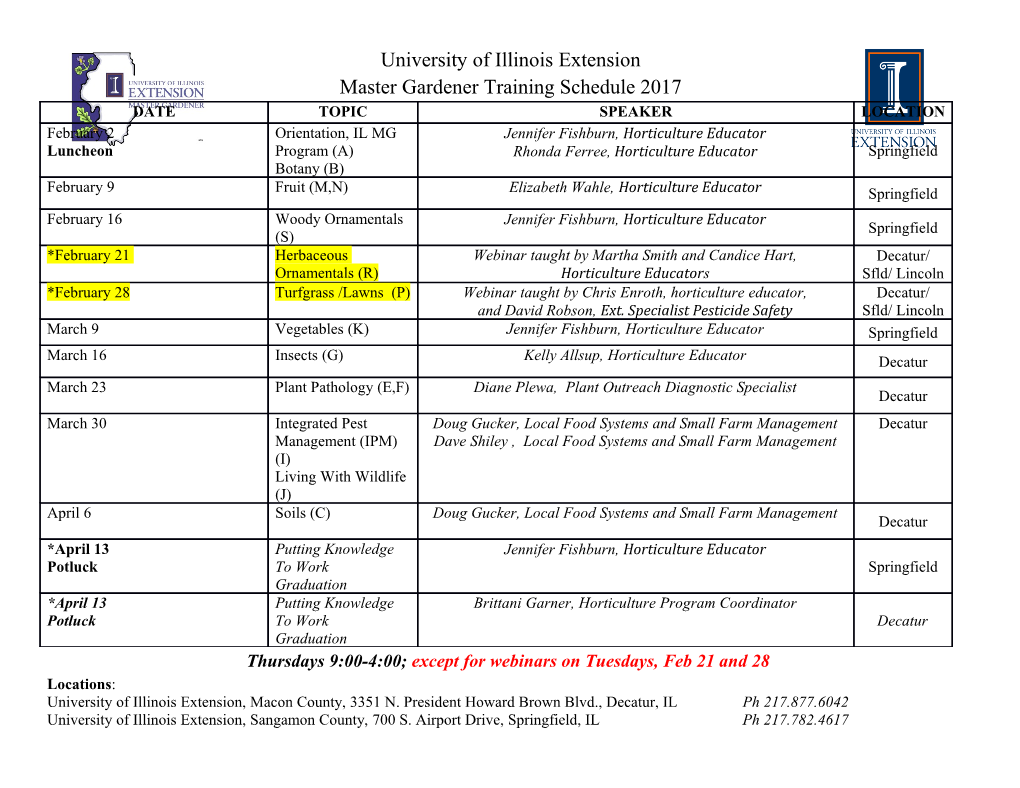
243 Abstract—Improved methods for The use of sampling weights in regression estimating saltwater recreational fishing catch and effort have been models of recreational fishing-site choices developed by the NOAA National Marine Fisheries Service. Sampling 1 weights that account for a complex Sabrina J. Lovell (contact author) sample design in surveys of anglers David W. Carter2 are now available with NMFS catch and effort estimates. Previously, es- Email address for contact author: [email protected] timates of the economic value to an- glers (known as the “willingness to 1 Economics and Social Analysis Division pay”) for additional fish caught that Office of Science and Technology were based on angler surveys did National Marine Fisheries Service, NOAA not typically account for the under- 1315 East-West Highway lying complex sample design. In this Silver Spring, Maryland 20910-3282 study, a recreational-demand model 2 was used for analysis of fishing site Southeast Fisheries Science Center choices in the Gulf of Mexico in 2009 National Marine Fisheries Service, NOAA among private-boat anglers who 75 Virginia Beach Drive target groupers (Epinephelus spp., Miami, Florida, 33149-1003 Hyporthodus spp., or Mycteroper- ca spp.) or red snapper (Lutjanus campechanus). Different versions of the model were developed with and without accounting for the complex sample design. Results between the In 2012, the NOAA National Marine rate survey, the NMFS Coastal unweighted version and weighted Fisheries Service (NMFS) released Household Telephone Survey (CHTS), versions of the model varied in esti- a new method for estimation of rec- that is used to estimate fishing effort mates of catch between sites and the reational fishing catch and effort by coastal residents by state, wave value anglers place on being able to based on data obtained from its Ac- (defined as a consecutive 2-month pe- catch and keep additional fish. cess Point Angler Intercept Survey riod), and fishing mode (private boat (APAIS) of saltwater anglers. Previ- and shore). Data from the APAIS on ous methods of estimation of catch the proportion of angler effort from and fishing effort from this intercept coastal residents to angler effort [interview] survey were subject to a from noncoastal and out-of-state res- number of different potential biases idents are used to scale the level of as pointed out by the National Re- angler effort from coastal residents search Council of the National Acad- up to an unbiased estimate of total emies (NRC, 2006). In particular, the effort for all anglers, both coastal earlier estimation methods did not and noncoastal. For example, 85% of account for the complex sample de- private boat trips that targeted grou- sign of the intercept survey and in- pers and red snapper in the Gulf of stead simple random sampling was Mexico in 2009 were taken by resi- assumed. The new method of esti- dents of coastal counties. Inclusion mating catch and effort uses special- of the APAIS sampling weights in ly calculated weights and variance recreational site-choice demand mod- adjustments (Breidt et al.1). els will ensure that results correctly The APAIS sampling weights in- reflect the true proportion of trips corporate information from a sepa- that come from coastal residents compared with trips from noncoastal 1 Breidt, F. J., H.-L. Lai., J. D. Opsomer, and D. A. Van Voorhees. 2012. A residents. This inclusion is important report of the MRIP sampling and because the costs associated with Manuscript submitted 2 May 2013. estimation project: improved estimation traveling between an angler’s home Manuscript accepted 13 June 2014. methods for the Access Point Angler and different fishing sites used in Intercept Survey component of the Fish. Bull. 112:243–252 (2014). the demand models will vary on the doi:10.7755/FB.112.4.1 Marine Recreational Fishery Statistics Survey. [Available from Fisheries basis of proximity to the coast. Statistics Division, Natl. Mar. Fish. A number of recreational site- The views and opinions expressed or Serv., NOAA, Silver Spring, MD, and choice demand models have been implied in this article are those of the from http://www.countmyfish.noaa.gov/ author (or authors) and do not necessarily projects/downloads/Final%20Report%20 developed with the APAIS data (e.g., reflect the position of the National of%20New%20Estimation_Method_for_ Whitehead and Haab, 2000; Gentner, Marine Fisheries Service, NOAA. MRFSS_Data-01242012.pdf.] 2007; Haab et al., 2012). These mod- 244 Fishery Bulletin 112(4) els examine how anglers make choices about which made (e.g., day). Following Whitehead and Habb (2000) fishing sites to visit on the basis of the costs of trav- and Gentner (2007), we limited the options available to el to a site and the qualities of a site. For models of each angler to trips at locations within a 300-mi (483 recreational fishing, site quality is typically measured km) round trip from an angler’s residence. We also as- by the average harvest rate per angler at a site. The sumed that the angler had already decided to fish from parameters of site-choice models are used to estimate a private boat and had decided which species to target economic values associated with recreational fishing. so that the primary choice was where to launch the However, failure to account for the complex sampling boat. In our model, this choice is made by comparing design of the NMFS APAIS survey could result in bi- the benefits or utility available from each potential ased demand-model parameters. It is important to launch site against the costs of getting to each site. have unbiased model parameters to obtain accurate es- The indirect utility, Uj, of going to site j for angler i timates of benefits and costs and to ensure that policy can be written as recommendations are not misleading. The APAIS data are collected by using a stratified, Uji = vji (qj, mi − cji) + εji, (1) multistage survey design with stratification that is where m = income; and based on intercept site, time of year, and other vari- i ables. Therefore, the proportion of anglers interviewed for angler i at site j: at each intercept site may reflect sampling allocations v = the observable portion of utility; and not necessarily reflect angler preferences or the ij cij = the trip cost; demand for one site over another. This problem of de- qj = a vector of attributes that defines the qual- mand estimation is commonly referred to as endogenous ity of fishing and other site features; and stratification. Another issue with demand estimation εij = the error term that represents the unob- can arise when using APAIS data because more avid served (to the analyst) portion of utility. users tend to be overrepresented in intercept surveys. This problem, referred to as avidity bias, can cause de- The observable portion of utility, such as travel costs mand-model parameters to be influenced more heavily and site characteristics (harvest rates for a site) or oth- by avid users. Hindsley et al. (2011), using simulated er site amenities (such as those at a marina), is based data sets, found evidence of both endogenous stratifica- on those attributes that can be observed and measured tion and avidity bias. However, their analysis was per- by the analyst. The unobserved portion includes infor- formed before the new NMFS estimation methods were mation on characteristics of the site or angler that are available. The sampling information made available unavailable to the analyst, for example, the presence through the updated estimation methods can be used of a tackle shop near a site or the number of years of to generate sampling weights to correct for endogenous experience an angler has at a given site. stratification in recreational site-choice demand models Under the assumptions of the random utility model developed with data from the APAIS. (McFadden, 1974), an angler will choose the site that The goal of our analysis was to compare the esti- provides the greatest level of utility: mates of parameters and economic value that result from the use of a typical NMFS recreational site-choice Vij (qj, mi − cij) + εij ≥ Vis(qs, mi − cis) + εis ∀ j ≠s, (2) demand model with and without the newly available sampling weights designed to correct for endogenous where V = the utility function; and stratification. We used a model of fishing site choices j = a member of s recreation sites. among private-boat anglers in the Gulf of Mexico who Assuming that the observed portion of utility is linear, target groupers (Epinephelus spp., Hyporthodus spp., or Vij (qj, mi − cij) = bqqj + bccij, and the unobserved por- Mycteroperca spp.) or red snapper (Lutjanus campecha- tions of utility, εj, have a type-I extreme value distri- nus). Following Kuriyama et al. (2013), we focus on the bution, the probability that angler i chooses site j can correction for endogenous stratification and do not at- be estimated with a standard conditional logit model: tempt to correct for potential avidity bias. More details on avidity bias and how to correct for it are given in exp(βqqj + βccij) Pij = P(yi = j) = J , (3) Thomson (1991) and Hindsley et al. (2011). ∑ exp(βqqj + βccij) s=1 where yi = the choice made by angler I; and Materials and methods bq and bc are parameters to be estimated. The parameters of the conditional logit model are typi- Model specification and estimation of angler willingness cally estimated through the use of maximum likelihood to pay with the following log-likelihood expression: The standard recreational discrete choice model that N J LL(β) = ∑ ∑ dijlogPij, (4) uses APAIS data has the angler choosing a preferred i=1 j=1 fishing site on any given occasion when a choice can be where N = the number of anglers in a sample; and Lovell and Carter: Sampling weights in regression models of recreational fishing-site choice 245 dij = 1 if angler i chooses site j and 0 otherwise.
Details
-
File Typepdf
-
Upload Time-
-
Content LanguagesEnglish
-
Upload UserAnonymous/Not logged-in
-
File Pages10 Page
-
File Size-