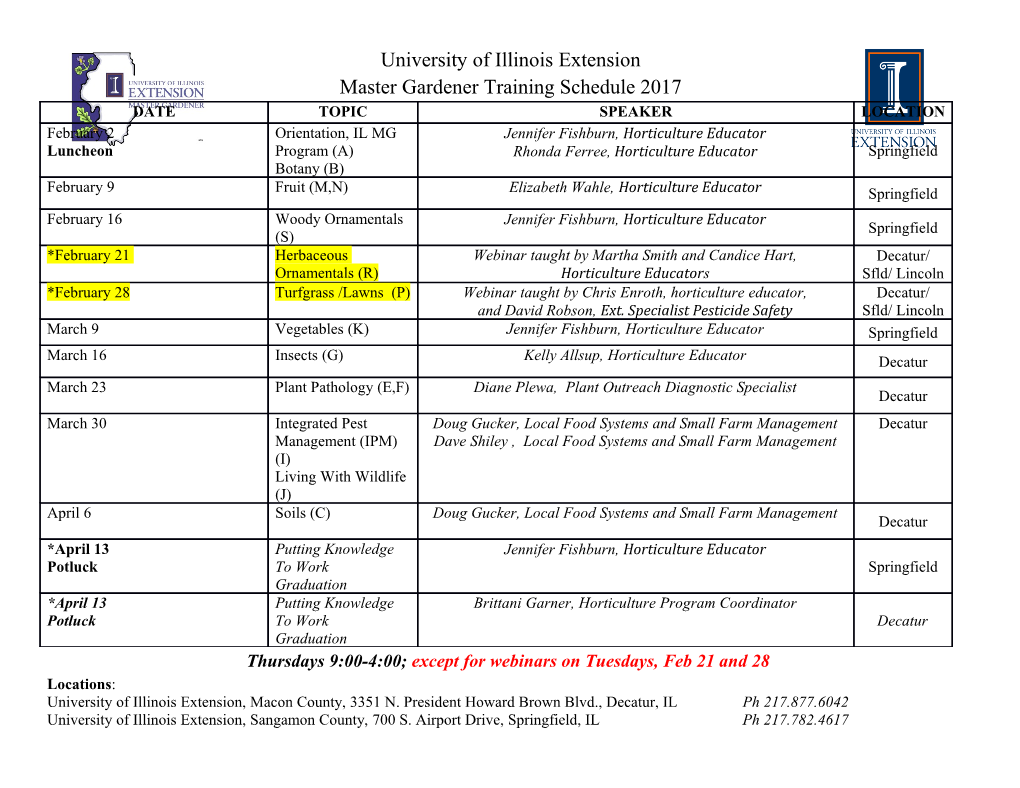
Stereo Matching with Color and Monochrome Cameras in Low-light Conditions Hae-Gon Jeon1 Joon-Young Lee2 Sunghoon Im1 Hyowon Ha1 In So Kweon1 [email protected] [email protected] [email protected] [email protected] [email protected] 1 Robotics and Computer Vision Lab., KAIST 2 Adobe Research Abstract Consumer devices with stereo cameras have become popular because of their low-cost depth sensing capability. However, those systems usually suffer from low imaging quality and inaccurate depth acquisition under low-light (a) A pair of color and monochrome images conditions. To address the problem, we present a new stereo matching method with a color and monochrome camera pair. We focus on the fundamental trade-off that monochrome cameras have much better light-efficiency than color-filtered cameras. Our key ideas involve com- pensating for the radiometric difference between two cross- spectral images and taking full advantage of complemen- (b) Estimated disparity map (c) Recovered color image tary data. Consequently, our method produces both an accurate depth map and high-quality images, which are applicable for various depth-aware image processing. Our method is evaluated using various datasets and the per- formance of our depth estimation consistently outperforms state-of-the-art methods. (d) Image refocusing (e) Image stylization Figure 1. Given the pair of images (a), we estimate the accurate disparity map (b) and recover the high-quality color image (c). Our 1. Introduction result is applicable to various depth-aware image processing (d, e). Stereo camera systems allow us to estimate depth infor- mation and have many advantages over active range sensors these approaches require high manufacturing cost and such as ToF (time-of-flight) cameras and laser scanners. specialized hardware. Stereo cameras are cost-effective and can work in both In this paper, we present a stereo matching framework indoor and outdoor environments. This is why they have with a color and monochrome image pair (Fig. 1(a)). Our been widely used in the computer vision and robotics fields system is designed to estimate an accurate depth map for several decades. Recently, consumer devices having a under low-light conditions without additional light sources stereo camera [2, 4] have been released for depth-aware (Fig. 1(b)). In order to obtain reliable correspondence, image editing applications. we exploit the fundamental trade-off between color sens- Despite the advantages, estimating accurate depth map ing capability and light efficiency of color cameras and in low-light conditions leads to severe image noise; thus is monochrome cameras, respectively. Because monochrome still challenging and limits the usefulness of stereo systems. cameras respond to all colors of light, they have much better Although a long exposure time or a flash light may alleviate light efficiency than Bayer-filtered color cameras [7, 3]. the problem, they can induce other imaging problems such In general, image luminance recorded from a color as motion blur or specular reflections [19]. To overcome camera is not consistent with that from a monochrome these issues, multi-modal and multi-spectral imaging ap- camera due to spatially-varying illumination and different proaches such as a color and infrared camera pair [16] and spectral sensitivities of the cameras. This degrades the cross-channel matching [26] have been proposed. However, performance of stereo matching. To solve this problem, 14086 Imaging Performance we sample appropriate decolorization parameters of a color 70 Blue image and perform locally adaptive radiometric alignment 60 Green Red Gray and correspondences augmentation iteratively. After esti- 50 mating a depth map, we recover a high-quality color image 40 by colorizing the image from a light-efficient monochrome camera. For accurate colorization, we introduce a local 30 20 chrominance consistency measure. Herein, we demonstrate QuantumEfficiency (%) the superior stereo matching performance over state-of-the- 10 0 art methods. In addition, we show our result can be applied 300 400 500 600 700 800 900 1000 to depth-aware image processing algorithms such as image Wavelength (nm) stylization and digital refocusing (Fig. 1(c)). (a) (b) Figure 2. (a) Spectral sensitivity of the color and monochrome camera [1] used in our prototype stereo system. (b) An example 2. Related Work image pair captured by our stereo system. Note that there is Our method is related to cross-spectral stereo matching visible difference of image noise due to the gap of light-efficiency and colorization. Prior to introducing previous studies, we between two cameras. refer the reader to [14] for a comprehensive discussion of equalization. As will be demonstrated in the experimental stereo matching with radiometric and noise variation. section (Sec. 7), our method is highly effective for accurate Cross-spectral stereo matching has been studied exten- disparity estimation and significantly outperforms the state- sively to find correspondence between multi-modal and of-the-art algorithms [12, 13, 18, 15]. In colorization, most color-inconsistent stereo images. Heo et al.[12] analyzed approaches concentrate on propagating limited numbers of a color formation model and proposed an adaptive nor- user-defined seeds, while we have lots of seed pixels with malized cross correlation for stereo matching, that would outliers around occlusion boundaries. To handle this issue, be robust to various radiometric changes. This was we introduced a new weighting term to correct inaccurate extended in [13], which presented an iterative framework seed pixels and successfully recover a high-quality color to simultaneously achieve both depth estimation and color image. consistency. Pinggera et al.[23] presented depth map Recent work by [7] presented the concept of an alter- estimation with cross-spectral stereo images, which uses native camera sensor that samples color information very dense gradient features based on the HOG descriptor [9]. sparsely. They recover a full color image by propagating Kim et al.[18] designed a dense descriptor for multi- the sparsely sampled colors into an entire image. This modal correspondences by leveraging a measure of adaptive work shares the same philosophy with our work that takes self-correlation and randomized receptive field pooling. the advantage of light-efficient monochrome sensors, but Holloway et al.[15] proposed an assorted camera array the concept may suffer from color noise that leads to an and a cross-channel point correspondence measure using erroneous color image. Moreover, we adopt the idea in a normalized gradient cost. stereo system and obtain an accurate depth-map and a noise- Colorization is a process of adding color channels to a free color image simultaneously. grayscale image and video. Levin et al.[21] presented a user-guided colorization method that takes partial color 3. Stereo System with Color and Mono Cameras information from user scribbles and automatically propa- gates the given seed color to make a complete color image. Most color cameras use a color filter array called a Yatziv and Sapiro [30] proposed a fast colorization method Bayer array to capture color information. The Bayer using the geodesic distance between neighboring pixels. array is positioned over the pixels of an image sensor Gastal and Oliveira [10] introduced an edge-aware filter in a and separates the incoming light into one of three primary transformed domain and showed a colorization result as one colors (red, green, or blue) by filtering the light spectra of its applications. Irony et al.[17] proposed an example- according to wavelength range. This process is effective based colorization based on an assumption that similarly for capturing color information, but it amplifies image noise textured regions have similar colors. under low-light conditions because the array occludes a lot In this study, we focused on simultaneously recon- of incoming light. It may also reduce image sharpness as a structing an accurate depth-map and a noise-free color result of using an anti-aliasing filter or optical low-pass filter image using a color + monochrome image pair. We to avoid aliasing or moire´ artifacts during the demosaicing achieve this by taking the advantage of our cross-spectral process. stereo system. We created a locally adaptive spectral Unlike color cameras, monochrome cameras receive all alignment algorithm that allows us to estimate an accurate the incoming light at each pixel and need no demosaicing disparity map without complex optimizations for intensity process. Therefore, they have much better light efficiency 4087 (b) Input pair (c) Gain map (a) Baseline (e) Disparity map by (f) Refined map by (g) High-quality disparity map iterative gain a tree-based filtering color image (d) Decolorization adjustment Figure 3. The overview of our algorithm. Our method produces the accurate depth map (f) and the high-quality color image (g), while the baseline stereo matching method with the luminance channels of the input images results in the poor disparity map (a). and provide shaper images. In Fig. 2, we compare the difference or image noise, we retain reliable correspon- imaging quality of a color and a monochrome camera. dences with a left-right consistency check and aggregate The comparison of spectral sensitivity (Fig. 2(a)) and the them from all candidate decolorized images. example image-pair captured under the same conditions After that, we have a set of reliable correspondences (Fig. 2(b)) prove the large difference in light efficiency and and use them to augment additional correspondences by image quality between the two types of cameras. That is, iterative gain compensation and disparity estimation. Given a color + monochrome camera pair is highly suitable for the grayscale input and aligned decolorized image, we achieving a noise-free color image in addition to accurate match the brightness of the input image to the decolorized depth estimation. image by estimating a local gain map (Sec. 5.3).
Details
-
File Typepdf
-
Upload Time-
-
Content LanguagesEnglish
-
Upload UserAnonymous/Not logged-in
-
File Pages9 Page
-
File Size-