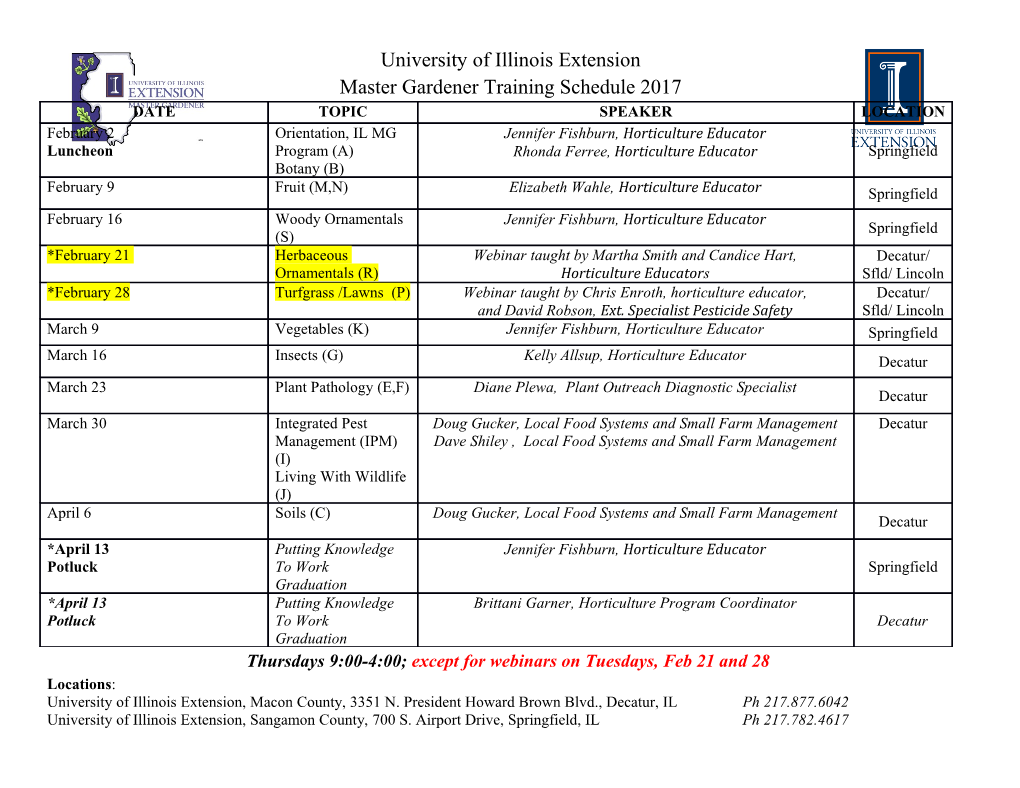
1APRIL 2002 NOTES AND CORRESPONDENCE 793 NOTES AND CORRESPONDENCE Climate Predictions with Multimodel Ensembles VIATCHESLAV V. K HARIN AND FRANCIS W. Z WIERS Canadian Centre for Climate Modelling and Analysis, Meteorological Service of Canada, Victoria, British Columbia, Canada 2 January 2001 and 2 November 2001 ABSTRACT Several methods of combining individual forecasts from a group of climate models to produce an ensemble forecast are considered. These methods are applied to an ensemble of 500-hPa geopotential height forecasts derived from the Atmospheric Model Intercomparison Project (AMIP) integrations performed by 10 different modeling groups. Forecasts are veri®ed against reanalyses from the European Centre for Medium-Range Weather Forecasts. Forecast skill is measured by means of error variance. In the Tropics, the simple ensemble mean produces the most skillful forecasts. In the extratropics, the regression-improved ensemble mean performs best. The ``superensemble'' forecast that is obtained by optimally weighting the individual ensemble members does not perform as well as either the simple ensemble mean or the regression-improved ensemble mean. The sample size evidently is too small to estimate reliably the relatively large number of optimal weights required for the superensemble approach. 1. Introduction to long-range forecasting of the monthly mean tropical The skill of climate predictions is limited by internal Paci®c sea surface temperatures. In a recent paper, De- atmospheric variability that is largely unpredictable be- rome et al. (2001) discussed a linear multivariate method yond the deterministic predictability limit of about two for blending climate forecasts produced by two dynam- weeks (e.g., Lorenz 1982). A standard approach that is ical models. used to reduce climate noise in model predictions is to Ensembles of predictions can be used for determin- average an ensemble of forecasts initiated from different istic or probability forecasting. Doblas-Reyes et al. initial conditions (e.g., Kharin et al. 2001, and refer- (2000) investigate the performance of multimodel cli- ences therein). mate predictions produced by three general circulation With the availability of climate predictions produced models and ®nd that the multimodel approach offers a by several dynamical models, multimodel ensemble systematic improvement when using the ensemble to forecasting has drawn some attention recently. In par- produce probabilistic forecasts. They ®nd that the mul- ticular, two recent studies (Krishnamurti et al. 1999, timodel ensemble improves skill only marginally when 2000) discuss a superensemble approach, in which a verifying the ensemble mean, however. On the other multimodel linear regression technique is used to im- hand, Krishnamurti et al. (2000) ®nd an apparent sys- prove deterministic forecasts locally. tematic improvement in mean square error for a mul- Other studies have also used linear methods to pro- timodel forecast over that of the individual model fore- duce better predictions by combining several indepen- casts. dent forecasts. For example, Danard et al. (1968) and With the increasing popularity of multimodel ensem- Thompson (1977) showed that the mean square error of ble forecasting, it is important to understand its virtues forecasts constructed from a particular linear combi- and limitations. In this paper, we consider deterministic nation of two independent predictions is less than that forecasting only. The goal is to evaluate the performance of the individual predictions. Fraedrich and Smith of several forecasts constructed from a multimodel en- (1989) discussed a linear regression method to combine semble of forecasts, as measured by forecast error var- two statistical forecast schemes and applied this method iance. We use monthly 500-hPa geopotential height data from several model simulations of the 1979±88 period produced for the Atmospheric Model Intercomparison Corresponding author address: Francis W. Zwiers, Canadian Cen- tre for Climate Modelling and Analysis, University of Victoria, P.O. Project (AMIP; Gates 1992). These integrations can be Box 1700, Stn CSC, Victoria, BC V8W 2Y2, Canada. viewed as two-tier climate forecasts (Bengtsson et al. E-mail: [email protected] 1993) in which the lower boundary condition predic- q 2002 American Meteorological Society Unauthenticated | Downloaded 09/28/21 04:12 AM UTC 794 JOURNAL OF CLIMATE VOLUME 15 tions are error free and in which initial conditions at the where Cov(Xi, Xk) and Cov(Xk, Y) are the covariances beginning of each forecast period are not speci®ed. and cross covariances between the model forecasts and The language that is used to describe ensemble-fore- the model forecasts and verifying observations Y, re- casting schemes can be confusing because similar words spectively. are used in the literature to describe schemes with sev- Some studies (e.g., Krishnamurti et al. 2000) have eral different con®gurations. The ensemble of forecasts interpreted the regression coef®cients as indicators of may have been produced with a single dynamical model, the relative model ``reliability.'' However, this interpre- or it may consist of a number of forecasts, each produced tation is not generally correct. To appreciate the dif®- with a different model. Krishnamurti et al. call the latter culty of doing so, consider a simple example in which a superensemble. However, this nomenclature appro- there are two model forecasts X1 and X 2, one that sys- priates a name that more aptly describes ensemble-fore- tematically oversimulates and the other that undersi- casting schemes in which each of several models is used mulates the amplitude of the predictable signal b in the to produce an ensemble of forecasts. In this paper, we observations Y. In particular, suppose use the term ensemble to denote a collection of fore- casts, each of which is produced with a different model. Y 5 b 1 e, X115 0.5b 1 e , and The plan of the paper is the following. First we de- X 5 1.5b 1 e . scribe a general linear regression method that will serve 22 as the basis for constructing several improved forecasts. Here e, e1, and e2 represent the unpredictable internal Results are presented as a function of the size of the variability that is present in the observations and in the multimodel ensemble, and we conclude with a sum- corresponding model forecasts. For convenience, we as- mary. sume that these are independent random variables with 2 0 time mean and the same variances e . The forecasts 2. Multimodel linear regression X1 and X 2 have the same mean square error MSE 5 2 22 2 0.5 ssbe1 2 , where s b is the variance of the predict- Let {Xi(t)}, i 5 1,...,M denote an ensemble of fore- able signal b. The regression coef®cients are given by casts produced by M models at a ®xed location. An ar- 22 2 a1 5 ssbb/(5 1 2 s e ) and a 2 5 3a1. Thus, in this ex- bitrary linear combination of these forecasts is given by ample and in general, equally reliable model forecasts M may not necessarily be weighted equally when com- F(t) 5 a 1 aX(t). (1) 0 O ii bined optimally. Hasselmann (1979) discusses this prob- i51 lem in greater generality and points out that the optimal The M 1 1 coef®cients ai, i 5 0,...,M may be subject coef®cients weight the model forecasts so as to maxi- to some constraints, as is discussed below. Within the mize the signal-to-noise ratio. Similar ideas apply to the bounds of those constrains, coef®cients may be chosen optimal climate change signal detection problem (e.g., to minimize the mean square error, Hasselmann 1997; Zwiers 1999). MSE [ (F 2 Y)225 Var(F 2 Y) 1 B , (2) An attractive property of the multimodel linear re- of the multimodel regression forecast F. Here Y rep- gression forecast Rall is that it is superior to all other resents the verifying observations, the overbar denotes linear combinations of the individual forecasts when time averaging, and B 52FYis the mean bias. De- very large ``training'' datasets are available for esti- pending on the constraints, several versions of this gen- mating the regression coef®cients. In practice, however, eral regression approach are possible, as will be outlined the coef®cients depend on estimates of covariances below. These versions will be identi®ed variously by Cov(Xi, Xk) and Cov(Xk, Y) that must be obtained from symbols C, U, or R depending upon the speci®c con- relatively short datasets. In these circumstances, esti- straints and the extent to which the coef®cients in Eq. mating too many regression coef®cients leads to over- (1) are adjustable. ®tting that causes a degradation in skill (Davis 1976). With no constraints on the coef®cients, Eqs. (1)±(2) Over®tting results in optimistically biased estimates describe the standard multimodel linear regression tech- of forecast skill when skill is assessed with the same nique that is described by Krishnamurti et al. (2000). data that are used to train the regression model. This bias is avoided by assessing skill in veri®cation data We denote this forecast by Rall, where the subscript all is used to indicate that all coef®cients are adjustable and that are independent of the training data. Cross vali- thus that all ensemble members may be weighted dif- dation (Michaelson 1987) is used here, as described ferently by the regression. The regression coef®cients subsequently, to increase the amount of veri®cation data. ai are found by solving M 1 1 linear equations M Over®tting can be reduced by imposing constraints a Cov(X , X ) 5 Cov(X , Y), k 5 1,...,M, on the coef®cients in the regression equation and there- O iikk i51 by effectively reducing the number of free parameters M that must be estimated from the data. One approach is a 1 aX 5 Y, to set some coef®cients to 0, thereby reducing the num- 0 O ii i51 ber of models providing forecasts that enter into Eq.
Details
-
File Typepdf
-
Upload Time-
-
Content LanguagesEnglish
-
Upload UserAnonymous/Not logged-in
-
File Pages7 Page
-
File Size-