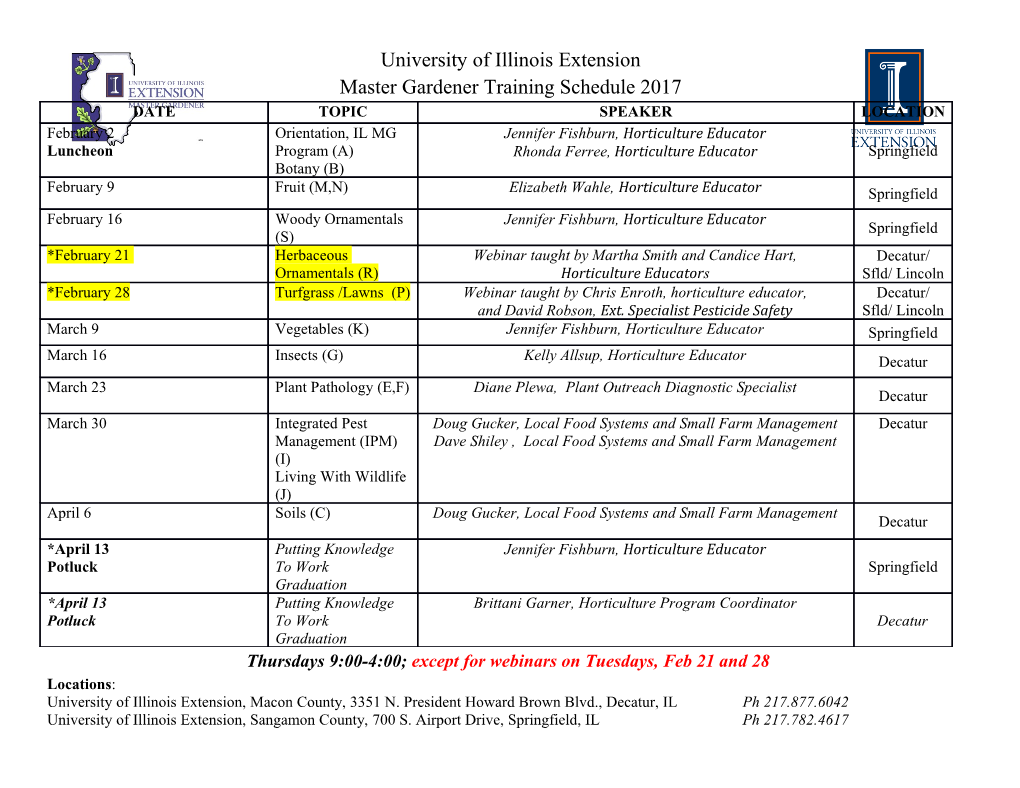
CUDACUDA FundamentalsFundamentals DavidDavid Luebke,Luebke, NVIDIANVIDIA ResearchResearch Beyond Programmable Shading: In Action TheThe ““NewNew”” MooreMoore’’ss LawLaw • Computers no longer get faster, just wider – Many people have not yet gotten this memo • You must re-think your algorithms to be parallel ! – Not just a good idea – the only way to gain performance – Otherwise: if its not fast enough now, it never will be • Data-parallel computing is most scalable solution – Otherwise: refactor code for 2 cores 4 cores 8 cores 16 cores… – You will always have more data than cores – build the computation around the data Beyond Programmable Shading: In Action EnterEnter thethe GPUGPU • Massive economies of scale • Massively parallel Beyond Programmable Shading: In Action EnterEnter CUDACUDA • CUDA is a scalable parallel programming model and a software environment for parallel computing – Minimal extensions to familiar C/C++ environment – Heterogeneous serial-parallel programming model • NVIDIA’s GPU architecture accelerates CUDA – Expose the computational horsepower of NVIDIA GPUs – Enable general-purpose GPU computing (CUDA also maps well to multicore CPUs…) Beyond Programmable Shading: In Action TheThe DemocratizationDemocratization ofof ParallelParallel ComputingComputing • GPU Computing with CUDA brings parallel computing to the masses – Over 85,000,000 CUDA-capable GPUs sold – A “developer kit” costs ~$100 (for 500 GFLOPS!) • Data-parallel supercomputers are everywhere – CUDA makes this power accessible – We’re already seeing innovations in data-parallel computing Massively parallel computing has become a commodity technology Beyond Programmable Shading: In Action CUDACUDA ProgrammingProgramming ModelModel Beyond Programmable Shading: In Action SomeSome DesignDesign GoalsGoals • Scale to 100’s of cores, 1000’s of parallel threads • Let programmers focus on parallel algorithms – not mechanics of a parallel programming language • Enable heterogeneous systems (i.e., CPU+GPU) – CPU good at serial computation, GPU at parallel Beyond Programmable Shading: In Action KeyKey ParallelParallel AbstractionsAbstractions inin CUDACUDA 0. Zillions of lightweight threads Î Simple decomposition model 1. Hierarchy of concurrent threads Î Simple execution model 2. Lightweight synchronization primitives Î Simple synchronization model 3. Shared memory model for cooperating threads Î Simple communication model Beyond Programmable Shading: In Action HierarchyHierarchy ofof concurrentconcurrent threadsthreads • Parallel kernels composed of many threads Thread t – all threads execute the same sequential program • Threads are grouped into thread blocks Block b – threads in the same block can cooperate t0 t1 … tB • Threads/blocks Kernel foo() have unique IDs . Beyond Programmable Shading: In Action HeterogeneousHeterogeneous ProgrammingProgramming • CUDA = serial program with parallel kernels, all in C – Serial C code executes in a CPU thread – Parallel kernel C code executes in thread blocks across multiple processing elements Serial Code Parallel Kernel . foo<<< nBlk, nTid >>>(args); Serial Code Parallel Kernel . bar<<< nBlk, nTid >>>(args); Beyond Programmable Shading: In Action Example:Example: VectorVector AdditionAddition KernelKernel // Compute vector sum C = A+B // Each thread performs one pair-wise addition __global__ void vecAdd(float* A, float* B, float* C) { int i = threadIdx.x + blockDim.x * blockIdx.x; C[i] = A[i] + B[i]; } Device Code int main() { // Run N/256 blocks of 256 threads each vecAdd<<< N/256, 256>>>(d_A, d_B, d_C); } Beyond Programmable Shading: In Action Example:Example: VectorVector AdditionAddition KernelKernel // Compute vector sum C = A+B // Each thread performs one pair-wise addition __global__ void vecAdd(float* A, float* B, float* C) { int i = threadIdx.x + blockDim.x * blockIdx.x; C[i] = A[i] + B[i]; } Host Code int main() { // Run N/256 blocks of 256 threads each vecAdd<<< N/256, 256>>>(d_A, d_B, d_C); } Beyond Programmable Shading: In Action SynchronizationSynchronization && CoordinationCoordination • Threads within block may synchronize with barriers … Step 1 … __syncthreads(); … Step 2 … • Blocks coordinate via atomic memory operations – e.g., increment shared queue pointer with atomicInc() • Implicit barrier between dependent kernels vec_minus<<<nblocks, blksize>>>(a, b, c); vec_dot<<<nblocks, blksize>>>(c, c); Beyond Programmable Shading: In Action ThreadsThreads && ThreadThread BlocksBlocks • What is a thread? –An independent thread of execution – Has its own PC, variables (registers), processor state, etc. • What is a thread block? –A virtualized multiprocessor – Fit processors to data & computation, each kernel launch –A (data) parallel task – all blocks in kernel have the same entry point – but may execute any code they want Beyond Programmable Shading: In Action BlocksBlocks MustMust BeBe IndependentIndependent • Any possible interleaving of blocks should be valid – presumed to run to completion without pre-emption – can run in any order – can run concurrently OR sequentially • Blocks may coordinate but not synchronize – shared queue pointer: OK – shared lock: BAD … can easily deadlock • Independence requirement gives scalability Beyond Programmable Shading: In Action BlocksBlocks RunRun onon MultiprocessorsMultiprocessors Kernel launched by host . Device processor array MT IU MT IU MT IU MT IU MT IU MT IU MT IU MT IU SP SP SP SP SP SP SP SP . Shared Shared Shared Shared Shared Shared Shared Shared Memory Memory Memory Memory Memory Memory Memory Memory Device Memory Beyond Programmable Shading: In Action LevelsLevels ofof ParallelismParallelism • Thread parallelism – each thread is an independent thread of execution • Data parallelism – across threads in a block – across blocks in a kernel • Task parallelism – different blocks are independent – independent kernels Beyond Programmable Shading: In Action MemoryMemory modelmodel Thread Block Per-block Per-thread Shared Local Memory Memory Beyond Programmable Shading: In Action MemoryMemory modelmodel Kernel 0 . Sequential Per-device Kernels Kernel 1 Global Memory . Beyond Programmable Shading: In Action MemoryMemory modelmodel Device 0 memory Host cudaMemcpy() memory Device 1 memory Beyond Programmable Shading: In Action CUDACUDA SDKSDK Libraries:FFT, BLAS,… Integrated CPU Example Source Code and GPU C Source Code NVIDIA C Compiler NVIDIA Assembly CPU Host Code for Computing CUDA Debugger Standard C Compiler Driver Profiler GPU CPU Beyond Programmable Shading: In Action GraphicsGraphics InteroperabilityInteroperability • Go “Beyond Programmable Shading” • Access textures from within CUDA – Free filtering, wrapping, 2/3D addressing, spatial caching... • Share resources (vertex, pixel, texture, surface) – Map resources into CUDA global memory, manipulate freely –Geometry: physics (collision, response, deformation, ...), lighting, geometry creation, non-traditional rasterization, acceleration structures, AI, ... –Imaging: image processing, (de)compression, procedural texture generation, postprocess effects, computer vision, ... Beyond Programmable Shading: In Action SummarySummary • CUDA is a powerful parallel programming model – Heterogeneous - mixed serial-parallel programming –Scalable- hierarchical thread execution model – Accessible - minimal but expressive changes to C – Interoperable - simple graphics interop mechanisms • CUDA is an attractive platform – Broad - OpenGL, DirectX, WinXP, Vista, Linux, MacOS – Widespread - over 85M CUDA GPUs, 60K CUDA developers CUDA provides tremendous scope for innovative graphics research “beyond programmable shading” Beyond Programmable Shading: In Action Questions?Questions? DavidDavid LuebkeLuebke [email protected]@nvidia.com Beyond Programmable Shading: In Action CUDACUDA DesignDesign ChoicesChoices Beyond Programmable Shading: In Action Goal:Goal: ScalabilityScalability • Scalable execution – Program must be insensitive to the number of cores – Write one program for any number of SM cores – Program runs on any size GPU without recompiling • Hierarchical execution model – Decompose problem into sequential steps (kernels) – Decompose kernel into computing parallel blocks – Decompose block into computing parallel threads • Hardware distributes independent blocks to SMs as available Beyond Programmable Shading: In Action BlocksBlocks RunRun onon MultiprocessorsMultiprocessors Kernel launched by host . Device processor array MT IU MT IU MT IU MT IU MT IU MT IU MT IU MT IU SP SP SP SP SP SP SP SP . Shared Shared Shared Shared Shared Shared Shared Shared Memory Memory Memory Memory Memory Memory Memory Memory Device Memory Beyond Programmable Shading: In Action Goal:Goal: easyeasy toto programprogram • Strategies: – Familiar programming language mechanics • C/C++ with small extensions – Simple parallel abstractions • Simple barrier synchronization • Shared memory semantics • Hardware-managed hierarchy of threads Beyond Programmable Shading: In Action HardwareHardware MultithreadingMultithreading • Hardware allocates resources to blocks – blocks need: thread slots, registers, shared memory – blocks don’t run until resources are available • Hardware schedules threads – threads have their own registers – any thread not waiting for something can run – context switching is (basically) free – every cycle • Hardware relies on threads to hide latency – i.e., parallelism is necessary for performance Beyond Programmable Shading: In Action GraphicsGraphics InteropInterop DetailsDetails Beyond Programmable Shading:
Details
-
File Typepdf
-
Upload Time-
-
Content LanguagesEnglish
-
Upload UserAnonymous/Not logged-in
-
File Pages85 Page
-
File Size-