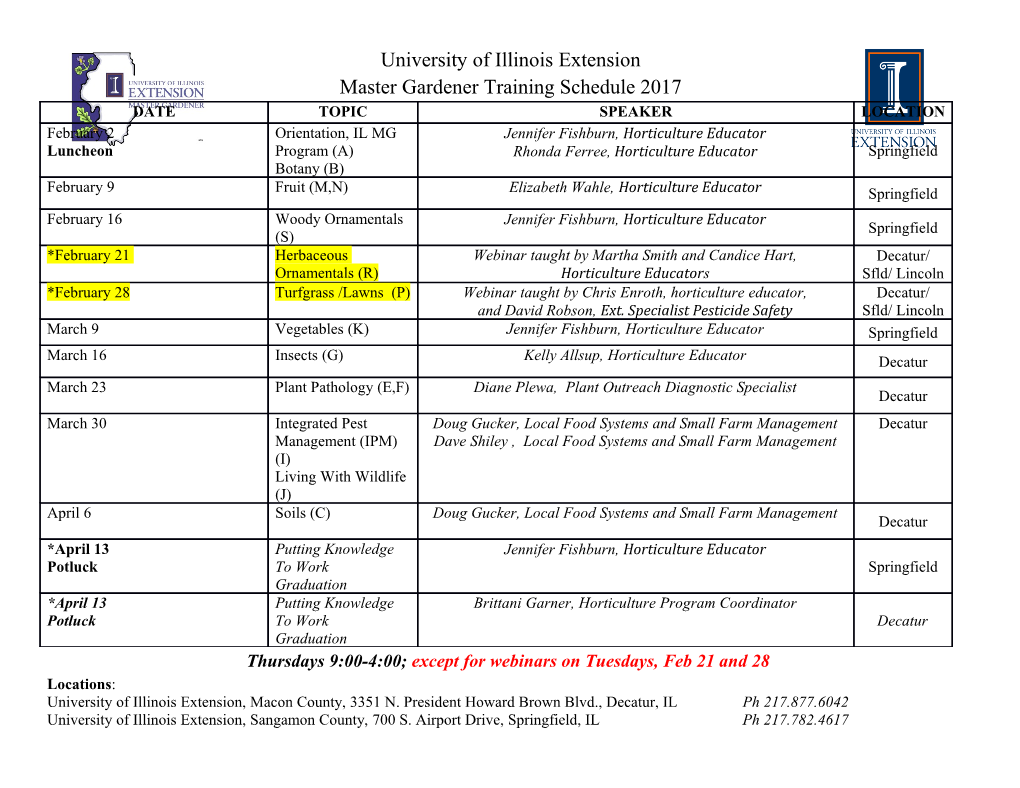
Analysis Methods for Area Data Introductory Methods for Values are associated with a fixed set of areal units covering the Area Data study region We assume a value has been observed for all areas Bailey and Gatrell Chapter 7 The areal units may take the form of a regular lattice or irregular units Lecture 17 November 11, 2003 Analysis Methods for Area Data Analysis Methods for Area Data Objectives For continuous data models we were more concerned with explanation of patterns in terms of locations § Not prediction - there are typically no unobserved values, the attribute is exhaustively measured. In this case explanation is in terms of covariates measured over the same units as well as in terms of the spatial § Model spatial patterns in the values associated with fixed arrangement of the areal units areas and determine possible explanations for such patterns Examples Relationship of disease rates and socio-economic variables 1 Analysis Methods for Area Data Analysis Methods for Area Data {Y (s), s Î R} Random variable Y indexed by locations Explore these attribute values in the context of global trend or first order variation and second order variation – the Random variable Y indexed by a fixed spatial arrangement of the set of areas {Y ( Ai ), ?i Î R} set of areal units § First order variation as variation in the of mean, mi of Yi A1 ÈL È An = R The set of areal units cover the study region R § Second order variation as variation in the COV(Yi , Yj ) Conceive of this sample as a sample from a super population – all realizations of the process over these areas that might ever occur. The sample observations are one possible realization Visualizing Area Data Visualizing Area Data Can use proportional symbols superimposed over the areal units Class intervals compress attribute ranges into a relatively small number of discrete values. Symbols are proportionate to the attribute value of the area Number of classes Can use choropleth maps – each area is shaded according the Should be a function of the range of data variability the attribute value associated with the areal unit Limited by human perception to ~8 classes § Attribute of interest is scaled to a set of discrete ranges or classes Rule of thumb: # classes = 1 + 3.3 log n § Each zone is shaded or colored according to its attribute value 5 observations = 3 classes 20 observations = 6 classes 40 observations = 7 classes 200 observations = 8 classes 1500 observations = 11 classes 2 Visualizing Area Data Visualizing Area Data Visual outcome depends heavily on class interval choice, color Same class intervals as applied to continuous data and shading § Equal Intervals - for fairly uniformly distributed data Use of discrete classes to assign colors can give false impressions of both uniformity (within units) and discontinuity (between units) § Trimmed equal intervals - handles a few outliers from a uniform distribution § Percentiles - rank data and get x evenly distributed classes of width 1/x § Quartile map - 4 classes, lowest quartile, second Equal Intervals Quantiles quartile, third, and highest § Standard Deviates - divide data into units of standard deviation around the mean Visualizing Area Data Visualizing Area Data Problems with choropleth maps Arbitrariness of the areal units Natural Breaks Standard deviates What was the origin of areal unit boundaries? Designed for convenience or efficiency rather than reflection of the underlying spatial pattern – most enumeration units Typically referred to as modifiable area unit problem - MAUP 3 Modifiable Area Unit Problem Visualizing Area Data Different zones will produce virtually any numbers from the same underlying distribution Problems with choropleth maps mean 3.75 var 0.50 mean 3.75 var 0.00 Original data (individuals § Large areas tend to dominate the information content of the map living in households Mean 3.75 var 2.6 § Area shading of attribute values means two visual variables apply to the data – color and area mean 3.75 var 0.93 mean 3.17 var 2.11 § Should not map absolute numbers with a choropleth map § With absolute counts there is no relation of the attribute variable to the absolute and/or relative size of the spatial units § Choropleth maps are not appropriate for counts unless the data are corrected for area, population or some other measure Source Jelenski and Wu (1996) "The modifiable area unit problem and implications that factors out the size of the enumeration districts. for landscape ecology". Landscape Ecology 11(3) p. 129 -140. Visualizing Area Data Map of murder rates per 100,000 US and Canada n The problem of class limits § Use of continuous shading n Area dependence problem § Correct for population or area § Transform areas to be proportionate to the attribute value 4 Corrects for Cartogram - Attempt to correct for area or population population Cartograms There are two distinct and conflicting goals in the construction of cartograms: adjusting region sizes retaining region shapes 5 Cartograms Cartograms Relaxation of contiguity and shape constraints A non-continuous population cartogram based on the population values for the census zones of Leicestershire created using Dorling's 'circle growing' algorithm. Both the circle areas and shades are proportionate to the zone populations. Comparison of traditional choropleth map with cartogram showing percent of male Cartograms population of working age (1891) Cartograms represent areas in relation to their population size. The traditional map gives the impression that the majority of areas have approximately 56% (yellow, brown) of the male population of working age. Patterns are displayed in relation to the number of people involved instead of the size of the area involved. The cartogram gives a different overall view - the majority of areas have a male population of working age of over 60% (red and purple on the map). 6 Exploring Area Data Proximity Measures ì1 centroid of Aj is one of k nearest centroids to that of Ai n Approaches for examining mean values over the areal units wij í î0 otherwise n Techniques for exploring spatial dependence ì1 centroid of Aj is within distance d of centroids of Ai wij í î0 otherwise What was essential information for exploration of these ì1 Aj shares a boundary with Ai with point and continuous data? wij í î0 otherwise g if intercentroid distance d < d Need measures of proximity for irregular areal units ìd ij ij wij í î 0 otherwise Proximity Measures Proximity Measures A A A A A A A 1 2 3 4 5 6 7 A1 A2 A3 A4 A5 A6 A7 A A2 1 æ0 1 1 0 0 1 1ö æ 0 1 1 0 0 0 1ö A4 ç ÷ A ç ÷ A 0 1 1 0 0 0 2 A4 1 0 1 1 0 0 0 2 ç ÷ ç ÷ A1 A3 ç ÷ ç ÷ A3 0 1 1 1 0 A A3 0 1 0 1 1 0 0 ç ÷ 1 ç ÷ A5 W = A ç 0 1 0 0÷ W =ç 0 1 1 0 1 0 0÷ 4 A5 A7 A6 ç ÷ ç ÷ A 0 1 0 A7 0 0 1 1 0 1 0 5 ç ÷ A6 ç ÷ A ç 0 1÷ ç 0 0 1 0 1 0 1÷ 6 ç ÷ ç ÷ 0 1 1 0 0 0 1 0 A7 è ø è ø Adjacency k nearest centroids The spatial proximity matrix W with elements wij represents a Not symmetric measure of proximity of Ai to Aj 7 Proximity Measures Spatial Moving Averages Proximity measures can be specified as measures of different One way to estimate the mean is by the average of the orders – spatial lags values in “neighboring” areas The proximity matrix W represents the neighbors These can be represented as different proximity matrices for different lags n å wij y j For example W represents first spatial lag, W the j=1 1 2 mˆi = second spatial lag, etc n å wij j=1 Median Polish If areal units are a regular grid can use median polish to estimate the trend Median is more resistant to outliers in the data than the mean Each value is decomposed into: yij = m + mi + m j + eij where m is a fixed overall effect mi and mj represent fixed row and column effects eij is a random error term The overall mean is mij = m + mi + m j 8 Median Polish algorithm Median Polish algorithm 5. Repeat steps 1-4 until no changes occur with the row or column 1. Take the median of each row and record the value to the side of medians the row – subtract the row median from each value in that row 1) s+1 3a) s+1 3 4 5 -1 0 1 4 -1 0 1 -1 2. Compute the median of the row medians , and record the value 0 -1 1 5 0 -1 1 0 0 1 0 5 0 1 0 0 as the overall effect, Subtract the overall effect from each of the 5 4 6 r+1 0 0 0 0 r+1 0 0 1 5 row medians 5 6 5 3b) s+1 2a) s+1 -1 0 0 -1 3. Take the median of each column and record the value beneath -1 0 1 4 1 2 3 s+1 0 -1 0 0 0 -1 1 5 the column, Subtract the column median from each value in that 1 3 4 5 0 0 1 -1 0 0 1 0 5 r+1 0 0 1 5 particular column 2 5 4 6 0 3 5 6 5 0 r+1 0 0 0 5 r+1 0 0 0 0 4. Compute the median of the column medians, and add the values 2b) s+1 4a) s+1 -1 0 1 -1 -1 0 0 0 to the current overall effect.
Details
-
File Typepdf
-
Upload Time-
-
Content LanguagesEnglish
-
Upload UserAnonymous/Not logged-in
-
File Pages11 Page
-
File Size-