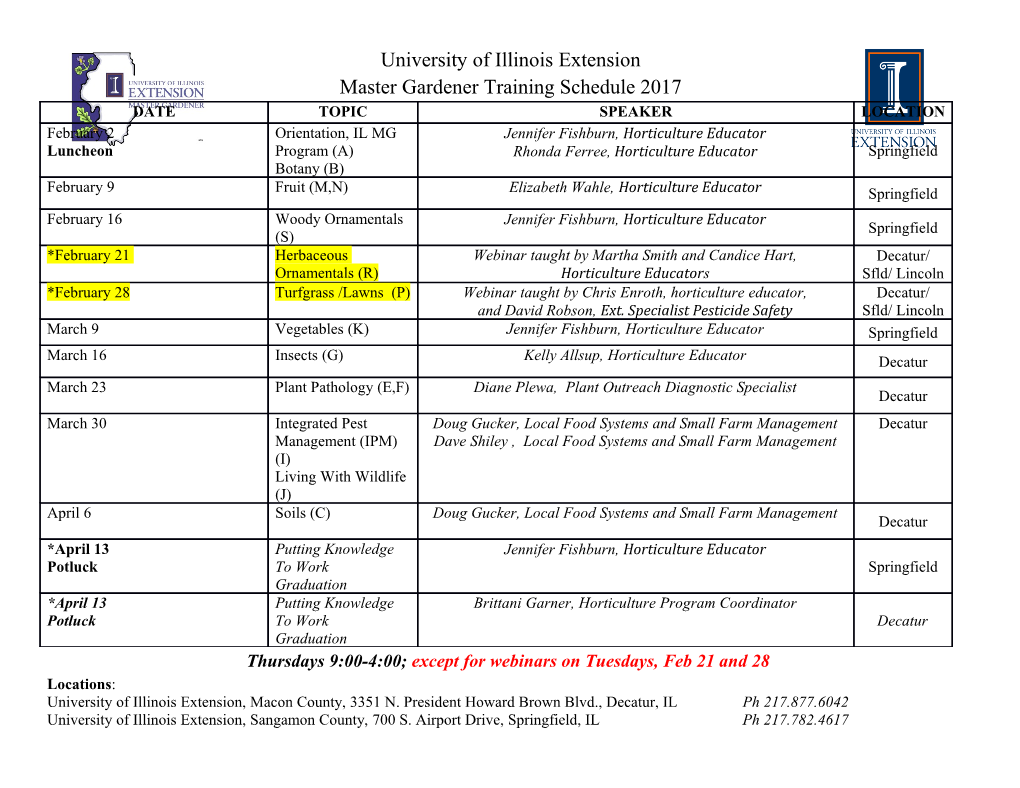
Apache Storm A framework for Parallel Data Stream Processing Storm • Storm is a distributed real-;me computaon plaorm • Provides abstrac;ons for implemen;ng event- based computaons on a cluster of physical nodes • Performs parallel computaons on data streams • Manages high throughput data streams • It can be used to design complex event-driven applicaons on intense streams of data Introduc;on • Began as a project of BackType, a marke;ng intelligence company bought by TwiFer in 2011 • TwiFer open-sourced the project and became an Apache project in 2014 • Storm = the Hadoop for Real-Time processing "Storm makes it easy to reliably process unbounded streams of data, doing for real8me processing what Hadoop did for batch processing.” • Has been designed for massive scalability, supports fault-tolerance with a “fail fast, auto restart” approach to processes, and provides the guarantee that every data of the stream will be processed. • Its default is “at least once” processing seman;cs, but offers the ability to implement also the “exactly once” processing seman;cs (transac;onal) Design Goals • Guaranteed Data processing – no data is lost • Imperave descrip;on of a streaming workflow (through stream manipulaon classes) • Horizontal Scalability • Fault- Tolerance • Programmable in different languages Main Concepts: Spouts and Bolts • Any Storm processing is defined as a Directed Acyclic Graph (DAG) of Spouts and Bolts, which is called a topology. • In the topology, Spouts and Bolts produce and consume a streams of tuples. • Tuple:: are generic objects without any schema, but can have named fields • Spouts:: are the tuple input modules; – can be “unreliable” (fire-and-forget) or “reliable” (replay failed tuples) • Bolts:: are the tuple processing or output modules, – consume streams and poten;ally produce new streams • Stream:: a poten;ally infinite sequence of Tuple objects that Storm serializes and passes to the next bolts in the topology. • Complex stream transformaons o]en require mul;ple steps (a chain of mul;ple bolts) • Storm topologies run on clusters and the Storm scheduler distributes work to nodes around the cluster, based on the topology configuraon. Applicaon represented as a topology Source: Heinze, Aniello, Querzoni, Jerzak, Cloud-based Data Stream Processing, DEBS 2014 • Unlike Map-Reduce jobs, topologies run forever or un;l manually terminated. • Spouts: – bring data into the system and hand the data off to bolts (which may in turn hand data to subsequent bolts) • Bolts: – do the processing on the stream. – may write data out to a database or file system, – send a message to another external system, or – make the results of the computaon available to the users. Typical Bolts • Func;ons – tuple transformaons • Filters • Aggregaon • Joins • Storage/retrieval from persistent stores Applicaon represented as a topology • Storm developer may set “parallelism hints” at elements of the topology. Source: Heinze, Aniello, Querzoni, Jerzak, Cloud-based Data Stream Processing, DEBS 2014 Storm strengths • a rich array of available spouts specialized for receiving data from all types of sources (e.g. from the TwiFer streaming API to Apache Kaa to JMS brokers, etc.) • it is straigh<orward to integrate with HDFS file systems, meaning Storm can easily interoperate with Hadoop, if needed. • Storm has support for mul;-language programming, and spouts and bolts can be wriFen in almost any language. • Storm is a very scalable, fast, fault-tolerant open source system for distributed computaon, with a special focus on calculang rolling metrics in real ;me over streams of data. Data Par;;oning Schemes • When a tuple is emiFed, to which task does it go? • Storm offers some flexibility to define the data par;;oning/ shuffling method • Stream groupings define the data flow in the topology • This is set for every spout and bold through the …grouping method when defining the topology Topology view Task view Types of Stream Grouping • Shuffle grouping - random distribu;on of tuples to the next downstream bolt tasks • Fields grouping – uses one/more named elements of the tuples to determine the des;naon task (by mod hashing) • All grouping – sends all tuples to all all tasks • Global grouping – all tuples go to the bolt task with the lowest Id • Direct grouping – explicit defini;on of the target bolt • Custom grouping – define a custom grouping method by implemen;ng the CustomStreamGrouping interface • LocalOrShuffle grouping: if the target bolt has >1 tasks in the same worker process, tuples will be shuffled to just those in-process tasks. Otherwise, it is the same as normal shuffle Topology with Grouping op;ons [“id1”, “id2”] shuffle bolt bolt spout global [“url”] bolt bolt all A Prac;cal Example: Word Count • Word count: the HelloWorld • Input: stream of text (e.g. from documents) • Output: number of appearance for each word A Prac;cal Example: Hello Storm A simple word count The Strom Topology Topology descripon • Using the Topologybuilder class and its methods setSpout() and setBolt() the spouts and bolts are declared and instan;ated. • setBolt returns an InputDeclarer object that is used to define the inputs to the bolt. With this a bolt explicitly subscribes to a specific stream of another component (spout or bolt), and … • chooses the data shuffling/par;;oning op;on • the parallelizaon hint for spouts and bolts is op;onal • The cluster class (its submitTopology method) is then used to map the topology to a cluster HelloStorm: contains the topology definion IRichSpout IRichSpout: is the interface that any spout must implement. • open method:: allows the spout to configure any connec;ons to the outside world (e.g. connec;ons to queue servers) and to receive the SpoutOutputCollector) • nextTuple method:: will emit (send) the next tuple downstream the topology, it is called repeatedly by the Storm infra-structure • declareOutputFields defies the fields of the tuples of the output streams • Methods ack and fail are called when Storm detects that a tuple emiFed from the Spout either successfully completed the topology, or failed to be completed. LineReaderSpout: reads docs and creates tuples BaseRichBolt Extend the abstract class BaseRichBolt or implement the iRichBolt interface • Prepare method:: passes to the bolt informaon about the topology. The Outputcollector object manages the interac;on between the bolt and the topology (e.g. transming and acknowledging tuples) • Execute method:: does the processing of incoming tuples • The collector.emit() method is used to send the transformed/new tuple to the next bolt. • Through collector.ack() and collector.fail() the bolt can no;fy Storm if the processing of the tuple was successful or if it failed, and for which reason (collector.reportError()) • declareOutputFields method:: is used do declare the fields of the output tuples or to define new named output streams. BaseRichBolt • Bolts can emit more than one stream. To make use of this, declare mul;ple named streams using the declareStream method of OutputFieldsDeclarer interface Name of the stream public void declareOutputFields (OutputFieldsDeclarer d) { ! !d.declare (new Fields (“first””, “second”, “third”))! Name of the fields !d.declareStream(“car”, new Fields(“first”));! !d.declareStream(“cdr”, new Fields(“second”,”third”))! }! • And then specify the named output streams using the emit method on SpoutOutputCollector! public void execute(Tuple input) {! List<Object> objs = input.select( new Fields(“first”,”second”,”third”) );! !collector emit(objs);! !collector emit(“car”, new Values(objs.get(0)));! !collector.emit(“cdr”, new Values(objs.get(1), objs.get(2)));! !collector.ack(input);! }! Access to the tuple fields WordSpliFerBolt: cuts lines into words WordCounterBolt: counts word occurrences Topology Execuon • A Topology processes tuples forever (un;l you kill it). It consists of many worker processes spread across many machines (managed by a supervisor) • A machine in a Cluster may run one or more worker processes. It is either idle or being used by a single topology. Each worker node may run one or more tasks of the same component. • Storm’s default scheduler applies a simple round- robin strategy to assign tasks to worker processes Architecture of a Storm Cluster • Nimbus: – distributes code around the cluster – Assigns tasks to machines/supervisors (i.e. allocates the execu;on of components - spouts – and bolts) - to the worker processes – Failure monitoring – Is fail-fast and stateless • Zookeeper: – Keeps the informaon of which supervisor machines are execu;ng (for discovery and coordinaon purposes) and if Nimbus machine is up. • Supervisor: – Listens to work assigned to its machine – Starts and stops worker processes based on Nimbus commands – Is fast-fail and stateless Tuple Tree Storm considers a tuple coming off a spout "fully processed" when the tuple tree has been exhausted and every message in the tree has been processed. A tuple is considered failed when its tree of messages fails to be fully processed within a specified ;meout. This ;meout can be configured (default is 30 seconds) Tuple emiFed by a spout The tuple tree generated by the processing of a sentence Anchoring • A tuple tree is defined by specifying the input tuple as the first argument of emit. • If the new tuple fails to be processed downstream, the root tuple can be idenfied. At-least-once processing guarantee • With anchoring, Storm can guarantee at-least-once seman;cs (in the presence of failures reported by bolts) without using intermediate queues. • Instead of retrying from the point that a failure has been reported, retries happen from the root of the tuple tree - spouts will simply re-emit the root tuple again. • Intermediate stages of bolt processing that had been completed successfully will be re-done. • This is a waste of processing, … • But has the advantage is there is no need to synchronize the processing of the tuples by the parallel tasks. • And if the operaon of the bolts is idempotent (no side effects) the re-processing actually defines exactly-once processing guarantee. Transac;onal Exactly-once processing guarantee But bolts may not do idempotent processing and processing may require exactly-once seman;cs: • e.g.
Details
-
File Typepdf
-
Upload Time-
-
Content LanguagesEnglish
-
Upload UserAnonymous/Not logged-in
-
File Pages35 Page
-
File Size-