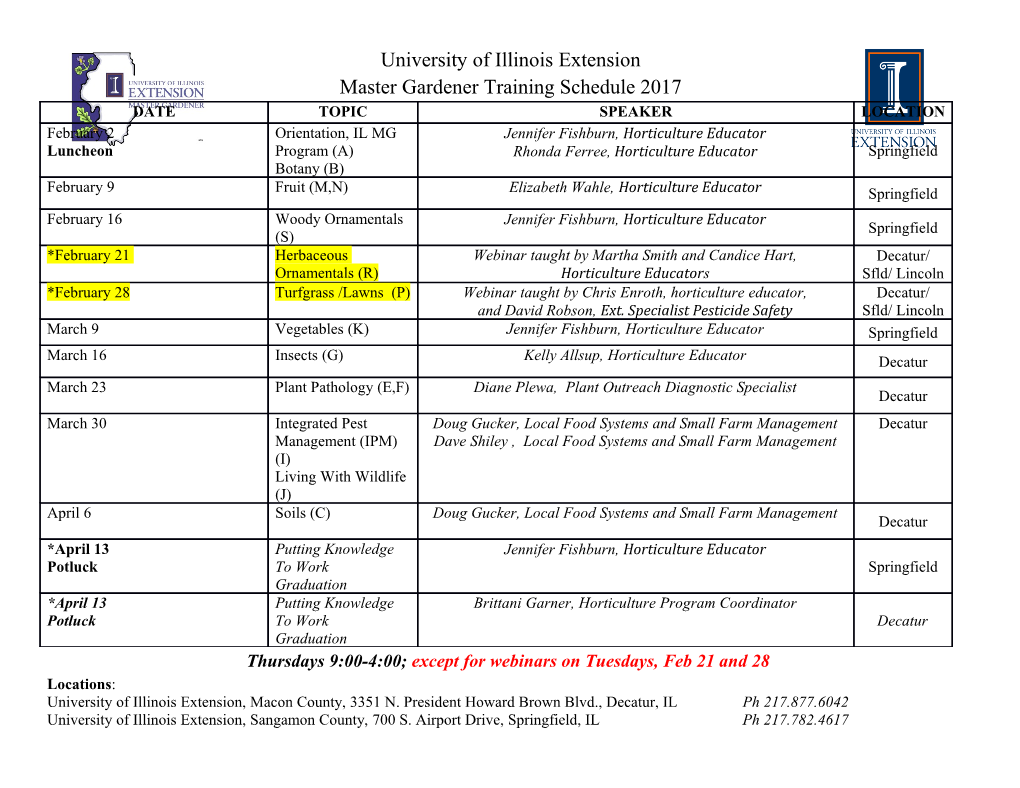
CS260: Machine Learning Algorithms Lecture 3: Optimization Cho-Jui Hsieh UCLA Jan 14, 2019 Optimization Goal: find the minimizer of a function min f (w) w For now we assume f is twice differentiable Machine learning algorithm: find the hypothesis that minimizes training error Convex function n A function f : R ! R is a convex function , the function f is below any line segment between two points on f : 8x1; x2; 8t 2 [0; 1]; f (tx1 + (1 − t)x2) ≤ tf (x1) + (1 − t)f (x2) Convex function n A function f : R ! R is a convex function , the function f is below any line segment between two points on f : 8x1; x2; 8t 2 [0; 1]; f (tx1 + (1 − t)x2)≤tf (x1) + (1 − t)f (x2) Strict convex: f (tx1 + (1 − t)x2) < tf (x1) + (1 − t)f (x2) Convex function Another equivalent definition for differentiable function: T f is convex if and only if f (x) ≥ f (x0) + rf (x0) (x − x0); 8x; x0 Convex function Convex function: (for differentiable function) rf (w ∗) = 0 , w ∗ is a global minimum If f is twice differentiable ) f is convex if and only if r2f (w) is positive semi-definite Example: linear regression, logistic regression, ··· Convex function Strict convex function: rf (w ∗) = 0 , w ∗ is the unique global minimum most algorithms only converge to gradient= 0 Example: Linear regression when X T X is invertible Convex vs Nonconvex Convex function: rf (w ∗) = 0 , w ∗ is a global minimum Example: linear regression, logistic regression, ··· Non-convex function: rf (w ∗) = 0 , w ∗ is Global min, local min, or saddle point (also called stationary points) most algorithms only converge to stationary points Example: neural network, ··· Gradient descent Generate the sequence w 1; w 2; ··· t converge to stationary points ( limt!1 krf (w )k = 0) Step size too large ) diverge; too small ) slow convergence Gradient Descent Gradient descent: repeatedly do w t+1 w t − αrf (w t ) α > 0 is the step size Step size too large ) diverge; too small ) slow convergence Gradient Descent Gradient descent: repeatedly do w t+1 w t − αrf (w t ) α > 0 is the step size Generate the sequence w 1; w 2; ··· t converge to stationary points ( limt!1 krf (w )k = 0) Gradient Descent Gradient descent: repeatedly do w t+1 w t − αrf (w t ) α > 0 is the step size Generate the sequence w 1; w 2; ··· t converge to stationary points ( limt!1 krf (w )k = 0) Step size too large ) diverge; too small ) slow convergence d ∗ will decrease f (·) if α (step size) is sufficiently small Why gradient descent? Successive approximation view At each iteration, form an approximation function of f (·): 1 f (w t + d) ≈ g(d) := f (w t ) + rf (w t )T d + kdk2 2α Update solution by w t+1 w t + d ∗ ∗ d = arg mind g(d) ∗ t 1 ∗ ∗ t rg(d ) = 0 ) rf (w ) + α d = 0 ) d = −αrf (w ) Why gradient descent? Successive approximation view At each iteration, form an approximation function of f (·): 1 f (w t + d) ≈ g(d) := f (w t ) + rf (w t )T d + kdk2 2α Update solution by w t+1 w t + d ∗ ∗ d = arg mind g(d) ∗ t 1 ∗ ∗ t rg(d ) = 0 ) rf (w ) + α d = 0 ) d = −αrf (w ) d ∗ will decrease f (·) if α (step size) is sufficiently small Illustration of gradient descent Illustration of gradient descent Form a quadratic approximation 1 f (w t + d) ≈ g(d) = f (w t ) + rf (w t )T d + kdk2 2α Illustration of gradient descent Minimize g(d): 1 rg(d ∗) = 0 ) rf (w t ) + d ∗ = 0 ) d ∗ = −αrf (w t ) α Illustration of gradient descent Update w t+1 = w t + d ∗ = w t −αrf (w t ) Illustration of gradient descent Form another quadratic approximation 1 f (w t+1 + d) ≈ g(d) = f (w t+1) + rf (w t+1)T d + kdk2 2α d ∗ = −αrf (w t+1) Illustration of gradient descent Update w t+2 = w t+1 + d ∗ = w t+1−αrf (w t+1) When will it diverge? Can diverge (f (w t )<f (w t+1)) if g is not an upperbound of f When will it converge? Always converge (f (w t )>f (w t+1)) when g is an upperbound of f Why? When α < 1=L, for any d, 1 g(d) = f (w t ) + rf (w t )T d + kdk2 2α L > f (w t ) + rf (w t )T d + kdk2 2 ≥ f (w t + d) So, f (w t + d ∗) < g(d ∗) ≤ g(0) = f (w t ) In formal proof, need to show f (w t + d ∗) is sufficiently smaller than f (w t ) Convergence Let L be the Lipchitz constant (r2f (x) LI for all x) 1 Theorem: gradient descent converges if α < L So, f (w t + d ∗) < g(d ∗) ≤ g(0) = f (w t ) In formal proof, need to show f (w t + d ∗) is sufficiently smaller than f (w t ) Convergence Let L be the Lipchitz constant (r2f (x) LI for all x) 1 Theorem: gradient descent converges if α < L Why? When α < 1=L, for any d, 1 g(d) = f (w t ) + rf (w t )T d + kdk2 2α L > f (w t ) + rf (w t )T d + kdk2 2 ≥ f (w t + d) In formal proof, need to show f (w t + d ∗) is sufficiently smaller than f (w t ) Convergence Let L be the Lipchitz constant (r2f (x) LI for all x) 1 Theorem: gradient descent converges if α < L Why? When α < 1=L, for any d, 1 g(d) = f (w t ) + rf (w t )T d + kdk2 2α L > f (w t ) + rf (w t )T d + kdk2 2 ≥ f (w t + d) So, f (w t + d ∗) < g(d ∗) ≤ g(0) = f (w t ) Convergence Let L be the Lipchitz constant (r2f (x) LI for all x) 1 Theorem: gradient descent converges if α < L Why? When α < 1=L, for any d, 1 g(d) = f (w t ) + rf (w t )T d + kdk2 2α L > f (w t ) + rf (w t )T d + kdk2 2 ≥ f (w t + d) So, f (w t + d ∗) < g(d ∗) ≤ g(0) = f (w t ) In formal proof, need to show f (w t + d ∗) is sufficiently smaller than f (w t ) When to stop? Fixed number of iterations, or Stop when krf (w)k < Applying to Logistic regression gradient descent for logistic regression Initialize the weights w0 For t = 1; 2; ··· Compute the gradient N 1 X ynxn rf (w) = − T N 1 + eynw xn n=1 Update the weights: w w − αrf (w) Return the final weights w Applying to Logistic regression gradient descent for logistic regression Initialize the weights w0 For t = 1; 2; ··· Compute the gradient N 1 X ynxn rf (w) = − T N 1 + eynw xn n=1 Update the weights: w w − αrf (w) Return the final weights w When to stop? Fixed number of iterations, or Stop when krf (w)k < Line Search: Select step size automatically (for gradient descent) Line Search In practice, we do not know L ··· need to tune step size when running gradient descent Line Search In practice, we do not know L ··· need to tune step size when running gradient descent Line Search: Select step size automatically (for gradient descent) A simple condition: f (w + αd) < f (w) often works in practice but doesn't work in theory A (provable) sufficient decrease condition: f (w + αd) ≤ f (w) + σαrf (w)T d for a constant σ 2 (0; 1) Line Search The back-tracking line search: Start from some large α0 α0 α0 Try α = α0; 2 ; 4 ; ··· Stop when α satisfies some sufficient decrease condition often works in practice but doesn't work in theory A (provable) sufficient decrease condition: f (w + αd) ≤ f (w) + σαrf (w)T d for a constant σ 2 (0; 1) Line Search The back-tracking line search: Start from some large α0 α0 α0 Try α = α0; 2 ; 4 ; ··· Stop when α satisfies some sufficient decrease condition A simple condition: f (w + αd) < f (w) A (provable) sufficient decrease condition: f (w + αd) ≤ f (w) + σαrf (w)T d for a constant σ 2 (0; 1) Line Search The back-tracking line search: Start from some large α0 α0 α0 Try α = α0; 2 ; 4 ; ··· Stop when α satisfies some sufficient decrease condition A simple condition: f (w + αd) < f (w) often works in practice but doesn't work in theory Line Search The back-tracking line search: Start from some large α0 α0 α0 Try α = α0; 2 ; 4 ; ··· Stop when α satisfies some sufficient decrease condition A simple condition: f (w + αd) < f (w) often works in practice but doesn't work in theory A (provable) sufficient decrease condition: f (w + αd) ≤ f (w) + σαrf (w)T d for a constant σ 2 (0; 1) Line Search gradient descent with backtracking line search Initialize the weights w0 For t = 1; 2; ··· Compute the gradient d = −∇f (w) For α = α0; α0=2; α0=4; ··· Break if f (w + αd) ≤ f (w) + σαrf (w)T d Update w w + αd Return the final solution w Stochastic Gradient descent 1 PN In general, f (w) = N n=1 fn(w), each fn(w) only depends on (xn; yn) Large-scale Problems Machine learning: usually minimizing the training loss N 1 X T minf `(w xn; yn)g := f (w) (linear model) w N n=1 N 1 X minf `(hw (xn); yn)g := f (w) (general hypothesis) w N n=1 `: loss function (e.g., `(a; b) = (a − b)2) Gradient descent: w w − η rf (w) | {z } Main computation Large-scale Problems Machine learning: usually minimizing the training loss N 1 X T minf `(w xn; yn)g := f (w) (linear model) w N n=1 N 1 X minf `(hw (xn); yn)g := f (w) (general hypothesis) w N n=1 `: loss function (e.g., `(a; b) = (a − b)2) Gradient descent: w w − η rf (w) | {z } Main computation 1 PN In general, f (w) = N n=1 fn(w), each fn(w) only depends on (xn; yn) Use stochastic sampling: Sample a small subset B ⊆ f1; ··· ; Ng Estimated gradient 1 X rf (w) ≈ rf (w) jBj n n2B jBj: batch size Stochastic gradient Gradient: N 1 X rf (w) = rf (w) N n n=1 Each gradient computation needs to go through all training samples slow when millions of samples Faster way to compute \approximate gradient"? Stochastic gradient Gradient: N 1 X rf (w) = rf (w) N n n=1 Each gradient computation needs to go through all training samples slow when millions of samples Faster way to compute \approximate gradient"? Use stochastic sampling: Sample a small subset B ⊆ f1; ··· ; Ng Estimated gradient 1 X rf (w) ≈ rf (w) jBj n n2B jBj: batch size Extreme case: jBj = 1 ) Sample one training data at a time Stochastic gradient descent Stochastic Gradient Descent (SGD) N Input: training data fxn; yngn=1 Initialize w (zero or random) For t = 1; 2; ··· Sample a small batch B ⊆ f1; ··· ; Ng Update parameter 1 X w w − ηt rf (w) jBj n n2B Stochastic gradient descent Stochastic Gradient
Details
-
File Typepdf
-
Upload Time-
-
Content LanguagesEnglish
-
Upload UserAnonymous/Not logged-in
-
File Pages57 Page
-
File Size-