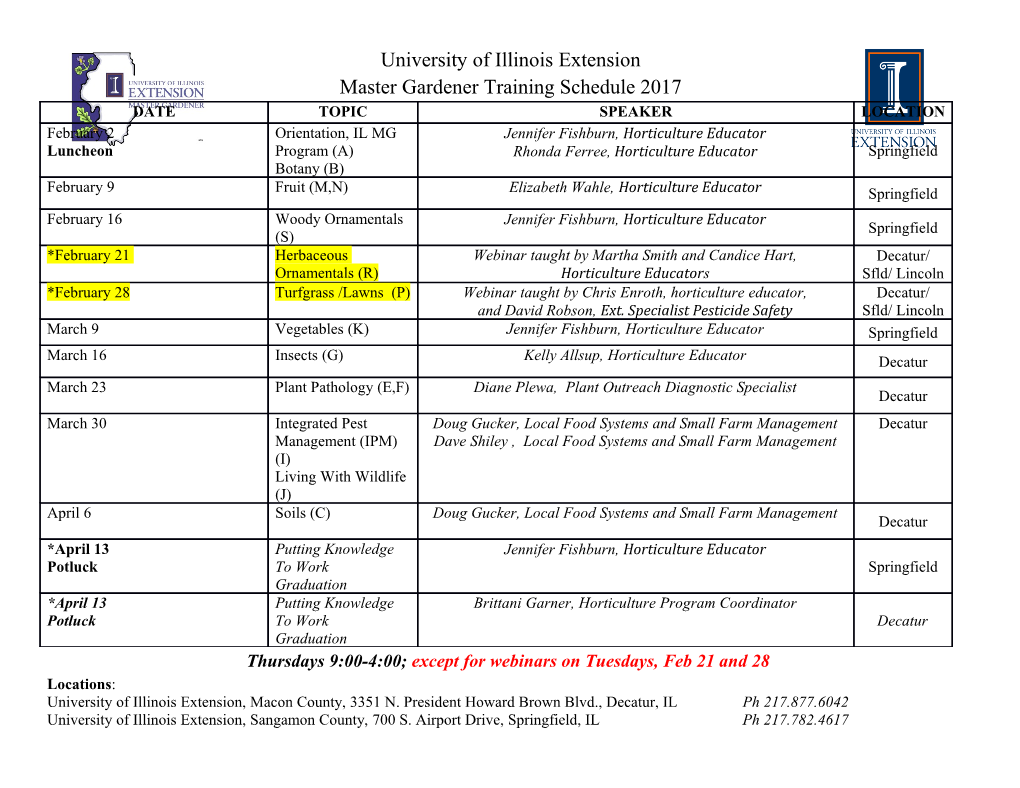
Probability Theory and Related Fields manuscript No. (will be inserted by the editor) Exchangeable graph-valued Feller processes Harry Crane Received: date / Accepted: date Abstract The transition law of every exchangeable Feller process on the space of countable graphs is determined by a σ-finite measure on the space of 0; 1 0; 1 -valued arrays. In discrete time, this characterization gives rise tof ag construction × f g from an independent, identically distributed sequence of exchangeable random functions. In continuous time, the behavior is enriched by a Levy–It´ o–Khintchine-typeˆ decomposition of the jump measure into mu- tually singular components that govern global, vertex-level, and edge-level dynamics. Every such process almost surely projects to a Feller process in the space of graph limits. Keywords exchangeability random graph graph limit Levy–It´ o–ˆ Khintchine decomposition · Aldous–Hoover· theorem Rado· graph Erdos–R˝ enyi´ random graph· · · Mathematics Subject Classification (2000) 05C80 60G09 60J05 60J25 · · · 1 Introduction A graph, or network, G = (V; EG) is a set of vertices V and a binary relation of ij edges EG V V. For i; j V, we write G to indicate the status of edge (i; j), ⊆ × 2 that is, ( ij 1; (i; j) EG; G := 1 (i; j) EG := 2 f 2 g 0; otherwise. ij Both the pair G = (V; EG) and the array G = (G )i;j V represent the same object. 2 We write V to denote the space of graphs with vertex set V. ConsiderG a population of individuals labeled distinctly in V and related to one another by the edges of a graph G = (V; EG). The population size is typi- cally large, but unknown, and so we assume a countably infinite population Rutgers University, Department of Statistics & Biostatistics, 110 Frelinghuysen Road, Piscataway, NJ 08854. [email protected] 2 Harry Crane and take V = N := 1; 2;::: . In practice, the labels N are arbitrarily assigned for the purpose of distinguishingf g individuals, and data can only be observed for a finite sample S N. Since labels are assigned arbitrarily, we often take S = [n]:= 1;:::; n whenever⊂ S N is finite with cardinality n 1. f g ⊆ ≥ These practical matters relate to the operations of ij – relabeling: the relabeling of G = (G )i;j 1 by any permutation σ : N N is ≥ ! σ σ(i)σ(j) G := (G )i;j 1; and (1) ≥ ij – restriction: the restriction of G = (G )i;j 1 to a graph with vertex set S N is ≥ ⊆ S ij G = G S := (G )i;j S: (2) j 2 In many relevant applications, the network structure changes over time, re- sulting in a time-indexed collection (Gt)t T of graphs. We consider exchange- able Markov processes as statistical models2 in this setting. 1.1 Graph-valued Markov processes A V-valued Markov process is a collection Γ = Γ : G V for which each G f G 2 G g ΓG = (Γt)t T is a family of random graphs that has Γ0 = G and 2 – the Markov property, that is, the past (Γs)s<t and future (Γs)s>t are condition- ally independent given the present Γt for all t T. 2 We also assume Γ is – exchangeable, that is, all ΓG share a common transition law for which σ σ P Γt Γt = F = P Γ Γ = F ; (3) f 0 2 · j g f t0 2 · j t g for all permutations σ : V V, all F V, and all t0 > t 0, and – consistent, that is, for all S !V the transition2 G law of Γ satisfies≥ ⊆ S S P Γ = F Γt = F0 = P Γ = F Γt = F00 ; F S; (4) f t0 j g f t0 j g 2 G for all F0; F00 V such that F0 S = F00 S. 2 G j j S Consistency implies that Γ = (Γt S)t T satisfies the Markov property for every G j 2 S V, for all G V. We call any Γ satisfying these properties an exchangeable, consistent⊆ Markov2 G process. In Proposition 1, we prove that consistency and the Feller property are equivalent for exchangeable Markov processes on N, so we sometimes also call Γ an exchangeable Feller process. G Any process Γ = ΓG : G V determines a random collection (Γt)t T with a given transitionf law and2 initial G g distribution ν by first drawing Γ 2ν 0 ∼ and then putting Γν = ΓG on the event Γ0 = G. With the underlying process Γ = ΓG : G V understood, we write Γν = (Γt)t T to denote a collection withf this behavior.2 G g 2 Exchangeable graph-valued Feller processes 3 Theorems 1-5 below characterize the behavior of exchangeable N-valued Feller processes in both discrete and continuous time. In discrete time,G every exchangeable Feller process can be constructed by an iterated application of independent, identically distributed (i.i.d.) exchangeable random functions, called rewiring maps; see Section 4.5. In continuous time, Γ admits a con- struction from a Poisson point process whose intensity measure has a Levy–´ Ito–Khintchine-typeˆ decomposition. The Levy–It´ o–Khintchineˆ representation classifies every discontinuity of Γ as one of three types. When ν is an exchangeable initial distribution on N, both discrete and G continuous time processes Γν project to a Feller process in the space of graph limits. These outcomes invoke connections to previous work on the theory combinatorial stochastic processes [4,13], partially exchangeable arrays [1,2, 8], and limits of dense graph sequences [11,12]. 1.2 Outline Before summarizing our conclusions (Section 3), we first go over key defini- tions and assumptions (Section 2). We then explain essential concepts in more detail (Section 4) and prove our main theorems for discrete time (Section 5) and continuous time (Section 6) processes. We make concluding remarks in Section 7. 2 Definitions and assumptions 2.1 Graphs For any graph G = (V; EG), we impose the additional axioms of (i) anti-reflexivity,(i; i) < EG for all i V, and 2 (ii) symmetry,(i; j) EG implies (j; i) EG for all i; j V, 2 2 2 and we specialize V to denote the set of all graphs satisfying (i) and (ii). G By the symmetry axiom (ii), we may write ij = i; j EG to indicate that there is an edge between i and j in G. By definition,fG hasg 2 no multiple edges, condition (i) forbids edges from a vertex to itself, and condition (ii) makes ij G undirected. In terms of the adjacency array G = (G )i;j V, (i) and (ii) above correspond to 2 (i’) Gii = 0 for all i V and (ii’) Gij = Gji for all2i; j V, 2 respectively.As we discuss in Section 7, our main theorems remain valid under some relaxation of these conditions. The above two notions of a graph— as a pair (V; EG) and as an adjacency array—are equivalent. We use them interchangeably and with the same notation. With SV denoting the space of permutations of V N, that is, bijective ⊆ functions σ : V V, relabeling (1) associates every σ SV to a map V V. ! 2 G !G 4 Harry Crane S For all S S0 V, restriction (2) determines a map S0 S, G G = G S. ⊆ ⊆ ij G !G 7! j Specifically, for n m 1, G [m] = (G )1 i;j m is the leading m m submatrix of ij ≥ ≥ j ≤ ≤ × G = (G )1 i;j n. By combining (1) and (2), every injection φ : S S0 determines ≤ ≤ ! a projection S S by G 0 !G φ φ(i)φ(j) G G := (G )i;j S: (5) 7! 2 We call a sequence of finite graphs (Gn)n N compatible if Gn [n] and 2 2 G Gn [m] = Gm for every m n, for all n N. Any compatible sequence of finitej graphs determines a≤ unique countable2 graph G , the projective limit of 1 (G1; G2;:::) with respect to the restriction operation in(2). We endow N with the product discrete topology induced, for example, by the ultrametricG d(G; G0):= 1= sup n N : G n = G0 n ; G; G0 N; (6) f 2 j[ ] j[ ]g 2 G with the convention that 1= = 0. From (6), we naturally equip N with the 1 G Borel σ-field σ [n] n N generated by the restriction maps. Under (6), N is h ·| i 2 G a compact and, therefore, complete and separable metric space; hence, N is Polish and standard measure theoretic facts apply in our analysis. G 2.2 Graph limits For n m 1, F m , and G n , we define the density of F in G by ≥ ≥ 2 G[ ] 2 G[ ] m δ(F; G):= ind(F; G)=n# ; (7) m where ind(F; G) is the number of embeddings of F into G and n# := n(n 1) (n m + 1). Specifically, − ··· − X ind(F; G):= 1 Gφ = F f g φ:[m] [n] ! is the number of injections φ :[m] [n] for which Gφ = F. ! S Given a sequence (Gn)n N in ∗ := n N [n], we define the limiting density 2 G 2 G of F in (Gn)n N by 2 δ(F; (Gn)n N ):= lim δ(F; Gn); if it exists: 2 n !1 For G N, we define the limiting density of F in G by 2 G δ(F; G):= lim δ(F; G [n]); if it exists: (8) n !1 j Definition 1 (Graph limit) The graph limit of G N is the collection 2 G S G := (δ(F; G))F m N [m] ; j j 2 2 G S provided δ(F; G) exists for every F m N [m].
Details
-
File Typepdf
-
Upload Time-
-
Content LanguagesEnglish
-
Upload UserAnonymous/Not logged-in
-
File Pages48 Page
-
File Size-