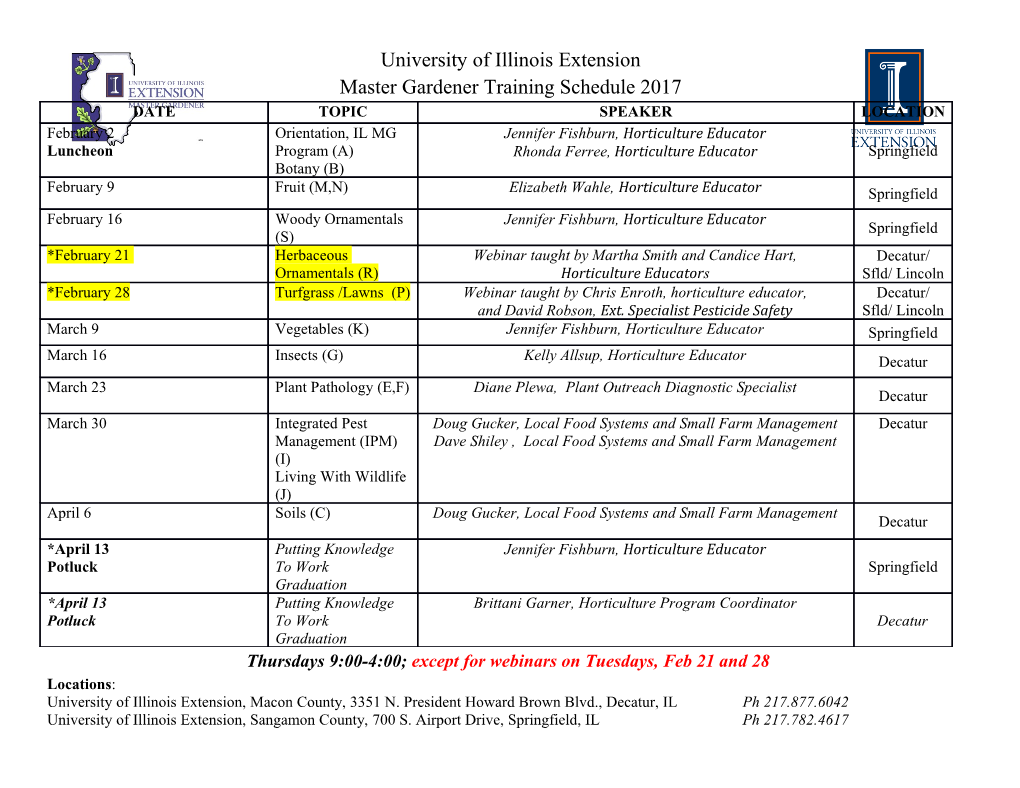
Michigan Technological University Digital Commons @ Michigan Tech Dissertations, Master's Theses and Master's Dissertations, Master's Theses and Master's Reports - Open Reports 2012 Automatic Food Intake Assessment Using Camera Phones Fanyu Kong Michigan Technological University Follow this and additional works at: https://digitalcommons.mtu.edu/etds Part of the Computer Engineering Commons Copyright 2012 Fanyu Kong Recommended Citation Kong, Fanyu, "Automatic Food Intake Assessment Using Camera Phones", Dissertation, Michigan Technological University, 2012. https://doi.org/10.37099/mtu.dc.etds/494 Follow this and additional works at: https://digitalcommons.mtu.edu/etds Part of the Computer Engineering Commons AUTOMATIC FOOD INTAKE ASSESSMENT USING CAMERA PHONES By Fanyu Kong A DISSERTATION Submitted in partial fulfillment of the requirements for the degree of DOCTOR OF PHILOSOPHY (Computer Engineering) MICHIGAN TECHNOLOGICAL UNIVERSITY 2012 c 2012 Fanyu Kong This dissertation, "Automatic Food Intake Assessment Using Camera Phones," is hereby approved in partial fulfillment of the requirements for the Degree of DOCTOR OF PHI- LOSOPHY IN COMPUTER ENGINEERING. Department of Electrical and Computer Engineering Signatures: Dissertation Advisor Dr. Jindong Tan Department Chair Dr. Daniel R. Fuhrmann Date Dedication To my wife and my parents! . Contents List of Figures . .xviii List of Tables ......................................xix Preface .........................................xxi Acknowledgments . .xxiii Abstract .........................................xxv 1 Introduction .................................... 1 1.1 Obesity: A challenge to public health . .1 1.2 Food intake assessment . .3 1.3 Opportunity and challenges . .4 1.4 Goals and specific aims . .5 1.5 Organization of this thesis . .6 Reference . .7 vii 2 DietCam: Automatic Dietary Assessment with Mobile Camera Phones . 11 Abstract . 11 2.1 Introduction . 11 2.2 System Architecture . 14 2.3 Food Classification . 17 2.3.1 Food Features . 17 2.3.2 Food Recognition . 18 2.3.3 Food Segmentation . 20 2.3.4 Occlusions and Redundancies . 20 2.4 Volume and Calorie Estimation . 21 2.4.1 Camera Calibration . 22 2.4.1.1 Intrinsic Parameters Calibration . 22 2.4.1.2 Extrinsic Parameters Calibration . 23 2.4.2 3D Model Reconstruction . 24 2.4.3 Volume Calculation . 25 2.4.4 Calorie Estimation . 26 2.5 Food Database . 26 2.6 Client-Server Architecture . 27 2.7 Evaluation . 28 viii 2.7.1 Experimental Setup . 28 2.7.2 Implementation . 28 2.7.3 Recognition Accuracy . 30 2.7.4 Volume Calculation . 32 2.7.5 Unrecognized food and residues . 34 2.8 Discussion . 34 2.9 Related Work . 35 2.10 Conclusion and Future Work . 37 Reference . 37 3 DietCam: Multi-View Regular Shape Food Recognition with a Camera Phone 43 Abstract . 43 3.1 Introduction . 44 3.2 Related Work . 47 3.3 Multi-View Food Recognition . 49 3.3.1 Food features . 50 3.3.2 Camera calibration . 51 3.3.3 Perspective distance . 53 3.3.4 Multi-view food recognition . 54 3.3.5 Object recognition from 3D reconstruction . 58 ix 3.4 Implementation . 58 3.5 Experiment . 59 3.5.1 Dataset . 59 3.5.2 Baseline methods . 61 3.5.3 Segmentation results . 62 3.5.4 Classification results . 62 3.6 Discussion . 64 3.7 Conclusion . 66 Reference . 66 4 DietCam: Multi-view, Multi-class Food Recognition Using a Multi-kernel Based SVM ......................................... 71 Abstract . 71 4.1 Introduction . 71 4.2 Related Work . 75 4.3 Ingredient detection . 76 4.4 Food classification . 80 4.4.1 Multiple viewpoints . 83 4.4.2 Multi-kernel . 85 4.5 Dataset . 86 x 4.6 Experiment . 87 4.6.1 Ingredient detection . 87 4.6.2 Food classification . 91 4.7 Discussion . 94 4.8 Conclusion . 96 Reference . 96 5 Indexing of Bags of Features: Efficient Image Retrieval from Large-Scale Database ......................................103 Abstract . 103 5.1 Introduction . 104 5.2 Related Work . 106 5.3 Short Representations of Images . 107 5.3.1 The average of SIFT features . 108 5.3.2 The weighted average of SIFT features . 108 5.3.3 PCA of SIFT features . 109 5.3.4 Image distinctiveness of SRs . 110 5.4 Binary index of short representations . 111 5.4.1 Locality similarity hashing . 112 5.4.2 Spatial hashing . 113 xi 5.5 Food Database . 114 5.6 Experiment . 115 5.6.1 Short representation . 115 5.6.2 Image indexing . 117 5.7 Conclusion . 119 Reference . 120 6 DietVolume: Food Volume Estimation through Metric 3D Reconstruction on a Mobile Phone ....................................125 Abstract . 125 6.1 Introduction . 126 6.2 Related work . 129 6.2.1 Structure from motion . 129 6.2.2 Visual odometry . 130 6.2.3 Egomotion . 130 6.2.4 Image based food volume estimation . 131 6.3 Problem Formulation . 132 6.3.1 3D model reconstruction . 132 6.3.2 Scale estimation . 133 6.3.3 Volume calculation . 135 xii 6.4 3D model reconstruction . 135 6.5 Scale factor estimation . 137 6.6 Volume Calculation . 141 6.7 Implementation and Visualization . 142 6.8 Experiment . 143 6.9 Conclusion . 145 Reference . ..
Details
-
File Typepdf
-
Upload Time-
-
Content LanguagesEnglish
-
Upload UserAnonymous/Not logged-in
-
File Pages194 Page
-
File Size-