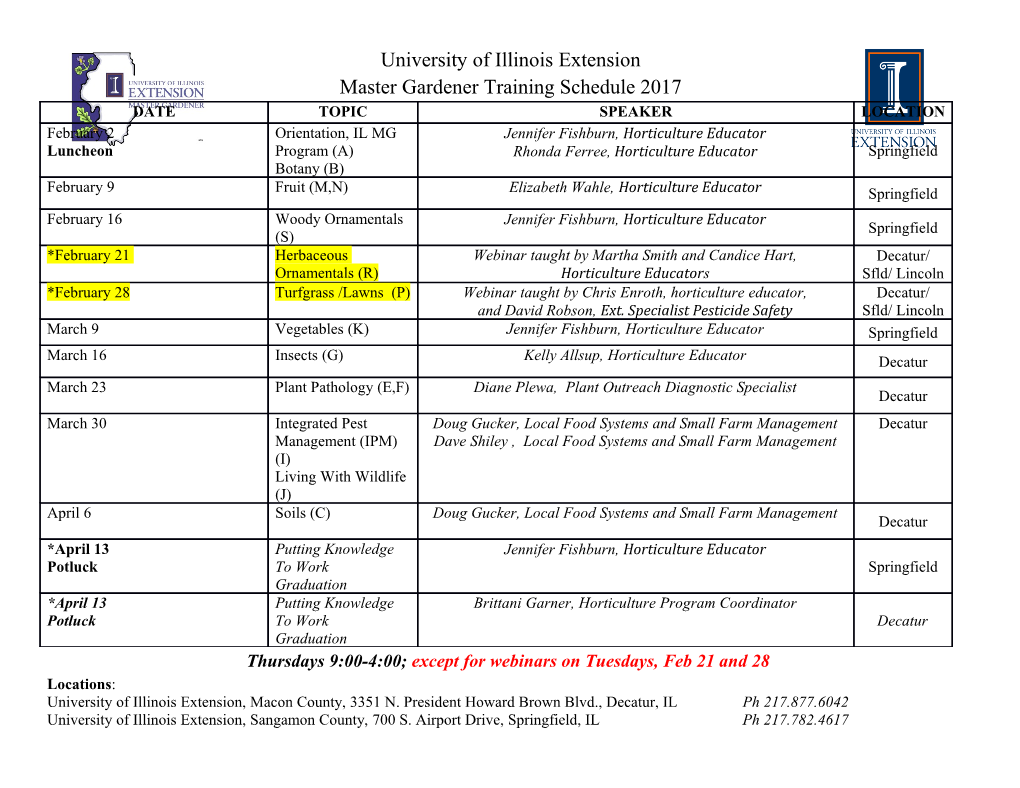
Fast 3D Optical Mammography using ICG Dynamics for Reader-Independent Lesion Differentiation Sophie Piper 1 P.Schneider 1, N. Volkwein 1, N.Schreiter 1, C.H. Schmitz 1,2 , A.Poellinger 1 1Charité University Medicine Berlin 2NIRx Medizintechnik GmbH, Berlin OSA Biomed 28 April - 2 May 2012, Miami, Florida U N I V E R S I T Ä T S M E D I Z I N B E R L I N [email protected] Introduction Why alternatives to the gold standard of X-ray mammography? • Very reader- dependent diagnosis • Need for a better differentiation between malignant and benign lesions • Non-invasive (radioation free) alternative for breast cancer screening and monitoring preferred General Benefits of optical mammography: • Non- invasive, no radiation • Tomographic imaging • Functional information • Dynamic imaging possible - Ability to track changes of internal parameters (Hb, HbO etc) or extrinsic contrast agents over time U N I V E R S I T Ä T S M E D I Z I N B E R L I N [email protected] Motivation Fast 3D Diffuse Optical Imaging (SR >1 Hz): • Early Bolus kinetics can now be adequately imaged 10 -4 bolus injection Measuring early bolus kinetics over the entire breast can help differentiating between malignant and benign or healthy breast tissue U N I V E R S I T Ä T S M E D I Z I N B E R L I N [email protected] Fast 3D Diffuse Optical Imaging System Optical Mammography with 1.9 Hz Temporal Resolution - DYNOT 232 optical tomography system (NIRx Medizintechnik, Berlin, Germany//NY, USA) Study- 31 co-located Design: source/detector fibers: 961 S/D, 760 and 830nm -- 22 ~ 2 patients: complete 14 volume malignant scans + 8 per benign second lesions, 3 controls -- Reconstruction 25mg ICG bolus (NIRx within NAVI 5-10 Software) sec of relative absorption changes in each of 2243 FEM nodes/ 14000 isometric voxel P. SchneiderC. H. Schmitz et al. etRofo al., Rev.,183(10):956-63 Sci. Instrum. (2011).(2002) U N I V E R S I T Ä T S M E D I Z I N B E R L I N [email protected] Bolus Peak Mapping Time-to-Peak Peak Amplitude 10 -4 fast slow relative absorption changes [-] Timeframes 1 frame = 0.5 s U N I V E R S I T Ä T S M E D I Z I N B E R L I N [email protected] Reader- dependent visual inspection Results healthy non-cancer tumor tumor In general: A priori information about the localization of the lesion and an experience reader is necessary. U N I V E R S I T Ä T S M E D I Z I N B E R L I N [email protected] Peak-Time grouped Amplitude (PTA) Mean time courses of voxel with equal peak time TTP =0.5sec TTP =1sec 200 200 TTP =1.5sec TTP =2sec malignant benign TTP =5sec 150 150 TTP =7.5sec mean breast 100 100 50 50 0 0 normalized absorption relative changes [%] -10 0 10 20 30 -10 0 10 20 30 40 Time [sec] Time [sec] Case1: 53y, 33mm Case2: 22y, invasive ductal carcinoma 22mm fibroadenoma 0 10 TTP [sec] 0 10 TTP [sec] U N I V E R S I T Ä T S M E D I Z I N B E R L I N [email protected] Mean PTA-curves over all patients Mean peak time grouped amplitudes (PTA) over time ** 2 100 1 0 BENIGN (8) 50 MALIGNANT (14) Standardized Mean Difference [a.u.] MeanGrouped Time Peak [%] Amplitude SMD Maximum SMD -1 0 2 4 6 8 10 12 Time To Peak [sec] Significant Difference of PTA at TTP= 1.5sec between the malignant and benign lesions (Wilcoxen test, p=0.0015) U N I V E R S I T Ä T S M E D I Z I N B E R L I N [email protected] Reader independent Classification Approach Mirrored ROC curve Mean peak time grouped amplitudes (PTA) over time 2 1 0.8 100 1 0.6 0 0.4 BENIGN (8) 50 MALIGNANT (14) Standardized Mean Difference [a.u.] Mean Peak Time Grouped Amplitude [%] SMD 0.2 AUC=0.92 Classifying Threshold True positive rate(Sensitivity) -1 0 2 4 6 8 10 12 Time To Peak [sec] 0 0 0.2 0.4 0.6 0.8 1 True negative rate (Specificity) Decision Boundary for Reader Independent Classification: PTA = 84.4% of the mean bolus signal P. Schneider et al. Rofo ,183(10):956-63 (2011). U N I V E R S I T Ä T S M E D I Z I N B E R L I N [email protected] Classification Rates for 22 patients Malignant, Detection Benign, Detection mean lesion size (range) Rate mean lesion size (range) Rate Invasive ductal carcinoma , Fibro-cystic 29mm (8-51mm) 8 / 9 mastopathy, 11mm 1 / 1 Invasive lobular carcinoma, Fibroadenoma, 24mm 25mm 1 / 1 (10-51mm) 5/ 6 Invasive lobular ductal Pseudoangiomatous 0/1 stromalhyperplasia 1/ 1 carcinoma, 17mm (PASH), (44mm) Metaplastic carcinoma, 28mm (19-37mm) 2 / 2 Ductal carcinoma in situ, 80mm 1 / 1 Sum 12 / 14 Sum 7/8 P. Schneider et al. Rofo ,183(10):956-63 (2011). U N I V E R S I T Ä T S M E D I Z I N B E R L I N [email protected] Summary • High-frame rate DOT allows to adequately image early bolus kinetics. • Extracting “Peak time grouped amplitudes” out of those kinetic curves can be used for an automatic differentiation between malignant and benign breast lesions. Mean peak time grouped amplitudes (PTA) over time 2 100 1 0 BENIGN (8) 50 MALIGNANT (14) [a.u.] Difference Mean Standardized Mean Peak Time Grouped Amplitude [%] [%] Amplitude Grouped Time Peak Mean SMD Classifying Threshold -1 0 2 4 Time To Peak6 [sec] 8 10 12 Published: P. Schneider et al. ,“ Fast 3D Near-Infrared Breast Imaging Using Indocyanine Green for Detection and Characterization of Breast Lesions,“ Rofo,183(10):956-63(2011). U N I V E R S I T Ä T S M E D I Z I N B E R L I N [email protected] Outlook • To further investigate the robustness of the suggested approach more patient data are needed. • Cooperation with the Machine Learning Group of the Technical University Berlin – Classifying PTA –values at TTP=1.5 sec : mean loss: 0.19±0.02 – Classifying PTA curves ( TTP: 0 – 40sec) : mean loss: 0.10±0.03 U N I V E R S I T Ä T S M E D I Z I N B E R L I N [email protected] Acknowledgements Special thanks to my colleagues and cooperators: Dept. of Radiology P. Schneider, Charité University Medicine Berlin N. Volkwein, N. Schreiter, A. Poellinger NIRx Medizintechnik GmbH Berlin C.H. Schmitz Berlin NeuroImaging Center, Charite Machine Learning Group S. Fazli Technical University Berlin B. Blankertz U N I V E R S I T Ä T S M E D I Z I N B E R L I N [email protected] Thank you for your attention! U N I V E R S I T Ä T S M E D I Z I N B E R L I N [email protected].
Details
-
File Typepdf
-
Upload Time-
-
Content LanguagesEnglish
-
Upload UserAnonymous/Not logged-in
-
File Pages14 Page
-
File Size-