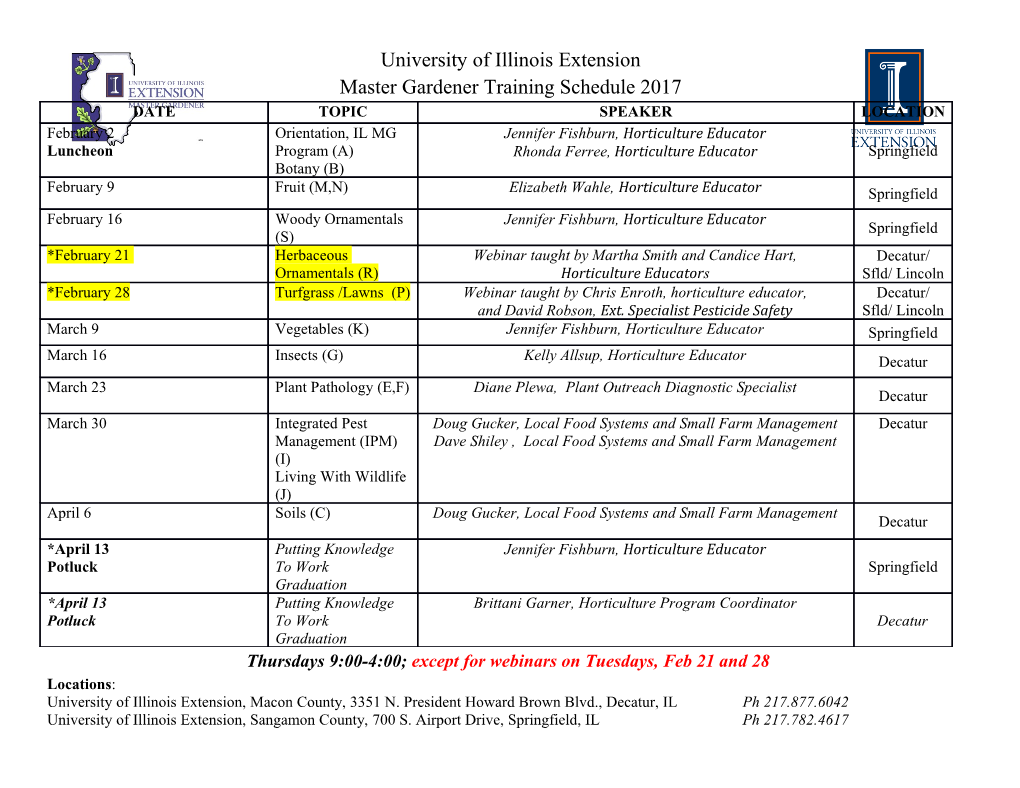
TEMPORAL MODELS IN DATA MINING: ENRICHMENT, SUMMARIZATION AND RECOMMENDATION Von der Fakultät für Elektrotechnik und Informatik der Gottfried Wilhelm Leibniz Universität Hannover zur Erlangung des Grades DOKTOR DER NATURWISSENSCHAFTEN Dr. rer. nat. genehmigte Dissertation von M.Sc. Anh Tuan Tran geboren am 24. März 1985, in Hanoi, Vietnam 2018 Referent: Prof. Dr. techn. Wolfgang Nejdl Korreferent: Prof. Dr. Vinay Setty Korreferent: Prof. Dr. Heribert Vollmer Tag der Promotion: 07. August 2018 ABSTRACT Time plays a major and multifaceted role when studying digital collections and their relationship with the user. This is especially true when digital contents are digested long after their time point of generation, creating rooms where numerous events can be observed: The contents are revised or overwritten, users are exposed to other contents in the collection with related information and thereby update their interpretation and interest. Furthermore, the evolution of context and new events beyond the collection can also influence user perception of relevance or values of the contents. Therefore, good information processing and retrieval systems should take into account these effects of time, not only within a single object but also from intra- as well as inter-collections. At this collective level, it is important to address the user cognitive behaviour in processing information, as humans have the ability of connecting different pieces of information from various sources, and they often exercise this power, consciously or unconsciously, when generating and consuming digital contents. Despite two decades of active research in temporal data mining and information re- trieval, little attention has been paid to the effects of the cognitive aspects on time-aware information access at the collection level. This includes aspects such as how users perceive and memorise long-running events reported in online news, or how human forgetfulness affects their behaviour in finding their own digital material, etc. In this thesis, we investigate several research questions in temporal data mining in the new perspective, inspired from the human cognitive processes in creating, organizing, ex- changing, and seeking temporal information. In particular, we address the tasks of: (1) identifying temporal topics from texts and enriching them with semantic annotations; (2) summarizing text data using timeline, as well as via cognitive-based models; (3) helping users in finding their own documents through studying the influence of time on their mem- ory at work. In more detail, we introduce a novel method to annotate topics for textual data that leverages social and temporal signals, and demonstrate its effectiveness for trending topics in social media posts such as in Twitter. We also address the scalability issue, both in algorithmic perspective and computational architecture perspective. Furthermore, we intro- duce the concept of entity-centric timeline summarization for long-running events in news collection, again exploiting social and temporal signals from encyclopedic resources such as Wikipedia together with features from text. For this purpose, we propose a novel adap- tive learning algorithms that leverage both the relevance and novelty of news articles. As another contribution in content summarization from a more cognitive perspective, we study the domain of summarizing dialogues for decisions, taking into account the foundations in human decision making theory. Finally, we make contributions in studying human memories in enterprise domain, and design a new graph learning method to recommend professional contents for a temporal task at hand. Keywords: information extraction, temporal data analysis, semantic data, cognitive models, summarization, recommender system, learning to rank, structured learning ZUSAMMENFASSUNG Die Zeit spielt eine wichtige und vielseitige Rolle dabei, die digitalen Sammlungen unter Berücksichtigung der Beziehung zu den Nutzern zu studieren. Dies gilt inbesondere, wenn digitale Inhalte lange nach ihrem Erstellungszeitpunkt verarbeitet werden und die Zeitraum, in dem zahlreiche Ereignisse beobachtet werden können, erzeugt wird. Zum Beispiel: Die Dokumente werden geändert oder überarbeitet; Benutzer werden anderen verbundenen In- formation in der Sammlung ausgesetzt und damit ihr Wissen, ihre Interpretation und Ihre Interesse aktualisieren. Ein weiteres Beispiel können die Veränderung des Kontexts und die Entstehung neuer Ereignisse die Wahrnehmung der Nutzer von Relevanz oder Werten der Inhalte beeinflussen. Daher sollte hochwertige Informationsverarbeitungs -und- aufruf- systeme diese Auswirkungen der Zeit berücksichtigen, nicht nur innerhalb eines einzelnen Objekt, sondern auch von Intra- sowie Intersammlungen. Auf dieser kollektiven Ebene ist es wichtig, das kognitive Verhalten des Nutzers bei der Verarbeitung von Informationen einzugehen. Der Grund dafür ist, dass Menschen die Fähigkeit haben, Informationen aus verschiedenen Quellen zu verbinden, und dass sie diese Befugnis oft bewußt oder bewußtlos ausüben, wenn sie digitale Inhalte erstellen oder erfassen. Trotz jahrzehntelanger Forschung in zeitlichem Data Mining und Information Retrieval wurde bislang den Auswirkungen der kognitiven Aspekte auf den zeitabhängigen Informa- tionsverarbeitung auf der Sammlungsebene nur wenig Aufmerksamkeit geschenkt. Dazu gehören Aspekte wie die Art und Weise, wie Nutzer Dauerereignisse z.B. in Online- Nachrichten verfolgen oder auswendig lernen, oder wie sich menschliche Vergesslichkeit auf ihr Verhalten bei der Suche nach ihrem eigenen digitalen Material auswirkt. In dieser Dissertation legen wir verschiedene Forschungsfragen im zeitlichen Data Min- ing aus einer neuen Perspektive fest, inspiriert von den menschlichen kognitiven Prozessen bei den Erstellungen, Organisierungen, Austauschen und Suchen nach zeitlichen Informa- tionen. Insbesondere richten wir auf folgende Fragestellung aus: (1) Wie die zeitlichen Themen von Textinhalte ermittelt werden, und damit wie die Inhalte um semantische Infor- mation angereichert werden; (2) Wie die Textdaten anhand der Zeitleiste sowie von kognitiv basierten Modellen richtig zusammengefasst werden; (3) Wie ein System die Nutzer bei der Such nach ihrem eigenen Dokumente unterstützen kann, indem die Auswirkung der Zeit auf ihre Gedächtnis berücksichtigt wird. Zur ersten Frage führen wir eine neue Methode für Anreicherung der zeitlichen Themen von Textdaten ein, die soziale und zeitliche Merkmale verwendet, und zeigen ihre Wirksamkeit, um Social-Media-Trending z.B. in Twitter anzure- ichern. Außerdem befassen wir uns mit dem Thema Skalierbarkeit, sowohl in Bezug auf die algorithmischen Modellen als auch auf die Infrastruktur. Zur zweiten Frage setzen wir das neue Konzept der Entity-basierten Zeitleiste als Zusammenfassung der Dauerereignisse ein, und entwickeln wir neue Methodenansätzen zur effektiven Zusammenfassen von Online- Nachrichtenartikeln. Unsere Methode kombiniert soziale und zeitliche Merkmalen, die aus Enzyklopädischen wie Wikipedia entstehen, mit herkömmliche Merkmalen der Tex- tinhalte. Die Methode kann auch anhand eines neuartigen adaptiven Lernalgorithmus die Relevanz und die Neuheit von Nachrichtenartikeln ausgleichen. Neben Online-Nachrichten untersuchen wir weiteren Bereich des Zusammenfassens von gesprochenen Dialogen unter Berücksichtigung der menschlichen Entscheidungsverfahren. Zur dritten Frage leisten wir Beiträge zur Erforschung menschlicher Erinnerungen im Unternehmensbereich und entwer- fen eine neue Graph-Lernmethode, um professionelle Inhalte für eine zeitliche Aufgabe zu empfehlen. Schlagwörter: Informationsextraktion, Zeitliche Datenanalyse, semantischen Daten, Kognitives Modell, Textzusammenfassung, Empfehlungsdienst, Lernen auf Rang, strukturi- ertes Lernen ACKNOWLEDGMENTS In the course of this thesis, I am grateful to many people for their support and assistance. First of all, I would like to acknowledge my advisor Prof. Dr. Wolfgang Nejdl, for giving me the opportunity to work in L3S Research Center together with the many excellent scientists in the area of Web Science, and on many interesting projects. I also thank associate Prof. Dr. Vinay Setty, for agreeing to consider and evaluate my PhD thesis. In addition, I would like to thank Ms Anca Vais and Ms Marion Walters for their organizational efforts during the beginning and last phases of my PhD study. I truly appreciate all of their time and work. A special thank to Dr. Claudia Niederée, who has been both my project man- ager and my research mentor since the very first day of my PhD. Dr. Niederée has given me invaluable guidance during my time at L3S, both professional and per- sonal, which has shaped my skills in conducting research, managing time, writing scientific and business works, etc. In this thesis, I would like to give her heartfelt thanks for all of her support. Additionally, I would like to express my gratitude to my colleagues and friends at L3S for many fruitful discussions, as well as for great experiences that we share in this house. It has been amazing six years with a lot of fun and lessons, without which I would not have been able to accomplish many of the achievements in this thesis. I would like to extend my sincerest thanks to my parents, my sisters Lan Anh and Hai Anh for their encouragement and continuous support during my time in Germany. Finally, I am indebted to my wife Nhung and my daughter Vy, for their unconditional love, and for being the source of my motivation and power every
Details
-
File Typepdf
-
Upload Time-
-
Content LanguagesEnglish
-
Upload UserAnonymous/Not logged-in
-
File Pages144 Page
-
File Size-