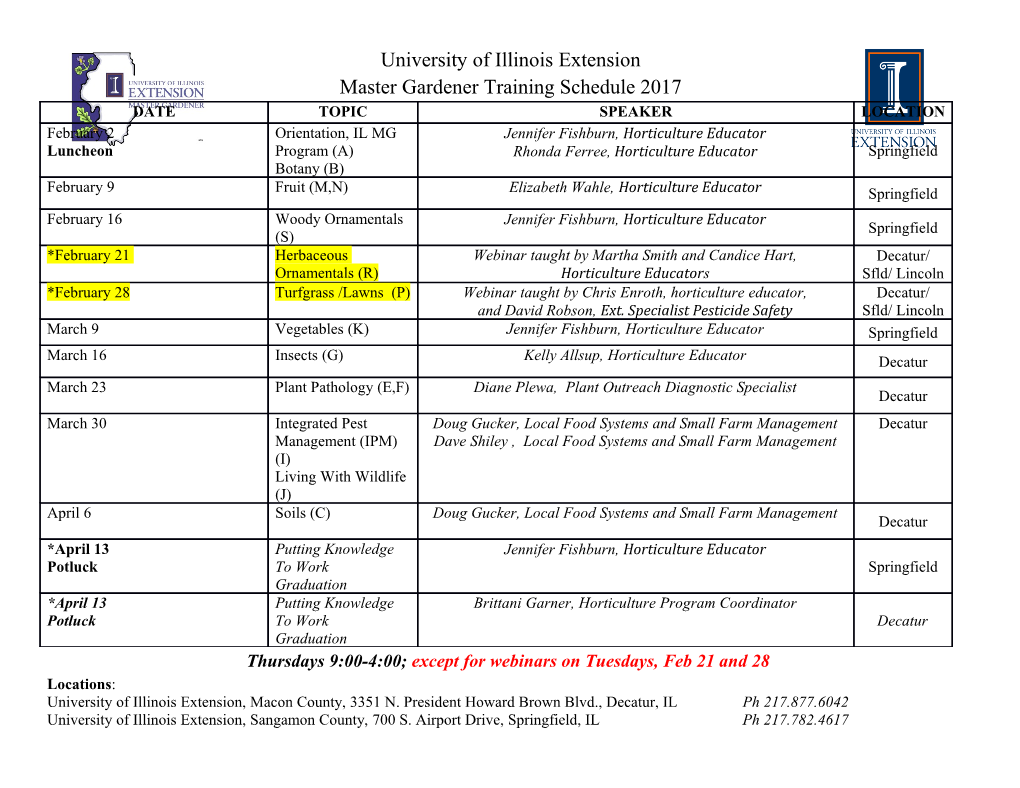
Xu et al. J Transl Med (2018) 16:274 https://doi.org/10.1186/s12967-018-1637-x Journal of Translational Medicine RESEARCH Open Access Molecular network‑based identifcation of competing endogenous RNAs and mRNA signatures that predict survival in prostate cancer Ning Xu1,2, Yu‑Peng Wu2, Hu‑Bin Yin1, Xue‑Yi Xue2 and Xin Gou1* Abstract Background: The aim of the study is described the regulatory mechanisms and prognostic values of diferentially expressed RNAs in prostate cancer and construct an mRNA signature that predicts survival. Methods: The RNA profles of 499 prostate cancer tissues and 52 non-prostate cancer tissues from TCGA were ana‑ lyzed. The diferential expression of RNAs was examined using the edgeR package. Survival was analyzed by Kaplan– Meier method. microRNA (miRNA), messenger RNA (mRNA), and long non-coding RNA (lncRNA) networks from the miRcode database were constructed, based on the diferentially expressed RNAs between non-prostate and prostate cancer tissues. Results: A total of 773 lncRNAs, 1417 mRNAs, and 58 miRNAs were diferentially expressed between non-prostate and prostate cancer samples. The newly constructed ceRNA network comprised 63 prostate cancer-specifc lncRNAs, 13 miRNAs, and 18 mRNAs. Three of 63 diferentially expressed lncRNAs and 1 of 18 diferentially expressed mRNAs were signifcantly associated with overall survival in prostate cancer (P value < 0.05). After the univariate and multivari‑ ate Cox regression analyses, 4 mRNAs (HOXB5, GPC2, PGA5, and AMBN) were screened and used to establish a predic‑ tive model for the overall survival of patients. Our ROC curve analysis revealed that the 4-mRNA signature performed well. Conclusion: These ceRNAs may play a critical role in the progression and metastasis of prostate cancer and are thus candidate therapeutic targets and potential prognostic biomarkers. A novel model that incorporated these candi‑ dates was established and might provide more powerful prognostic information in predicting survival in prostate cancer. Keywords: Competing endogenous RNAs, Prostate cancer, 4-mRNA signature, Survival Background for CRPC, including chemotherapy, androgen receptor- In men, prostate cancer remains the second leading cause targeted agents, and radiopharmaceuticals. Nevertheless, of deaths due to cancer in the US [1]. Approximately there are currently no efective biomarkers for the early 26,000 men were expected to die from prostate cancer in diagnosis and treatment of prostate cancer. 2016 [2]. Siegel et al. [2] also estimated that many patients Morphological, immunological, and molecular features with advanced prostate cancer will develop castration- have been used to predict the progression and prognosis resistant prostate cancer (CRPC). Previous studies [3–6] of prostate cancers [7, 8]. Over the past several decades, have reported that there are several treatment options urologists have devoted much efort toward identifying prostate cancer-related protein-coding genes [9]. How- ever, only approximately 2% of all transcripts in mam- *Correspondence: [email protected] 1 Department of Urology, The First Afliated Hospital of Chongqing mals are protein-coding RNAs [10]. Tus, the functions Medical University, No. 1 Youyi Rd., Yuzhong District, Chongqing 400016, of non-coding RNAs should be examined [11]. Previous China studies [12–16] proposed a competing endogenous RNA Full list of author information is available at the end of the article © The Author(s) 2018. This article is distributed under the terms of the Creative Commons Attribution 4.0 International License (http://creativecommons.org/licenses/by/4.0/), which permits unrestricted use, distribution, and reproduction in any medium, provided you give appropriate credit to the original author(s) and the source, provide a link to the Creative Commons license, and indicate if changes were made. The Creative Commons Public Domain Dedication waiver (http://creativecommons.org/ publicdomain/zero/1.0/) applies to the data made available in this article, unless otherwise stated. Xu et al. J Transl Med (2018) 16:274 Page 2 of 15 (ceRNA) hypothesis, which described an intricate post- www.targe tscan .org/), miRTarBase (http://mirta rbase transcriptional regulatory network in which mRNAs, .mbc.nctu.edu.tw/), and miRDB (http://www.mirdb lncRNAs, and other RNAs act as natural miRNA sponges .org/). Next, miRanda Tools (http://www.micro rna.org/ to weaken the function of miRNA via sharing one or micro rna/home.do) was used to predict the interactions more miRNA response elements. between lncRNAs and miRNAs. Finally, miRNAs that In this study, a ceRNA network was constructed to regulated the expression of both lncRNAs and mRNAs identify the ceRNAs that are involved in prostate can- were selected for construction of the ceRNA network cer using data from the TCGA database. Te RNA pro- using Cytoscape v.3.8.5. fles of 499 prostate cancer tissues and 52 non-prostate cancer tissues were analyzed. Finally, a prostate cancer- Survival analysis and defnition of mRNA‑related associated ceRNA network was established, based on prognostic model our bioinformatics prediction and correlation analysis, Te association between diferentially expressed mRNAs consisting of 63 lncRNAs, 13 miRNAs, and 18 mRNAs. and overall survival was evaluated by univariate Cox pro- We examined the functions of the diferentially expressed portional hazards regression analysis using the R survival miRNAs that we identifed and developed a novel model package. Only mRNAs with P < 0.01 were considered to using several candidates to predict survival in prostate be candidates and selected for multivariate Cox regres- cancer patients. Tis study aimed to identify prostate sion analysis. Te best explanatory and most informative cancer-specifc RNAs as ceRNAs that regulate target predictive model was identifed using Akaike Informa- genes and are involved in the pathogenesis and prognosis tion Criterion (AIC), which assesses the goodness of ft of of prostate cancer. a statistical model. Methods Gene ontology and pathway analysis Data collection To understand the underlying biological processes and RNA profles of prostate cancer and control samples were pathways between diferentially expressed genes in the downloaded from the genomic data commons (GDC) ceRNA network, the database for annotation, visualiza- data portal and the cancer genome atlas (TCGA, https tion, and integrated discovery (DAVID) (http://david ://tcga-data.nci.nih.gov/tcga/) database. A total of 551 .abcc.ncifc rf.gov/) was used to perform functional samples were collected, comprising 499 primary prostate enrichment analysis. Ten, signifcantly diferentially cancer samples and 52 normal solid tissue samples. expressed mRNAs were analyzed in the gene ontology (GO) database (http://www.geneo ntolo gy.org). Finally, Diferential gene expression analysis signifcantly enriched GO terms were selected to ana- mRNA, lncRNA, and miRNA expression in the prostate lyze their biological function. Te kyoto encyclopedia of cancer samples were analyzed using the RNASeqV2 and genes and genomes (KEGG; http://www.kegg.jp/) was Illumina HiSeq 2000 miRNA sequencing platforms. Sam- used to perform the pathway enrichment analysis. ples were divided into prostate cancer tissues versus adja- cent non-tumor tissues to identify diferentially expressed Survival analysis of key members in the ceRNA network RNAs using edgeR. Diferences in the expression of each Te clinical data on the patients were combined with RNA between prostate cancer and adjacent non-tumor prostate cancer data in TCGA to evaluate the prognostic tissue were expressed as fold-change and the associated value of diferential RNAs in the ceRNA network. Sur- P value. Downregulated and upregulated RNAs were vival curves were generated using the survival package in defned as those that decreased and increased by a fold- R for samples with diferentially expressed mRNAs, lncR- change of > 1.5, respectively, with an FDR-adjusted P of NAs, and miRNAs. Survival was analyzed by Kaplan– < 0.05. Meier method, and P values < 0.05 were considered to be signifcant. Construction of the ceRNA network Te regulatory network was constructed using data Results on the mRNAs, lncRNAs, and miRNAs. First, prostate Identifcation of signifcantly diferentially expressed cancer-specifc RNAs, including mRNAs, lncRNAs, and lncRNAs miRNAs, were fltered. Downregulated and upregu- In this study, 551 samples were obtained from the TCGA lated RNAs were assigned fold-changes > 1.5 with FDR- database. Diferential expression was analyzed by com- adjusted P < 0.05. Ten, the mRNAs that were targeted paring the expression of 14,254 lncRNAs in prostate can- by miRNAs were predicted using Targetscan (http:// cer and adjacent normal prostate tissues in the TCGA Xu et al. J Transl Med (2018) 16:274 Page 3 of 15 database. Fold-change > 1.5 and P value < 0.05 were set lncRNAs between prostate cancer and adjacent normal as cutofs to identify signifcantly diferentially expressed prostate tissue were obtained—of which 414 were upreg- lncRNAs. As a result, 773 diferentially expressed ulated and 359 were downregulated (Fig. 1; Table 1). Fig. 1 Heat maps of diferentially expressed messenger RNAs (mRNAs) in prostate cancer Xu et al. J Transl Med (2018) 16:274 Page 4 of 15 Table 1 Top diferential mRNAs for prostate cancer cancer patients was analyzed by Kaplan–Meier method. logFC logCPM P value FDR Tree of 63 diferentially expressed lncRNAs were linked to the prognosis in prostate cancer: LINC00355 SERPINA5
Details
-
File Typepdf
-
Upload Time-
-
Content LanguagesEnglish
-
Upload UserAnonymous/Not logged-in
-
File Pages15 Page
-
File Size-