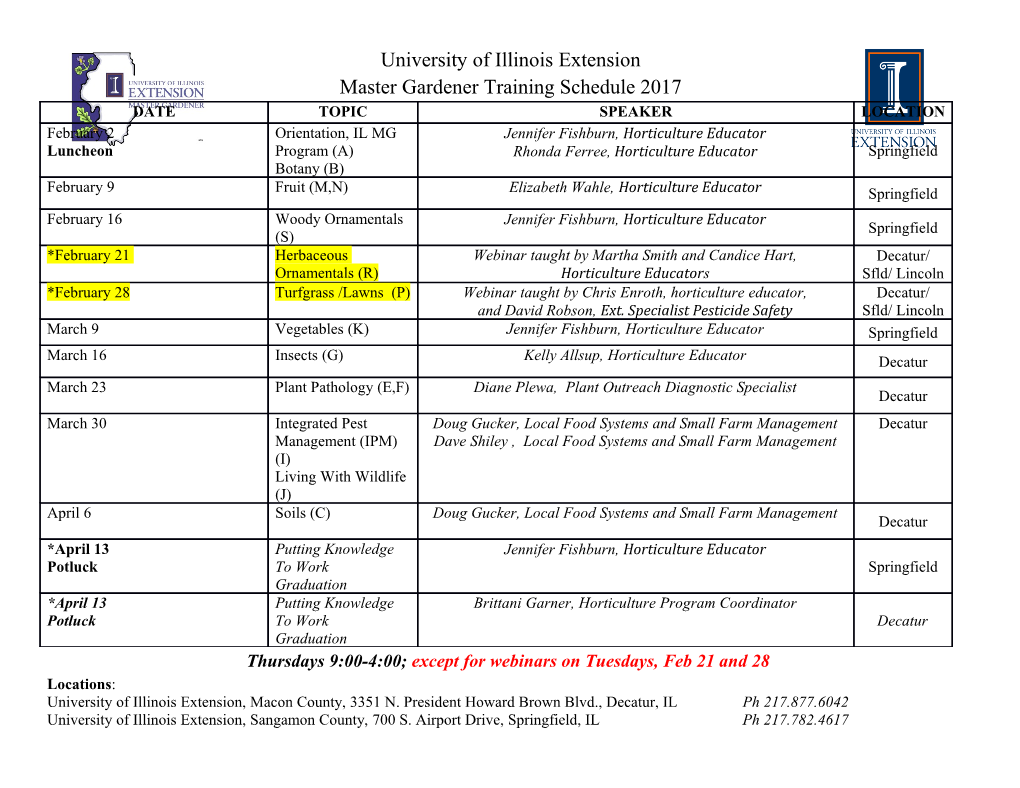
Opinion TRENDS in Cognitive Sciences Vol.8 No.10 October 2004 Universal Grammar, statistics or both? Charles D. Yang Department of Linguistics and Psychology, Yale University, 370 Temple Street 302, New Haven, CT 06511, USA Recent demonstrations of statistical learning in infants division of labor between endowment and learning: have reinvigorated the innateness versus learning plainly, things that are built in need not be learned, and debate in language acquisition. This article addresses things that can be garnered from experience need not these issues from both computational and developmen- be built in. tal perspectives. First, I argue that statistical learning The influential work of Saffran, Aslin and Newport [8] using transitional probabilities cannot reliably segment on statistical learning (SL) suggests that children are words when scaled to a realistic setting (e.g. child- powerful learners. Very young infants can exploit transi- directed English). To be successful, it must be con- tional probabilities between syllables for the task of word strained by knowledge of phonological structure. Then, segmentation, with only minimal exposure to an artificial turning to the bona fide theory of innateness – the language. Subsequent work has demonstrated SL in other Principles and Parameters framework – I argue that a full domains including artificial grammar, music and vision, explanation of children’s grammar development must as well as SL in other species [9–12]. Therefore, language abandon the domain-specific learning model of trigger- learning is possible as an alternative or addition to the ing, in favor of probabilistic learning mechanisms that innate endowment of linguistic knowledge [13]. might be domain-general but nevertheless operate in This article discusses the endowment versus learning the domain-specific space of syntactic parameters. debate, with special attention to both formal and deve- lopmental issues in child language acquisition. The first Two facts about language learning are indisputable. First, part argues that the SL of Saffran et al. cannot reliably only a human baby, but not her pet kitten, can learn a segment words when scaled to a realistic setting language. It is clear, then, that there must be some (e.g. child-directed English). Its application and effective- element in our biology that accounts for this unique ness must be constrained by knowledge of phonological ability. Chomsky’s Universal Grammar (UG), an innate structure. The second part turns to the bona fide theory of form of knowledge specific to language, is a concrete UG – the Principles and Parameters (P&P) framework theory of what this ability is. This position gains support [14,15]. It is argued that an adequate explanation of from formal learning theory [1–3], which sharpens the children’s grammar must abandon the domain-specific logical conclusion [4,5] that no (realistically efficient) learning models such as triggering [16,17] in favor of learning is possible without a priori restrictions on the probabilistic learning mechanisms that may well be learning space. Second, it is also clear that no matter how domain-general. much of a head start the child gains through UG, language is learned. Phonology, lexicon and grammar, although Statistics with UG governed by universal principles and constraints, do vary It has been suggested [8,18] that word segmentation from from language to language, and they must be learned on continuous speech might be achieved by using transitional the basis of linguistic experience. In other words, it is a probabilities (TP) between adjacent syllables A and B, truism that both endowment and learning contribute to where TP(A/B)ZP(AB)/P(A), where P(AB)Zfrequency language acquisition, the result of which is an extremely of B following A, and P(A)Ztotal frequency of A. Word sophisticated body of linguistic knowledge. Consequently, boundaries are postulated at ‘local minima’, where the both must be taken into account, explicitly, in a theory of TP is lower than its neighbors. For example, given language acquisition [6,7]. sufficient exposure to English, the learner might be able to establish that, in the four-syllable sequence Contributions of endowment and learning ‘prettybaby’, TP(pre/tty) and TP(ba/by) are both higher Controversies arise when it comes to the relative contri- than TP(tty/ba). Therefore, a word boundary between butions from innate knowledge and experience-based pretty and baby is correctly postulated. It is remarkable learning. Some researchers, in particular linguists, that 8-month-old infants can in fact extract three-syllable approach language acquisition by characterizing the words in the continuous speech of an artificial language scope and limits of innate principles of Universal Gram- from only two minutes of exposure [8]. Let us call this SL mar that govern the world’s languages. Others, in model using local minima, SLM. particular psychologists, tend to emphasize the role of experience and the child’s domain-general learning abil- Statistics does not refute UG ity. Such a disparity in research agenda stems from the To be effective, a learning algorithm – or any algorithm – Corresponding author: Charles D. Yang ([email protected]). must have an appropriate representation of the relevant (learning) data. We therefore need to be cautious about the www.sciencedirect.com 1364-6613/$ - see front matter Q 2004 Elsevier Ltd. All rights reserved. doi:10.1016/j.tics.2004.08.006 452 Opinion TRENDS in Cognitive Sciences Vol.8 No.10 October 2004 interpretation of the success of SLM, as Saffran et al. SL tradition; its success or failure may or may not carry themselves stress [19]. If anything, it seems that their over to other SL models. results [8] strengthen, rather than weaken, the case for The segmentation results using SLM are poor, even innate linguistic knowledge. assuming that the learner has already syllabified the A classic argument for innateness [4,5,20] comes from input perfectly. Precision is 41.6%, and recall is 23.3% the fact that syntactic operations are defined over specific (using the definitions in Box 1); that is, over half of the types of representations – constituents and phrases – but words extracted by the model are not actual words, and not over, say, linear strings of words, or numerous other close to 80% of actual words are not extracted. This is logical possibilities. Although infants seem to keep track of unsurprising, however. In order for SLM to be usable, a statistical information, any conclusion drawn from such TP at an actual word boundary must be lower than its findings must presuppose that children know what kind neighbors. Obviously, this condition cannot be met if the of statistical information to keep track of. After all, an input is a sequence of monosyllabic words, for which a infinite range of statistical correlations exists: for space must be postulated for every syllable; there are no example, What is the probability of a syllable rhyming local minima to speak of. Whereas the pseudowords with the next? What is the probability of two adjacent in Saffran et al. [8] are uniformly three-syllables long, vowels being both nasal? The fact that infants can use much of child-directed English consists of sequences of SLM at all entails that, at minimum, they know the monosyllabic words: on average, a monosyllabic word is relevant unit of information over which correlative followed by another monosyllabic word 85% of time. As statistics is gathered; in this case, it is the syllable, rather long as this is the case, SLM cannot work in principle. than segments, or front vowels, or labial consonants. Yet this remarkable ability of infants to use SLM could A host of questions then arises. First, how do infants still be effective for word segmentation. It must be con- know to pay attention to syllables? It is at least plausible strained – like any learning algorithm, however powerful that the primacy of syllables as the basic unit of speech is – as suggested by formal learning theories [1–3]. Its innately available, as suggested by speech perception performance improves dramatically if the learner is studies in neonates [21]. Second, where do the syllables equipped with even a small amount of prior knowledge come from? Although the experiments of Saffran et al. [8] about phonological structures. To be specific, we assume, used uniformly consonant–vowel (CV) syllables in an uncontroversially, that each word can have only one artificial language, real-world languages, including primary stress. (This would not work for a small and English, make use of a far more diverse range of syllabic closed set of functional words, however.) If the learner types. Third, syllabification of speech is far from trivial, knows this, then ‘bigbadwolf ’ breaks into three words for involving both innate knowledge of phonological struc- free. The learner turns to SLM only when stress tures as well as discovering language-specific instanti- information fails to establish word boundary; that is, it ations [22]. All these problems must be resolved before limits the search for local minima in the window between SLM can take place. two syllables that both bear primary stress, for example, between the two a’s in the sequence ‘languageacquisition’. This is plausible given that 7.5-month-old infants are Statistics requires UG sensitive to strong/weak patterns (as in fallen) of prosody To give a precise evaluation of SLM in a realistic setting, [22]. Once such a structural principle is built in, the we constructed a series of computational models tested on stress-delimited SLM can achieve precision of 73.5% and speech spoken to children (‘child-directed English’) [23,24] recall of 71.2%, which compare favorably to the best (see Box 1). It must be noted that our evaluation focuses performance previously reported in the literature [26].
Details
-
File Typepdf
-
Upload Time-
-
Content LanguagesEnglish
-
Upload UserAnonymous/Not logged-in
-
File Pages6 Page
-
File Size-