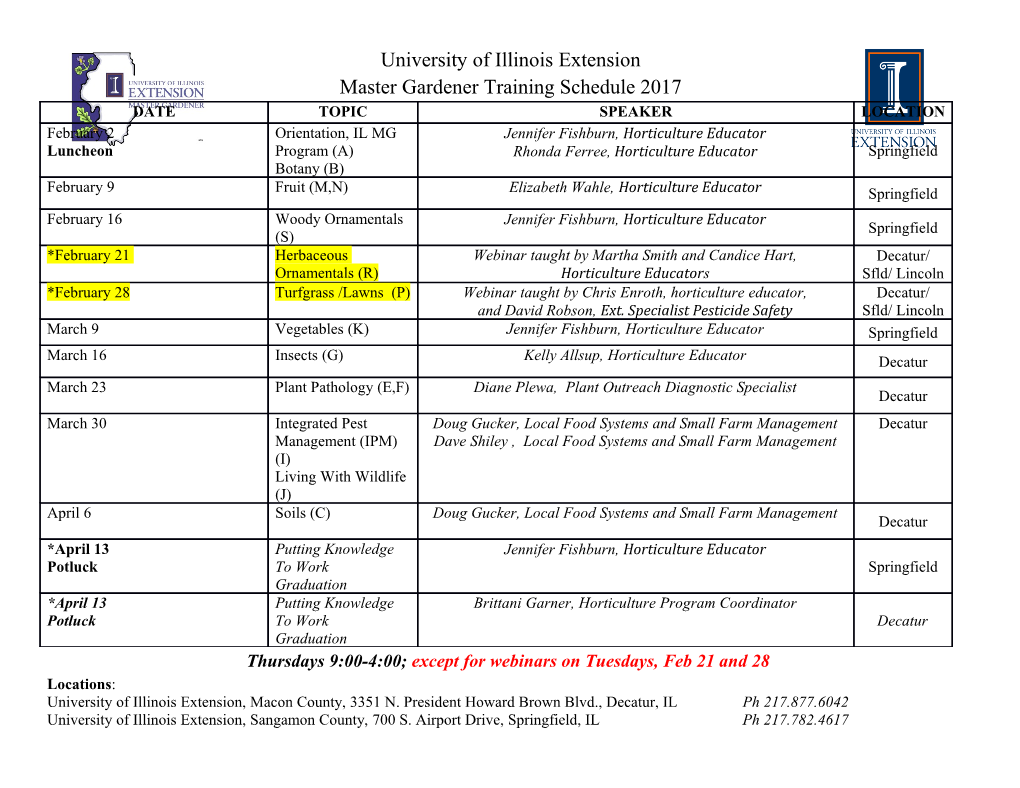
MAS programmes: Stochastic Models and Forecasting 1 Distribution theory and the Poisson process 1.1 Lifetime distributions Let the random variable (r.v.) T represent a lifetime, which might, for example, be the length of life of some animal or the time to failure of some machine component. It follows that T is necessarily a non-negative r.v., that is, a r.v. that can take only non-negative values. The distribution of such a r.v. T may be referred to as its lifetime distribution. Assume further that T is a continuous r.v. with p.d.f. f. Because T is a non-negative r.v., we shall need to specify f(t) for t ¸ 0 only, since f(t) = 0 for t < 0. In general, the (cumulative) distribution function (d.f.) F of a continuous r.v. T with p.d.f. f is de¯ned by Z t F (t) = P(T · t) = f(u)du t 2 R: ¡1 When T represents a lifetime, and hence is positive, 8 < 0 t < 0 F (t) = : : R t 0 f(u)du t ¸ 0 The survivor function Q(t) of the lifetime distribution is de¯ned by Z 1 Q(t) = P(T > t) = f(u)du t ¸ 0: t Thus Q(t) is the probability that an individual survives beyond age t. Note that Q(0) = 1, Q(t) = 1 ¡ F (t) t ¸ 0 and f(t) = F 0(t) = ¡Q0(t) t ¸ 0: (1) Consider an individual who has survived to age t. What is the probability that he dies in the next interval of time of length ±t? P(t < T < t + ±t; T > t) P(t < T < t + ±tjT > t) = P(T > t) P(t < T < t + ±t) = P(T > t) f(t)±t + o(±t) = : Q(t) 1 The hazard function r(t) (alternatively the mortality rate, force of mortality, age-speci¯c death rate, age-speci¯c failure rate or failure rate function, h(t) or ¸(t)) is the death rate at age t. More precisely, we de¯ne r(t) by P(t < T < t + ±tjT > t) r(t) = lim t ¸ 0: ±t!0 ±t Thus f(t) r(t) = t ¸ 0: (2) Q(t) From Equations (1) and (2), ¡Q0(t) d r(t) = = ¡ ln Q(t) t ¸ 0; (3) Q(t) dt which gives the hazard function in terms of the survivor function. Integrating Equation (3) and using the condition that Q(0) = 1, µ Z t ¶ Q(t) = exp ¡ r(u)du t ¸ 0; (4) 0 which gives the survivor function in terms of the hazard function. Example: the exponential distributions. If T has an exponential distribution with pa- rameter ¸ then f(t) = ¸e¡¸t t > 0; Z 1 Q(t) = ¸e¡¸udu = e¡¸t t > 0; t and ¸e¡¸t r(t) = = ¸ t > 0: e¡¸t The exponential distributions are the only distributions that have a constant hazard function. This fact expresses the \memoryless property" of the exponential distributions. No matter what the age of an item currently in use, the probability that it fails in the next interval of time of length ±t is the same, ¸±t + o(±t). ² Exponential distributions may be used to model, at least approximately, lengths of tele- phone calls and service times in some queueing systems. However, they would seem to be inappropriate to model human lifetime distributions (as far as we can tell in the current state of a®airs)! The memoryless property of exponential distributions is also expressed by the fact that if T has an exponential distribution then P(T > t + sjT > t) = P(T > s) ´ Q(s) s; t > 0: (5) 2 Equivalently, if the age of the item currently in use is t then the probability that it survives a further length of time at least s is Q(s), which does not depend upon t. The proof of Equation (5) is as follows. P(T > t + s; T > t) P(T > t + sjT > t) = P(T > t) P(T > t + s) Q(t + s) = = P(T > t) Q(t) e¡¸(t+s) = = e¡¸s = Q(s): e¡¸t Expectation The mean of the lifetime distribution, the expected length of the lifetime T , is given by Z 1 · Z x ¸ ¹ ´ E(T ) = tf(t)dt = lim tf(t)dt : 0 x!1 0 It will always be the case that ¹ > 0. It will usually be the case that ¹ is ¯nite, but it is possible that ¹ = +1. We now ¯nd an alternative expression for ¹ that often turns out to be useful in applications that involve lifetimes. Lemma 1.1.1 (weighted tail behaviour of distribution) If ¹ is ¯nite then xQ(x) ! 0 as x ! 1. Proof. Z 1 Z 1 0 · xQ(x) = xf(t)dt · tf(t)dt ! 0 x x as x ! 1, since ¹ is ¯nite. ¤ Theorem 1.1.2 (mean in terms of survivor function) Whether or not ¹ is ¯nite, Z 1 ¹ = Q(t)dt: 0 Proof. Integrating by parts, Z x Z x x Q(t)dt = [tQ(t)]0 + tf(t)dt 0 0 Z x = xQ(x) + tf(t)dt: 0 Letting x ! 1, and using the result of Lemma 1.1.1 if ¹ is ¯nite, and interchanging the order of integration on the L.H.S. otherwise, we obtain the result of Theorem 1.1.2. ¤ 3 De¯nition 1.1.3 (Maxima and minima of independent lifetimes) Let T1;T2;:::;Tn be independently distributed lifetimes, with Fi the distribution function and Qi the survivor function of Ti; i = 1; 2; : : : ; n. De¯ne the r.v.s V and W by V = maxfT1;T2;:::;Tng and W = minfT1;T2;:::;Tng: ² If, in some system, components with lifetimes T1;T2;:::;Tn are placed in parallel, so that the system functions as long as at least one of the components is still functioning, then what is critical for the functioning of the system is the maximum lifetime V . If the components are placed in series, so that the system fails as soon as one of the components fails, then what is critical for the functioning of the system is the minimum lifetime W . Now P(V · v) = P(T1 · v; T2 · v; : : : ; Tn · v) = P(T1 · v)P(T2 · v) ::: P(Tn · v); since T1;T2;:::;Tn are independently distributed. Thus the distribution function FV of V is given by Yn FV (v) = Fi(v) v ¸ 0: i=1 Similarly, P(W > w) = P(T1 > w; T2 > w; : : : ; Tn > w) = P(T1 > w)P(T2 > w) ::: P(Tn > w): Thus the survivor function QW of W is given by Yn QW (w) = Qi(w) w ¸ 0: i=1 Equivalently, the distribution function FW of W is given by Yn FW (w) = 1 ¡ (1 ¡ Fi(w)) w ¸ 0: i=1 Example: the exponential distributions. If T has an exponential distribution with pa- rameter ¸ then Z Z 1 1 1 E(T ) = Q(t)dt = e¡¸tdt = : 0 0 ¸ 4 Let T1;T2;:::;Tn be independently distributed lifetimes such that Ti has an exponential dis- tribution with parameter ¸i; i = 1; : : : ; n, and let W = minfT1;T2;:::;Tng: The survivor function QW of W is given by à ! Yn Xn QW (w) = exp(¡¸iw) = exp ¡ ¸iw w > 0: i=1 i=1 Pn Thus W has an exponential distribution with parameter i=1 ¸i. If there are n identical components, functioning simultaneously and each having an expo- nential lifetime distribution with parameter ¸, the length of time until the ¯rst failure of one of the components has an exponential distribution with parameter n¸. 1.2 Counting variables and probability generating functions Let N be a non-negative discrete random variable. The probability distribution of such a r.v. is speci¯ed by its probability mass function, the sequence (pn : n ¸ 0), where pn = P(N = n) n ¸ 0: Such a r.v. N commonly represents a count, e.g., the number of accidents that have occurred in a given period of time, the number of individuals in some population at a given point of time, or the number of customers who have joined a queue by a given time. 1.2.1 Probability generating functions | a review of their properties The probability generating function (p.g.f.) G(z) of N or of (pn) is de¯ned by X1 n G(z) = pnz : n=0 Equivalently, ¡ ¢ G(z) = E zN : P1 Because n=0 pn = 1, the p.g.f. is always de¯ned for at least jzj < 1, and G(1) = 1. Assuming that ¹ ´ E(N) and σ2 ´ var(N) are both ¯nite, ¯ ¯ dG(z)¯ ¹ = ¯ dz z=1 and ¯ d2G(z)¯ σ2 = ¯ + ¹ ¡ ¹2: 2 ¯ dz z=1 5 If N1;N2;:::;Nr are independently distributed r.v.s with p.g.f.s G1;G2;:::;Gr, respec- tively, and Sr = N1 + N2 + ::: + Nr then the p.g.f. GSr of Sr is given by Yr GSr (z) = Gi(z): i=1 1.2.2 Examples of p.g.f.s The Poisson distribution with parameter ¹, so that ¹n p = e¡¹ n ¸ 0; n n! has G(z) = e¹(z¡1): The binomial distribution with parameters K and p, so that µ ¶ K p = pnqK¡n n = 0; 1; : : : ; K; n n where q = 1 ¡ p, has G(z) = (pz + q)K : The shifted geometric distribution with parameter p, so that n pn = q p n ¸ 0; where q = 1 ¡ p, has p G(z) = : 1 ¡ qz 1.2.3 Expectation -revisited. The mean, the expectation, of the r.v. N is given by " # X1 Xr ¹ ´ E(N) = npn = lim npn : r!1 n=1 n=1 Note that 0 · ¹ · +1.
Details
-
File Typepdf
-
Upload Time-
-
Content LanguagesEnglish
-
Upload UserAnonymous/Not logged-in
-
File Pages13 Page
-
File Size-