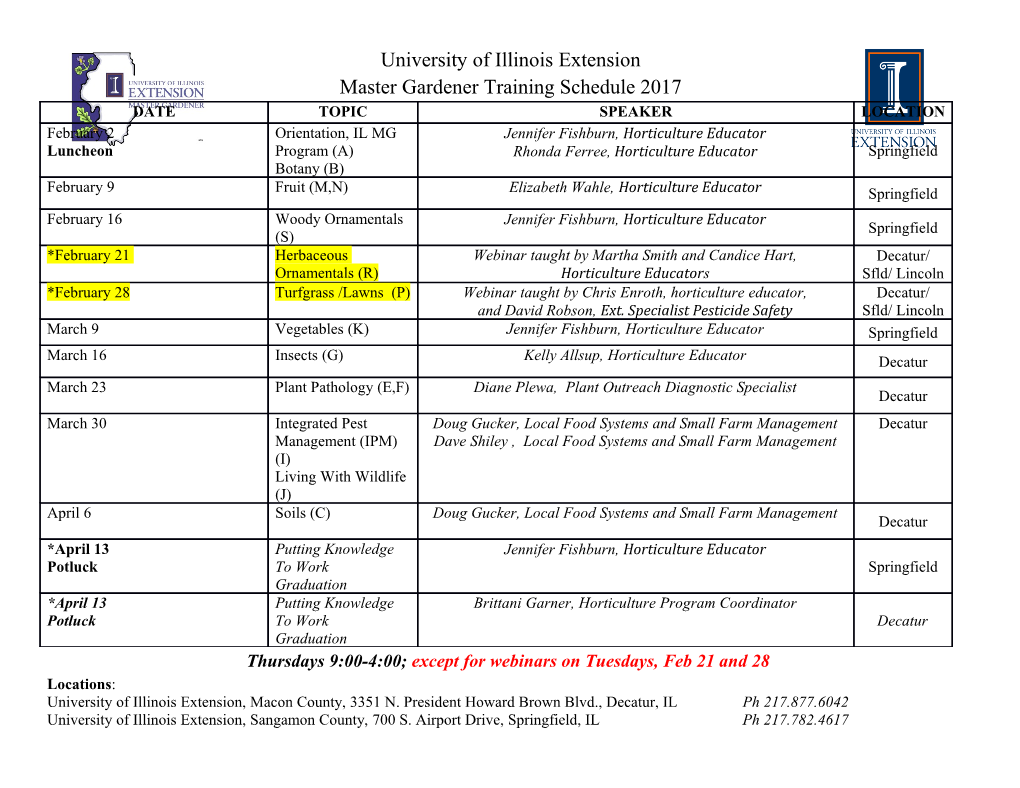
Mathematics of Risk Measures And the measures of the Basel Committee Master’s Degree Thesis MARTINA F. VAN RIJN Supervisors: Prof. Dr. Roberto Fernández Dr. Karma Dajani Utrecht, The Netherlands - Januari 2015 Student number: 0410985 Mathematics of Risk Measures And the measures of the Basel Committee by Martina F. van Rijn In partial fulfilment for the degree of Master of Science at the department of Mathematics, Utrecht University. Supervisors: Prof. Dr. Roberto Fernández Utrecht University Dr. Karma Dajani Utrecht University Abstract Risk measurement continues to be of the utmost importance in practice. The axiomatic approach to risk measures of Artzner et all. [3] gave rise to a whole new theory round this topic. With their axiomatic approach the class of coherent risk measures was completely characterized. In later years it became evident that coherent risk measures as defined by these axioms do not posses all of the properties desired in practice, since they are not necessarily robust. Robustness of risk measures is important to properly determine the underlying loss distribution through methods like backtesting. In 2013 Gneiting [19] published an article in which he described elicitable risk measures, this class of risk mea- sure is robust for (small) changes in the data. He showed that a necessary condition for elicitability is that of convex level sets, the question whether it is also sufficient still re- mains open. In my thesis I shall compare the properties of these two different classes of risk mea- sures, and argue that a risk measure should be both coherent and elicitable. Whereas there is a whole class of risk measures that are coherent or that are elicitable, there is only one such statistical functional that fits both criteria, the expectiles. Furthermore I will compare special cases of these classes by means of a simple, ran- dom foreign exchange portfolio. Which will show not only the mathematical differences but also the practical implications of choosing a risk measure. Since the practical implications where the key to further research on risk measures, I take a close look at the risk measures introduced by the Basel Committee on Banking Supervision and give a comparison to coherent, elicitable risk measures by means of the axioms they are based upon. I Acknowledgements This thesis was written as the final part of my master Mathematical Sciences at Utrecht University. I had the opportunity to start writing my thesis at the University of Malta as a visiting student. First of all I would like to thank my supervisors, prof. dr. Roberto Fernández and dr. Karma Dajani, for their helpful comments and good advice. Without their constant support and guidance I would not have materialized this thesis. I would also like to thank dr. Paul David Suda of the University of Malta for intro- ducing me to the topic of risk measures. And for his help during the first steps of this project. II Preface Baring’s Bank was the most prestigious bank of the United Kingdom, it collapsed in 1995 due to poor speculative investments in the Asian market done by one of its employers, Nick Leeson. Leeson falsified his losses, and reported to the bank and the British Tax Authorities that he was making substantial profits. He could no longer hide the losses when the Kobe earthquake sent the Asian financial markets into a tailspin. By the time the bank had discovered the losses made by Leeson, the total amount was more then $1.3 billion and the bank collapsed. Orange County, a suburban in California USA, had to file for bankruptcy in 1994 after heavy borrowing and risky investments resulting in a loss of $1.6 billion. This massive loss was the result of the risky trading strategy of the treasurer at that time, Bob Cit- ron. His trading strategy resulted in higher returns at first, but when the US government started a series of six consecutive interest rate hikes his strategy resulted in severe losses. Because of events such as the ones stated above it became evident that the risky posi- tions taken by banks should be monitored more accurately. Since the financial crisis of 2007/2008 the research on risk measures and the mea- surement of risk has become increasingly popular and the task of risk measurement has become increasingly complex. As in all situations when modelling complex issues and translating these into a workable model in practice, the difficulty lies in making a suffi- ciently realistic, but simple model. To quote Einstein: “ As simple as possible, but not simpler. ” III Contents Abstract I AcknowledgementsII Preface III Contents VI I Background1 1 Financial Risks2 1.1. Introduction . .2 1.2. Introduction Risk Measurement . .3 2 Axiomatic Approach to Risk Measures5 2.1. Acceptance Sets . .5 2.2. Risk Measures . .6 3 Classes of Risk Measures9 3.1. Coherent Risk Measures . .9 3.2. Elicitable Risk Measures . 12 II Risk Measures 15 4 Value-at-Risk 16 4.1. Definition Value-at-Risk . 16 4.2. Limitations of Value-at-Risk . 19 5 Expected Shortfall 22 5.1. Definition of Expected Shortfall . 22 IV 5.2. Limitations of Expected Shortfall . 26 6 Expectile Value-at-Risk 28 6.1. The Expectiles . 28 6.2. Definition of Expectile Value-at-Risk . 29 7 Simulation Study 32 7.1. Set-Up and Descriptive Statistics . 32 7.2. Results . 35 IIIThe Basel Accords 36 8 The Basel Committee 37 8.1. Establishment of the Basel Committee . 37 8.2. The Aim of the Basel Committee . 38 8.3. The Future of The Basel Committee . 39 9 Risk Measures of the Basel Accords 40 9.1. Basel I: the Basel Capital Accord . 40 9.2. Basel II: the New Capital Framework . 41 9.3. Basel III: the Liquidity Coverage Ratio & Liquidity Risk Monitoring Tools . 41 10 Natural Risk Statistics 44 10.1.Axiomatic Approach to Natural Risk Statistics . 44 10.2.Risk Measures vs Risk Statistics . 45 10.3.True Risk Measures Axioms vs Natural Risk Statistic Axioms . 46 IVOverview 48 11 Summary 49 12 Conclusion 51 13 Recommendations 53 Bibliography 56 V V Appendices 59 A Appendix 60 A.1. Quasi-Convexity . 60 A.2. Comonotonicity . 60 A.3. MLE for Pareto Distribution . 60 B Appendix 62 B.1. Currency Graphs . 62 C Appendix 65 C.1. List of All Jurisdictions Included in the BCBS . 65 VI Part I Background 1 Financial1 Risks 1.1 Introduction There are many kinds of financial risks against which institutions need to guard them- selves. In general you could divide them in five groups. Market Risk The risk of losses due to volatilities and movements of the financial market prices. These include interest rate risk, exchange rate risk, investment risk and many more. Credit Risk The risk of losses due to changes in the credit quality of the counterpar- ties. Counterparty default is the most extreme case, but losses can already occur when a counterparty’s credit merely decreases. Liquidity Risk The risk of losses due to travel-time of securities and assets. In other words, the risk that a given security or asset cannot be traded quickly enough in the market to prevent a loss. Operational Risk The risk of losses due to failed internal processes, the use of a wrong pricing model for instance. But also the risk of losses due to fraud and human mistakes. Legal & Regulatory Risk This type of risk includes losses due to changes in tax laws for instance. But also the risk of losses due to the lacking of appropriate licenses. Hence it is important to monitor the amount of risk a company is at, this is done by appointing a measure to a risky position. These risk measures can be classified into two categories, internal risk measures and external risk measures. Internal risk measurement is used at a level of individual institutions, in this case any institution is free to choose a risk model that best fits their beliefs and are allowed to choose which data they use. 2 While external risk measurement is used for external regulations and the same models are imposed for all relevant institutions. 1.2 Introduction Risk Measurement Since the 1997 article of Artzner et all. [2] risk measurement, and hence risk measures, have gained enormously in interest under economist, bank regulators and mathemati- cians, giving rise to a new theory. In my thesis I shall assume that risk measurement is a decision problem, ie a problem of the yes or no type as is also assumed in [2]. I find this approach most suitable since risk is ever present. The question therefore doesn’t concern the magnitude of the risk as much as the acceptability of the risky position. Definition 1.2.1 Risk is the future net worth of a position. At the beginning risk measurement was mainly focussed on the mathematical prop- erties which reflect the underlying economical meaning, however in the last years the statistical properties have become of increasing interest. Nowadays it is obvious to all working with risk, be it in practice or theory, that the procedure of risk measurement in fact involves two steps. (1) Estimating the loss distribution of the position. (2) Constructing a risk measure that summarizes the risk of the position. The position’s loss distribution in practice is generally unknown, and therefore must be estimated from (historical) data. The estimation is essentially done by backtesting. Recall that backtesting is the procedure of periodically comparing the forecasted risk measure with realized values in the financial market. Each one of the steps above should be regarded as equally important. Because risk measurement is of great practical importance, risk measures should be formalized with the regulations of the practical world in mind.
Details
-
File Typepdf
-
Upload Time-
-
Content LanguagesEnglish
-
Upload UserAnonymous/Not logged-in
-
File Pages77 Page
-
File Size-