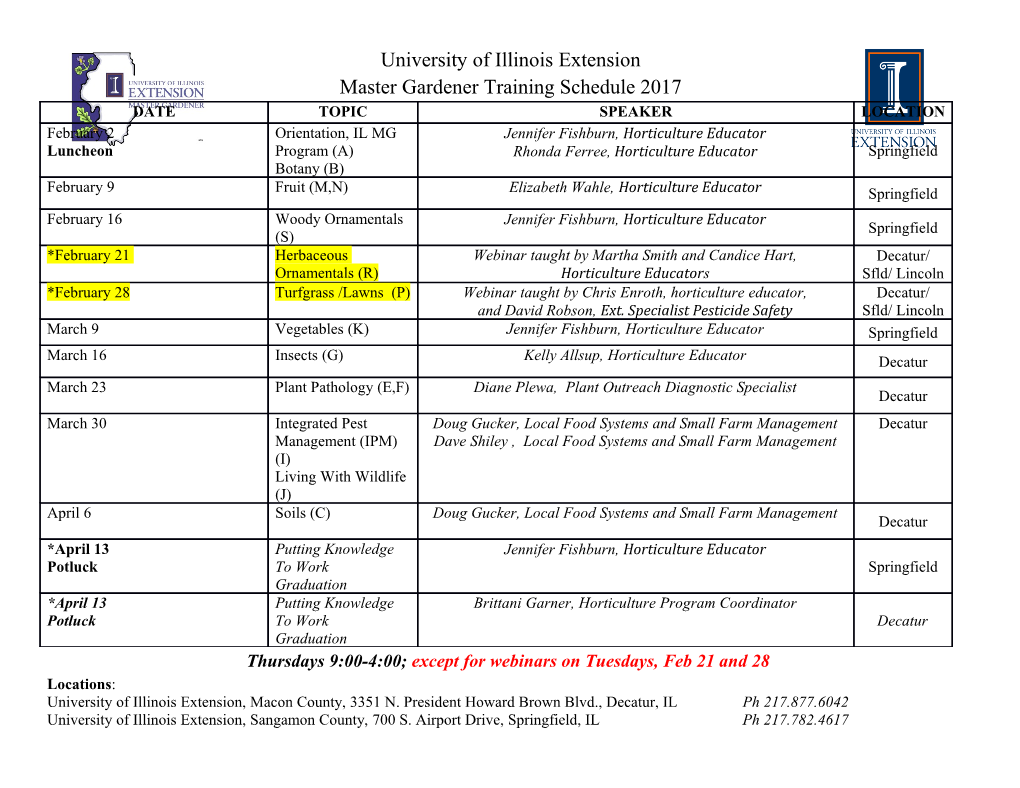
S EMINAR AND COLLOQUIUM ANNOUNCEMENTS November 4th – 8th 2019 Monday, 4th MATH BIOLOGY SEMINAR TITLE: Introduction to Complexity Theory SPEAKER: Louis Gross TIME: 10:10 AM ROOM: Claxton 105 ALGEBRA SEMINAR TITLE: Clifford Algebras SPEAKER: Betsy Downs TIME: 3:35 PM ROOM: Ayres 114 Tuesday, 5th TOPOLOGY/ GEOMETRY SEMINAR TITLE: Inductive Dimension of Coarse Proximity Spaces SPEAKER: Jeremy Siegert, UTK TIME: 11:10AM ROOM: Ayres 114 Abstract: In this talk, we define the asymptotic inductive dimension, $asInd$, of coarse proximity spaces. In the case of metric spaces equipped with their metric coarse proximity structure, this definition is equivalent to the definition of $asInd$ given by Dranishnikov for proper metric spaces. We show that if the boundary of a coarse proximity space is completely traceable, then the asymptotic inductive dimension of the space is equal to the large inductive dimension of its boundary. Consequently the large inductive dimension of well known boundaries such as the Gromov boundary and the visual boundary of Cat($0$) spaces is characterized. We also provide conditions on the space under which the boundary is completely traceable. Finally, we use neighborhood filters to define an inductive dimension of coarse proximity spaces whose value agrees with the Brouwer dimension of the boundary. S EMINAR AND COLLOQUIUM ANNOUNCEMENTS STOCHASTICS/PROBABILITY SEMINAR TITLE: Stochastic Dini's theorem with applications (Part 2) SPEAKER: Jan Rosinski, UTK TIME: 2:10 PM-3:25 PM ROOM: Ayres 112 Abstract: A stochastic version of Dini's theorem was found by Ito and Nisio. It provides a powerful tool to deduce the uniform convergence of stochastic processes from their pointwise convergence. Unfortunately, this tool fails in stronger than uniform modes of convergence, such as Lipschitz or phi-variation convergence, the latter mode being natural for processes processes with jumps. In this work we establish a stochastic version of Dini's theorem in a new framework that covers processes with jumps and strong modes of convergence. We apply these results to Levy driven stochastic differential equations Wednesday, 6th Oral Specialty Examination Speaker: Jesse Sautel, UTK Title: Oral Specialty Exam Room and Time: A 308H, 2:30 pm His committee consist of Professors Richter (Chair), Nicoara, and Sundberg. Householder Lecture Title: Speaker: Chun Liu Time: 3:30pm Room: Ayres 405 Abstract: S EMINAR AND COLLOQUIUM ANNOUNCEMENTS Colloquium Title: Robust Statistical Procedures for Noisy, High-dimensional Data Speaker: Anna Little Time: 4:45pm ROOM: Ayres 405 Abstract: This talk addresses two topics related to robust statistical procedures for analyzing noisy, high- dimensional data: (I) path-based spectral clustering and (II) robust multi-reference alignment. Both methods must overcome a large ambient dimension and lots of noise to extract the relevant low dimensional data structure in a computationally efficient way. In (I), the goal is to partition the data into meaningful groups, and this is achieved by a novel approach which combines a data driven metric with graph-based clustering. Using a data driven metric allows for strong theoretical guarantees and fast algorithms when clusters concentrate around low-dimensional sets. In (II), the goal is to recover a hidden signal from many noisy observations of the hidden signal, where each noisy observation includes a random translation, a random dilation, and high additive noise. A wavelet based approach is used to apply a data-driven, nonlinear unbiasing procedure, so that the estimate of the hidden signal is robust to high frequency perturbations. Thursday, 7th MATHEMATICAL DATA SCIENCE SEMINAR TITLE: Challenges in Bayesian inference via Markov chain Monte Carlo for neural networks SPEAKER: Papamarkou, Theodore TIME: 2:10 PM ROOM: Ayres 111 Abstract: Markov chain Monte Carlo (MCMC) methods and neural networks are instrumental in tackling inferential and prediction problems. However, Bayesian inference based on joint use of MCMC methods and of neural networks is limited. This talk reviews the main challenges posed by neural networks to MCMC developments, including lack of parameter identifiability due to weight symmetries, prior specification effects, and consequently high computational cost and convergence failure. Population and manifold MCMC algorithms are combined to demonstrate these challenges via multilayer perceptron (MLP) examples and to develop case studies for assessing the capacity of approximate inference methods to uncover the posterior covariance of neural network parameters. Some of these challenges, such as high computational cost arising from the application of neural networks to big data and parameter identifiability arising from weight symmetries, stimulate research towards more scalable approximate MCMC methods or towards MCMC methods in reduced parameter spaces. S EMINAR AND COLLOQUIUM ANNOUNCEMENTS Colloquium Friday, 8th TITLE: Mathematical modeling of cellular organization: regulatory signaling, cell mechanics, and collective cell behavior SPEAKER: Cole Zmurchok TIME: 3:35pm ROOM: Ayres 405 Abstract: Cell shape and polarity is regulated by a complex network of cytoskeletal signaling regulators (Rho-family GTPases) and mechanical feedback from the cell itself. I use mathematical modeling, computational approaches, and analysis (PDEs, ODEs, and agent-based models) to probe the cellular behaviors that result from these complex interactions. In this talk, I will discuss (1) how Rho GTPase dynamics can give rise to a diverse set of signaling profiles that match experimentally observed morphologies, (2) how the feedback between mechanics and signaling provides a physical mechanism for cells to adapt to high concentration signaling environments, and (3) how these feedbacks can generate collective cell dynamics seen in tissues. Together, these results help unravel the complex interactions between Rho GTPase signaling and mechanics that organize cell behavior. .
Details
-
File Typepdf
-
Upload Time-
-
Content LanguagesEnglish
-
Upload UserAnonymous/Not logged-in
-
File Pages4 Page
-
File Size-