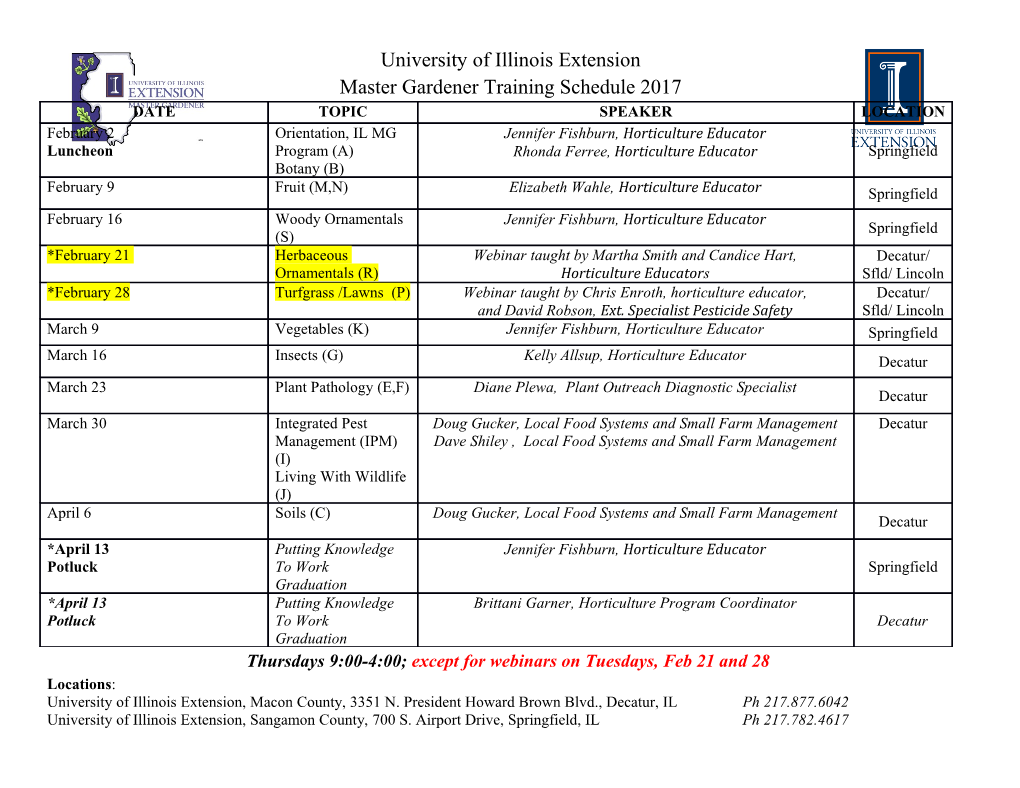
International Journal of Research and Scientific Innovation (IJRSI) | Volume V, Issue VII, July 2018 | ISSN 2321–2705 Stock Market Index Prediction Using Multilayer Perceptron and Long Short Term Memory Networks: A Case Study on BSE Sensex # R. Arjun Raj #Research Scholar, APJ Abdul Kalam Technological University, College of Engineering, Trivandru, Kerala, India Abstract- Stock market index is a statistical measure that portion of the overall market. A stock market index is the total quantifies the changes in a portfolio of stocks which represents a value obtained by the combination of different stocks or portion of the overall stock market. Prediction of stock market several other investment instruments altogether. It expresses has been a challenging task and of great interest for scholars as the total values with respect to a base value from a specific the very fact that stock market is a highly volatile in its period. Knowledge of Index values assist the investors track behaviour. Prediction of stock market is substantial in finance and is gathering more attention, due to the verity that if the changes in market values over longer periods of time. Stock direction of the market is predicted successfully the investors Market indices reflect an overall stock market and trace the may be effectively guided.” market‟s changes over time. Investors can track the changes in the value index over time .They can also utilize it as a “Deep Learning technique is a subfield of machine benchmark against which to make comparison of the returns learning which is concerned with algorithms necessitated by the function and structure of the brain called artificial neural from the portfolio. Stock market indices evaluate the value and networks. The most popular techniques are Multilayer merits of groups of different stocks. Perceptron Networks, Restricted Boltzmann Machines, As the stock market being chaotic, nonlinear, and Convolutional Neural Networks and Long Short-Term Memory dynamic in nature it is very difficult to understand because of Recurrent Neural Networks.” its volatility, hence it is of great importance for the investors “This work focuses on the task of predicting the stock to know its behaviour which would help for their effective market Index. The objective of the project work is to develop and investment in it. Artificial Neural Network (ANN) has the compare the performances of Multilayer Perceptron (MLP) and capability to identify the chaotic and nonlinear inter relation Long Short-Term Memory (LSTM) Networks in forecasting within the input data set without a priori presumption of stock market indices. Recent ten years historical data of Bombay Stock Exchange (BSE) Sensex from the Indian Stock market has knowledge of correlation between the input data and the been chosen for the experimental evaluation. The Adam output. Hence Artificial Neural Networks suits better than Optimizer is used for the training of deep neural networks. Root other models in forecasting the stock market returns [1]. mean square Error (RMSE) is used to compare the performance Many research work attempts have been conducted of the prediction models. As seen from the results, the prediction is fairly accurate in both cases and MLP has outperformed on stock market field to extract and identify some useful LSTM model, in predicting stock market indices. Neural patterns and forecast their variations and movements. The networks have proved to be a good technique, to forecast a values in Stock market in itself is a continuously evolving chaotic time series data like stock market index.” framework and is highly non-linear, perplexing, dynamicin nature.In this project, Multilayer Perceptron and Deep Keywords: Stock market index, Deep Learning, Multilayer Perceptron, Long Short-Term Memory Networks, Neural Recurrent Neural Network using LSTM (Long-Short Term networks. Memory) has been proposed for the reliable prediction results. 1) Problem definition: Multilayer Perceptron and Recurrent I. INTRODUCTION neural networks (RNN) are a very powerful technique for he task of prediction of stock market is very challenging analysing and processing the sequential data such as sound, Tand of great interest for researchers as the very fact that time series data or data written natural language. Long Short- stock market is a highly volatile in its behaviour. Prediction of Term Memory (LSTM) model is one of the most booming and stock market is substantial in finance and it is drawing more successful recurrent neural networks architectures. Only attention, due to the fact that the investors may be better limited works have been carried out for the prediction of guided if the direction and trend of the stock market is Indian Stock Market indices using Deep learning models such predicted successfully. as Multilayer Perceptron (MLP) and Long Short-Term Memory (LSTM) Networks. An index in stock market is a statistical measure which reflects the changes in a portfolio of stocks which represents a The stock market indices are highly volatile, chaotic and fluctuating, fluctuations affect the investor‟s calculations and www.rsisinternational.org Page 46 International Journal of Research and Scientific Innovation (IJRSI) | Volume V, Issue VII, July 2018 | ISSN 2321–2705 belief. Hence finding out more productive methods of stock Kai et al. through their work explained the power of Long market index forecasting is necessary for stock market Short Term Memory Networks in sequence learning for stock investors so that they can make more informed and accurate market prediction in China and it is understood from the investment decisions. results that normalization was very useful for enhancing accuracy [5]. Kaustubh et al. made a comparative analysis 2) Objectives of the work: The present work focuses on between Multilayer Perceptron (MLP) model and Long Short- predicting the future values of Bombay Stock Exchange Term Memory (LSTM) model leveraging the power of Sensex. The objectives of the project work are to develop and technical analysis and found that “Feed Forwards Multilayer compare the performances of Multilayer Perceptron (MLP) Perceptron perform superior to Long Short-Term Memory, at and Long Short-Term Memory (LSTM) Networks in predicting the short - term prices of a stock.Artificial Neural forecasting stock indices. networks have demonstrated to be a good technique, to predict 3) Research Methodology: Initially performed literature a chaotic and volatile framework like Stock Market[6]. survey to identify the various prediction models suitable for Mahanta et al. have selected Radial Basis Functional Network forecasting stock market indices, model parameters, deep (RBFN), Multilayer Perceptron model (MLP) and an learning models. Then collected data and analysed it. And optimized Radial Basis Functional neural network for developed deep learning models for stock market index predicting the closing prices of Sensex and Nifty using 11 prediction. The models considered in this study are Multilayer technical indicators. The proposed model optimized RBFgives Perceptron (MLP) and Long Short Term Memory (LSTM) better result as compare to MLP and RBF[7]. Networks. After that, the perfect combination of model Yoshua gave “the practical guide with recommendations parameters for the better prediction accuracy by parameter for some of the most commonly used hyper-parameters, in tuning was examined. The proposed models weretrained and particular in the context of learning algorithms based on back validated. Finally, compared the prediction accuracy of propagated gradient and gradient-based optimization The different variants of MLP and LSTM models based on the study also described the parameters used to effectively train performance measure RMSE. and debug large-scale and often deep multi-layer neural A. Literature Review networks models [8]. Several models have been utilized by analysts to predict Diederik and Jimmy introduced “Adam, an algorithm for the stock market values by using Artificial Neural Networks first-order gradient-based optimization of stochastic objective (ANN). The financial time series models which are functions based on adaptive estimates of lower-order represented by financial concepts and theories forms the moments. It is demonstrated that Adam works well in practice essence for predicting a series of data in the present century. and compares favourably to other stochastic optimization Still, these concepts cannot be directly applied to forecast the methods [9]. market values which are having external impact. The Bing et al. (2017) modelled a deep neural network enhancement of multilayer concept aids Artificial Neural ensemble to predict Chinese stock market index based on the Networks to be selected as a forecasting technique apart from input indices of recent days. A set of component networks are other methods[2]. Deep neural network is a machine-learning trained by historical data for this task, where Backpropagation paradigm for modelling complex nonlinear mappings between and Adam algorithm are used to train each network [10]. inputs and outputs, and the internal parameters are updated iteratively to make the given inputs fit with target outputs. From the literature review it is understood that the multilayer perceptron is one of the most commonly used The multilayer perceptron model is one of the important neural network topologies.Long Short Term Memory networks and commonly used prediction techniques. MLP is believed to are a kind of models that have the ability
Details
-
File Typepdf
-
Upload Time-
-
Content LanguagesEnglish
-
Upload UserAnonymous/Not logged-in
-
File Pages5 Page
-
File Size-