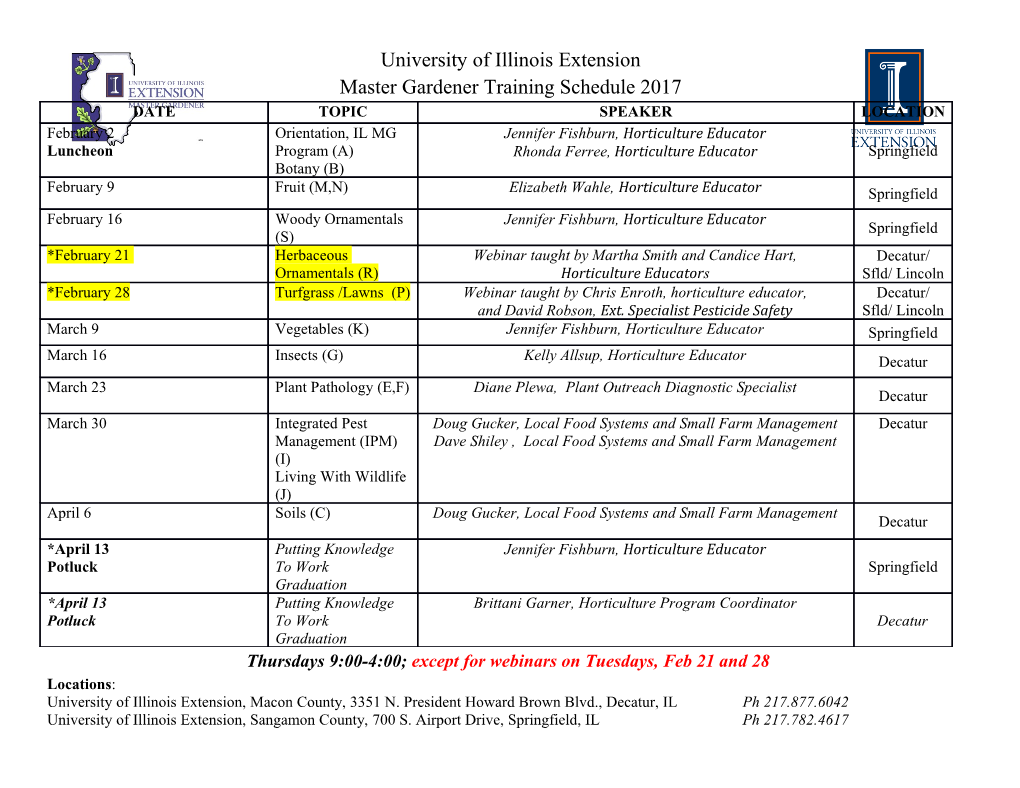
AI Ignition Ignite your AI curiosity with Greg Brockman How do we take responsibility for the varied uses of AI? From Deloitte’s AI Institute, this is AI Ignition—a monthly chat about the human side of artificial intelligence with your host, Beena Ammanath. We will take a deep dive into the past, present, and future of AI, machine learning, neural networks, and other cutting-edge technologies. Here is your host, Beena. Beena Ammanath (Beena): Hi, my name is Beena Ammanath and I am the executive director for the Deloitte AI Institute, and today on AI Ignition we have Greg Brockman, the co-founder and CTO of Open AI, a research organization that aims to ensure that artificial general intelligence benefits all of humanity. Welcome to the show, Greg. We are so excited to have you. Greg, I am so happy to have you on AI Ignition today. Thank you so much for joining us. Greg: Thank you for having me. Excited to be here. Beena: So, let’s start with the current status of AI. AI is such a broad topic, and you hear so much in the news about it, everybody knows what you are doing at Open AI. What do you think is the current status of AI today? Greg: So, I think AI is definitely a field in transition. I mean, if you look at the history of the field, I think it has gotten a reputation as the field of empty promises. And you look back to the 50s and people were trying to figure out, hey maybe the hardest problem is chess, and if we can solve chess, then we are going to solve all of AI and we’ll have an artificial human and it will be great. In the 90s, chess came and went, and it didn’t really feel like our lives changed at all. I think that 2012 was really the moment that things started to transition with the creation of AlexNet, which is the first sort of convolutional network on GPU that was actually able to just meaningfully outperform everything else. It’s the first time that like all of these, you are a human, you think hard about a domain, you write down all the rules, all those systems just were paled in comparison to what you could do by just having a neural net or just kind of an architecture that can absorb whatever data you show it, and suddenly that’s the best way of doing things. In image recognition, and everyone kind of looked at it and said, “Okay, that’s a cool trick, but hey it’s never going to work for language. It’s never going to work for machine translation.” And it kind of has. Basically, the whole past decade has been, okay we are starting to see that for all of these areas that feel like machines have never really been able to touch them, things like language, things like understanding what’s in a video. We are starting to be able to crack it, and the way we crack it is it’s all with the same piece of technology, it’s just a neural net. So, that’s all the background, that’s where we have been. And I think GPT-3 is also a moment that shows a sea change in terms of what the tech can do. This is the first time where you take one of these big neural nets. It’s basically just an architecture you dump all this data into, and suddenly it’s actually economically useful. Like people actually want to use our API to just talk to our model. Some people do it for fun, but actually many, many people are building businesses on top of it. You have various applications ranging from assistants that help you write better copy, whether it’s A/B testing and we actually have had a number of users do pretty rigorous tests with GPT-3 versus human copywriters and found that actually GPT-3 does pretty darn well. You have people who use it for games, things like AI Dungeon where you actually have an infinite dungeon like a DND style game that is generated by this model, and there is a huge range of other applications, such as people who are trying to help tenants who receive eviction notices or other legal proceedings in very complex language, trying to summarize it and point out the important points for people who don’t have access to their own legal counsel. I think there is this huge variety of different things that people can do once you have a machine that can start to actually interact, meet humans in the language that we all operate in. So, I think that it’s this exciting moment where you can actually train one of these networks and they are actually economically useful. Beena: Yeah, so true, and I am going to date myself here because I studied AI back in the late 80s, early 90s and that time it was mostly theory. We didn’t have access to massive amounts of data or the compute power that was needed. So, it was mostly what was written in the books and ideas around it. I am not kidding but back then even personalized marketing, which is so prevalent now, was considered to be too complex for an AI or any kind of software to do. Greg: But I also think that all of that work laid a really good foundation. There is always this joke that everything was invented by Schmidhuber in the 90s and it’s not entirely false. That there are a couple of ideas that are out there now that are kind of totally new and unique, and partly because we saw like when you actually build things in this certain way and we have this particular hardware architecture for GPU that maybe doing massive parallelism in this particular way is useful, and that is where a transformer comes from. But most of it I think is actually, we basically have 70 years’ worth of people thinking super hard about the same neural net piece of technology we’re using, and so we can just build on the shoulder of giants. Beena: That is so fascinating because I think in a way we were unencumbered by restrictions. So, it was just ideas that we’re spouting on, what if this was real, what are all the possibilities. That’s a great point. I never thought of it that way. Greg: The thing that I find most interesting about this field is that it doesn’t comport with the way that we think science should go. And you can really look back to the history of these debates between the connectionists, the people who basically said, “Hey, these neural nets which are kind of this like if you squinted at a model of the information processing of the brain,” and then the people who are the symbolic systems people, who have said, “We humans are really smart, we know how systems work, let’s just write down all the rules and try to like really engineer a system that operates like we do.” And there were these great debates of which one was going to win, and it really looked in the 60s like these connections people were a bunch of hacks. There was really this whole campaign waged with books like the Perceptron and things like that to discredit all of them and it really worked. And somehow the connectionist school of thought has just been this steady rising tide. We see that there is this smooth continuous curve of increasing progress that has lasted since 2012, but we actually did a study too where we extended it backwards and we found that actually there has been a smooth exponential in terms of the increased performance of these networks over decades now, and I think that points to something really real that’s going on, and it’s just so fascinating. Beena: Yes. Now you are looking at core AI. How do you see AI creating new opportunities across industries? Greg: I think that this is really the most important question. We think about AI, I actually think AI has a pretty bad brand. Like if you ask someone on the street what they think of AI, you are probably going to get some reference to a Hollywood movie or something like worrying about how it will impact jobs or people’s lives, and I think all of that is fair. I think it is actually really important to confront the downsides, but I think that the reason we work on it, the reason we are so excited to build it, the reason that we have a whole—that Open AI as a company dedicated to trying to build advanced AI is because we see the promise that it can bring. I think that the real goal is to be able to build systems that can help us solve problems that are otherwise out of reach. You think about healthcare. So, I have a very close friend who has a very rare medical condition and every time he talks to a new specialist, they always bounce him to another specialist and it’s a real problem. He is often bounced back to the same person and they are like, “Oh well, there is this thing that they didn’t consider.” Imagine if you just had one doctor who just understood the whole extent of human medicine.
Details
-
File Typepdf
-
Upload Time-
-
Content LanguagesEnglish
-
Upload UserAnonymous/Not logged-in
-
File Pages10 Page
-
File Size-