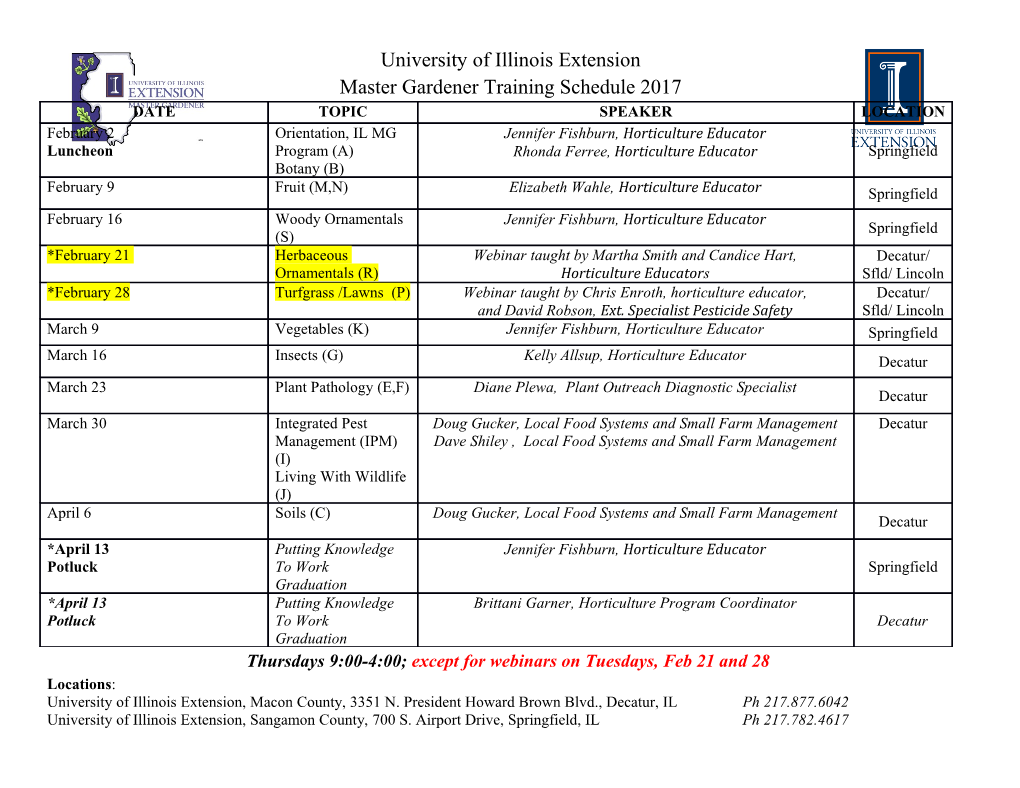
Machine Learning Models in Fullerene/Metallofullerene Chromatography Studies Xiaoyang Liu Thesis submitted to the faculty of the Virginia Polytechnic Institute and State University in partial fulfillment of the requirements for the degree of Master of Science In Computer Science and Application Yang Cao, Advisor Harry C. Dorn Lenwood S. Heath August 8, 2019 Blacksburg, VA 24060, U.S. Keywords: Machine learning, Neural Network, Chromatography, Fullerene, Modeling, Random Forest, XGBoost, Linear Regression, SVM regression, Nearest Neighbor Machine Learning Models in Fullerene/Metallofullerene Chromatography Studies Xiaoyang Liu ABSTRACT Machine learning methods are now extensively applied in various scientific research areas to make models. Unlike regular models, machine learning based models use a data-driven approach. Machine learning algorithms can learn knowledge that are hard to be recognized, from available data. The data-driven approaches enhance the role of algorithms and computers and then accelerate the computation using alternative views. In this thesis, we explore the possibility of applying machine learning models in the prediction of chromatographic retention behaviors. Chromatographic separation is a key technique for the discovery and analysis of fullerenes. In previous studies, differential equation models have achieved great success in predictions of chromatographic retentions. However, most of the differential equation models require experimental measurements or theoretical computations for many parameters, which are not easy to obtain. Fullerenes/metallofullerenes are rigid and spherical molecules with only carbon atoms, which makes the predictions of chromatographic retention behaviors as well as other properties much simpler than other flexible molecules that have more variations on conformations. In this thesis, I propose the polarizability of a fullerene molecule is able to be estimated directly from the structures. Structural motifs are used to simplify the model and the models with motifs provide satisfying predictions. The data set contains 31947 isomers and their polarizability data and is split into a training set with 90% data points and a complementary testing set. In addition, a second testing set of large fullerene isomers is also prepared and it is used to testing whether a model can be trained by small fullerenes and then gives ideal predictions on large fullerenes. Machine Learning Models in Fullerene/Metallofullerene Chromatography Studies Xiaoyang Liu GENERAL AUDIENCE ABSTRACT Machine learning models are capable to be applied in a wide range of areas, such as scientific research. In this thesis, machine learning models are applied to predict chromatography behaviors of fullerenes based on the molecular structures. Chromatography is a common technique for mixture separations, and the separation is because of the difference of interactions between molecules and a stationary phase. In real experiments, a mixture usually contains a large family of different compounds and it requires lots of work and resources to figure out the target compound. Therefore, models are extremely import for studies of chromatography. Traditional models are built based on physics rules, and involves several parameters. The physics parameters are measured by experiments or theoretically computed. However, both of them are time consuming and not easy to be conducted. For fullerenes, in my previous studies, it has been shown that the chromatography model can be simplified and only one parameter, polarizability, is required. A machine learning approach is introduced to enhance the model by predicting the molecular polarizabilities of fullerenes based on structures. The structure of a fullerene is represented by several local structures. Several types of machine learning models are built and tested on our data set and the result shows neural network gives the best predictions. ACKNOWLEDGEMENT I would like to express my appreciation to my advisor Dr. Young Cao for giving me the opportunity to pursue a master degree in computer science. Studying toward a computer science degree opens a new world for me. The project is in the intersection between computer science knowledge and my Ph.D. dissertation. I also thank my committee members, Dr. Harry Dorn and Dr. Lenwood Heath. I thank Dr. Harry Dorn for his support and understanding for my work and study in computer science. I thank Dr. Lenwood Heath for his help in discussion and revision of my thesis. It is my best luck to have the helpful committee members. iv Table of Content 1. Background ............................................................................................................................ 1 1.1 Chromatography .............................................................................................................. 4 1.2 High Performance Liquid Chromatography .................................................................... 8 1.3 HPLC in Fullerenes/Metallofullerenes Separations ....................................................... 20 2. Machine Learning Models Based on Molecular Geometry ................................................. 32 2.1 Machine Learning Models and Applications in Chemistry ........................................... 32 2.2 Feature Selection ............................................................................................................ 33 2.3 Model Selection ............................................................................................................. 37 3. Conclusion ........................................................................................................................... 45 References ................................................................................................................................ 46 v 1. Background Machine learning has been in its rapid development for the past few decades[1]. We may find machine learning in news feed, searching, and image recognition. Machine learning combines statistics and computer science and builds models to realize the tasks, which are hardly achieved by regular methods. Fundamentally, machine learning algorithms extract information or key features from available data and represent the data set using general models. There are numerous machine learning methods and it has been well established that different models are capable to be used for different tasks. Generally, there are two categories of tasks, regression and classification[2]. Most machine learning models can be modified for both tasks. Machine learning models are trained by raw data and then applied to infer things for unknown data. The training of machine learning models is a big challenge and nowadays, there are several methods to achieve sufficient training of models due to the development of algorithms and computing resources. Different machine learning methods have different structures to make them general for most tasks. The learning of machine learning models is a step to acquiring the hidden key features of data. The structures of knowledge from the raw data take different forms. For example, linear regression is a model to describe the linear relationship between features and the result data. Decision tree uses a different way of applying rules and applies a set of parameters to make decisions under different conditions. The application of a machine learning algorithm is also referred to as data mining, which is the process to obtain key information or features from a large amount of data. Among machine learning algorithms, neural network, or named as deep learning, is the most commonly used technique for scientific research. In the past few decades, various neural networks have been developed. For example, the convolutional neural network has shown its 1 power in image processing. Also, the ability to process an image or recognizing patterns is suitable to be converted to solve chemistry problems that involve chemical structures. Another essential feature to note is that deep learning can extract features automatically. One of the big challenges of machine learning is designing features, and designing features requires no only machine learning knowledge but also domain knowledge. Therefor deep learning is then versatile for different studies. Nowadays, with enough amount of data, deep learning models can defeat professional people in their areas. Behind the fancy results of machine learning, math plays an essential role. Machine learning algorithms, fundamentally, are built based on linear algebra, statistics, and programming. The first step of building machine models is to convert data into vectors, which is the language computer program can understand. Then training machine learning models is then to solve linear algebra systems following the given structures of machine learning algorithms. The starting point of many machine learning algorithms is the probability, and then the decision or result is estimated based on probability distributions or likelihood. A machine learning models have a certain structure, but contains several parameters, which are decided by the data. The way to learning information from the data set is to figure out the parameters suitable for the data set. Therefore, the training of a machine learning model is to optimize the parameter associated with the general machine learning model. The development of optimization algorithms has been a central topic in machine learning for decades. A typical method to handle a large amount
Details
-
File Typepdf
-
Upload Time-
-
Content LanguagesEnglish
-
Upload UserAnonymous/Not logged-in
-
File Pages56 Page
-
File Size-