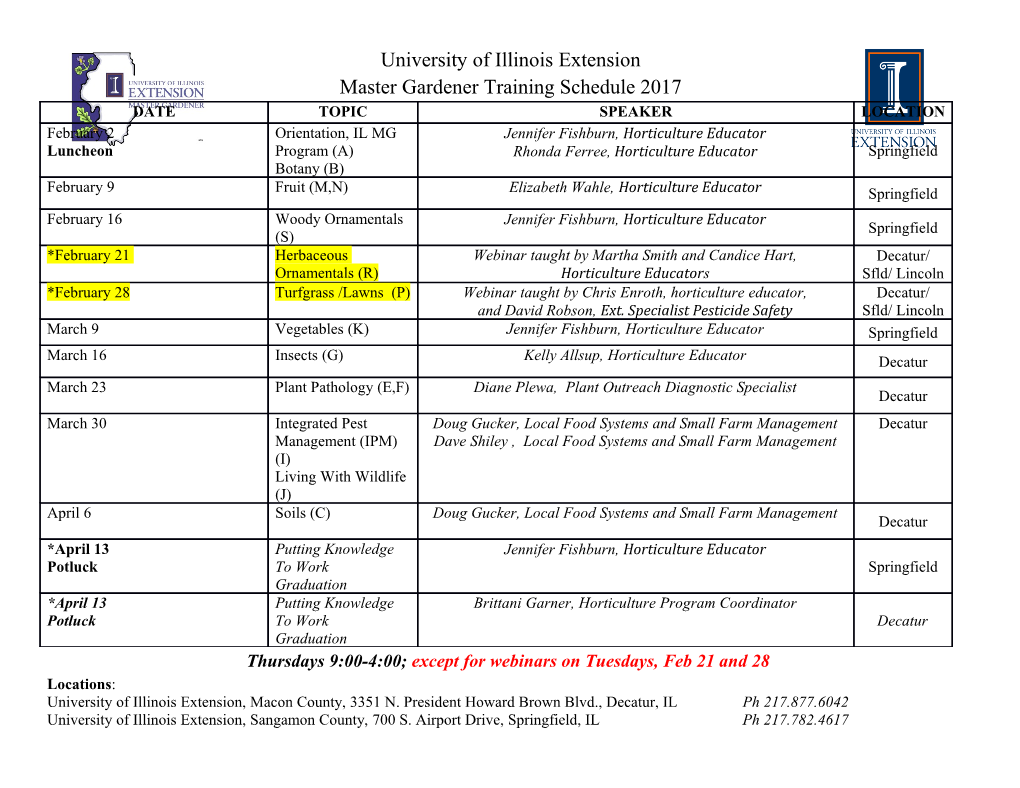
Automatic Autocorrelation and Spectral Analysis Piet M.T. Broersen Automatic Autocorrelation and Spectral Analysis With 104 Figures 123 Piet M.T. Broersen, PhD Department of Multi Scale Physics Delft University of Technology Kramers Laboratory Prins Bernhardlaan 6 2628 BW, Delft The Netherlands British Library Cataloguing in Publication Data Broersen, Piet M. T. Automatic autocorrelation and spectral analysis 1.Spectrum analysis - Statistical methods 2.Signal processing - Statistical methods 3.Autocorrelation (Statistics) 4.Time-series analysis I.Title 543.5’0727 ISBN-13: 9781846283284 ISBN-10: 1846283280 Library of Congress Control Number: 2006922620 ISBN-10: 1-84628-328-0 e-ISBN 1-84628-329-9 Printed on acid-free paper ISBN-13: 978-1-84628-328-4 © Springer-Verlag London Limited 2006 MATLAB®is a registered trademark of The MathWorks, Inc., 3 Apple Hill Drive, Natick, MA 01760-2098, U.S.A. http://www.mathworks.com Apart from any fair dealing for the purposes of research or private study, or criticism or review, as permitted under the Copyright, Designs and Patents Act 1988, this publication may only be reproduced, stored or transmitted, in any form or by any means, with the prior permission in writing of the publishers, or in the case of reprographic reproduction in accordance with the terms of licences issued by the Copyright Licensing Agency. Enquiries concerning reproduction outside those terms should be sent to the publishers. The use of registered names, trademarks, etc. in this publication does not imply, even in the absence of a specific statement, that such names are exempt from the relevant laws and regulations and therefore free for general use. The publisher makes no representation, express or implied, with regard to the accuracy of the infor- mation contained in this book and cannot accept any legal responsibility or liability for any errors or omissions that may be made. Printed in Germany 987654321 Springer Science+Business Media springer.com To Rina Preface If different people estimate spectra from the same finite number of stationary stochastic observations, their results will generally not be the same. The reason is that several subjective decisions or choices have to be made during the current practice of spectral analysis, which influence the final spectral estimate. This applies also to the analysis of unique historical data about the atmosphere and the climate. That might be one of the reasons that the debate about possible climate changes becomes confused. The contribution of statistical signal processing can be that the same stationary statistical data will give the same spectral estimates for everybody who analyses those data. That unique solution will be acceptable only if it is close to the best attainable accuracy for most types of stationary data. The purpose of this book is to describe an automatic spectral analysis method that fulfills that requirement. It goes without saying that the best spectral description and the best autocorrelation description are strongly related because the Fourier transform connects them. Three different target groups can be distinguished for this book. Students in signal processing who learn how the power spectral density and the autocorrelation function of stochastic data can be estimated and interpreted with time series models. Several applications are shown. The level of mathe- matics is appropriate for students who want to apply methods of spectral analysis and not to develop them. They may be confident that more thorough mathematical derivations can be found in the referenced literature. Researchers in applied fields and all practical time series analysts who can learn that the combination of increased computer power, robust algorithms, and the improved quality of order selection have created a new and automatic time series solution for autocorrelation and spectral estimation. The increased computer power gives the possibility of computing enough candidate models such that there will always be a suitable candidate for given data. The improved order-selection quality always guarantees that one of the best candidates will be selected automatically and often the very best. The data themselves decide which is their best representation, and if desired, they suggest possible alter- natives. The automatic computer program ARMAsel provides their language. viii Preface Time series scientists who will observe that the methods and algorithms that are used to find a good spectral estimate are not always the methods that are preferred in asymptotic theory. The maximum likelihood theory especially has very good asymptotic theoretical properties, but the theory fails to indicate what sample sizes are required to benefit from those properties in practice. Maximum likelihood estimation often fails for moving average parameters. Furthermore, the most popular order-selection criterion of Akaike and the consistent criteria perform rather poorly in extensive Monte Carlo simulations. Asymptotic theory is concerned primarily with the optimal estimation of a single time series model with the true type and the true order, which are considered known. It should be a challenge to develop a sound mathematical background for finite-sample estimation and order selection. In finite-sample practice, models of different types and orders have to be computed because the truth is not yet known. This will always include models of too low orders, of too high orders, and of the wrong type. A good selection criterion has to pick the best model from all candidates. Good practical performance of simplified algorithms as a robust replacement for truly nonlinear estimation problems is not yet always understood. The time series theory in this book is limited to that part of the theory that I consider relevant for the user of an automatic spectral analysis method. Those subjects are treated that have been especially important in developing the program required to perform estimation automatically. The theory of time series models presents estimated models as a description of the autocorrelation function and the power spectral density of stationary stochastic data. A selection is made from the numerous estimation algorithms for time series models. A motivation of the choice of the preferred algorithms is given, often supported by simulations. For the description of many other methods and algorithms, references to the literature are given. The theory of windowed and tapered periodograms for spectra and lagged products for autocorrelation is considered critically. It is shown that those methods are not particularly suitable for stochastic processes and certainly not for automatic estimation. Their merit is primarily historical. They have been the only general, feasible, and practical solutions for spectral analysis for a long period until about 2002. In the last century, computers were not fast enough to compute many time series models, to select only one of them, and to forget the rest of the models. ARMAsel has become a useful time series solution for autocorrelation and spectral estimation by increased computer power, together with robust algorithms and improved order selection. Piet M.T. Broersen November 2005 Contents 1 Introduction ...................................................................................................... 1 1.1 Time Series Problems................................................................................ 1 2 Basic Concepts ................................................................................................ 11 2.1 Random Variables ................................................................................... 11 2.2 Normal Distribution ................................................................................ 14 2.3 Conditional Densities .............................................................................. 17 2.4 Functions of Random Variables .............................................................. 18 2.5 Linear Regression.................................................................................... 20 2.6 General Estimation Theory...................................................................... 23 2.7 Exercises.................................................................................................. 26 3 Periodogram and Lagged Product Autocorrelation.................................... 29 3.1 Stochastic Processes................................................................................ 29 3.2 Autocorrelation Function......................................................................... 31 3.3 Spectral Density Function ....................................................................... 33 3.4 Estimation of Mean and Variance ........................................................... 38 3.5 Autocorrelation Estimation ..................................................................... 40 3.6 Periodogram Estimation .......................................................................... 49 3.7 Summary of Nonparametric Methods ..................................................... 55 3.8 Exercises.................................................................................................. 56 4 ARMA Theory ................................................................................................ 59 4.1 Time Series Models................................................................................. 59 4.2 White Noise............................................................................................
Details
-
File Typepdf
-
Upload Time-
-
Content LanguagesEnglish
-
Upload UserAnonymous/Not logged-in
-
File Pages300 Page
-
File Size-