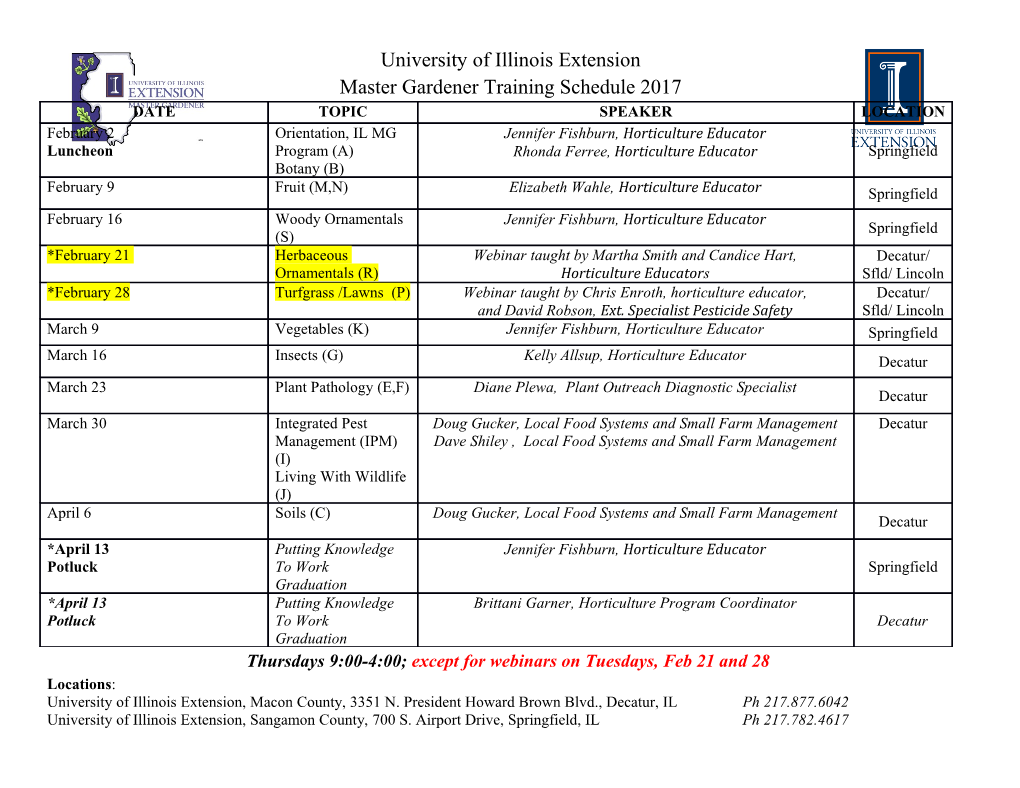
Explained Variation and Explained Randomness for Proportional Hazard Models John O'Quigley and Ronghui Xu Lukas Butikofer¨ Biostatistics Journal Club Zurich, 15 May 2013 Introduction Expl. Variation Expl. Randomness Based on Fisher Info Properties/Interpretation Extensions Illustrations Outline 1. Introduction 2. Explained Variation 3. Explained Randomness 4. Measure of Predictability based on Fisher Information 5. Properties and Interpretation 6. Extensions 7. Illustrations Lukas Butikofer¨ 2/ 30 Introduction Expl. Variation Expl. Randomness Based on Fisher Info Properties/Interpretation Extensions Illustrations Goal provide a quantifying measure of a proportional hazard model's predictability ! how strong are predictive effects? statements about: - how much of survival is explained by treatment? - changes in predictability by adding a prognostic factor to a model compare non-nested models Lukas Butikofer¨ 3/ 30 Introduction Expl. Variation Expl. Randomness Based on Fisher Info Properties/Interpretation Extensions Illustrations Predictability Measures regression coefficients: - depend on scale of covariates explained variation R2: - only straightforward for linear models - generalizations for proportional hazard models depend on distribution of the covariate - not invariant to transformations of response variable deviance: likelihood has to be fully specified nested models required Lukas Butikofer¨ 4/ 30 Introduction Expl. Variation Expl. Randomness Based on Fisher Info Properties/Interpretation Extensions Illustrations Desired Characteristics of Predictability Measures direct relation to predictability of survival ranks ! perfect prediction: 1; absence of effect: 0 ! intermediate values should be interpretable invariance to monotonic transformation of time (as regression coefficient in Cox model) invariance to linear transformation of covariates unaffected by independent censoring accommodation of time-dependent covariates interpretation as explained variation or randomness Lukas Butikofer¨ 5/ 30 Introduction Expl. Variation Expl. Randomness Based on Fisher Info Properties/Interpretation Extensions Illustrations Model and Notation T : potential failure time, C : potential censoring time ! time observed: X = min(T ; C ) Z (t): possibly time-dependent covariates i = 1; 2; :::; n subjects with (Ti ; Ci ; Zi ) δ = I (T ≤ C ) ! 1: failure, 0: censoring Y (t) = I (X ≥ t) ! 1: at risk at time t proportional hazard: h(tjZ (t)) = h0(t) exp(βZ (t)) Lukas Butikofer¨ 6/ 30 Introduction Expl. Variation Expl. Randomness Based on Fisher Info Properties/Interpretation Extensions Illustrations Cox Partial Likelihood Conditional probability of subject i chosen to fail given all individuals at risk and that one failure occurs: Yi (t) exp(βZi (t)) πi (β; t) = n P [Yj (t) exp(βZj (t)] j =1 Cox partial likelihood [Cox, 1972]: n Y Y δi Lp(β) = πi (β; t) = πi (β; t) failures i=1 2 n 3 X X lp(β) = 4log(Yi (t) exp(βZi (t))) − log [Yj (t) exp(βZj (t))]5 failures j =1 Lukas Butikofer¨ 7/ 30 Introduction Expl. Variation Expl. Randomness Based on Fisher Info Properties/Interpretation Extensions Illustrations Cox Scores 2 n 3 P [Zj (t)Yj (t) exp(βZj (t)] 6 7 X 6 j =1 7 Scores: S(β) = Zi (t) − n 6 P 7 failures 4 [Yj (t) exp(βZj (t)] 5 j =1 X = [Zi (t) − Eβ(Z jt)] failures n P n [Zj (t)Yj (t) exp(βZj (t)] X j =1 since: Eβ(Z jt) = Zj (t)πj (β; t) = n P j =1 [Yj (t) exp(βZj (t)] j =1 Lukas Butikofer¨ 8/ 30 Introduction Expl. Variation Expl. Randomness Based on Fisher Info Properties/Interpretation Extensions Illustrations Residuals ^ X MLE of β: S(β) = 0 = [Zi (t) − Eβ^(Z jt)] failures ^ Schoenfeld residuals: ri (β) = Zi (Xi ) − Eβ^(Z jXi ) for δi = 1 [Schoenfeld, 1982] n X X Note that: S(β) = [ri (β)] = [δi ri (β)] failures i=1 sum of residuals is set to 0 to estimate unknown parameter ! analogous to ordinary regression Lukas Butikofer¨ 9/ 30 Introduction Expl. Variation Expl. Randomness Based on Fisher Info Properties/Interpretation Extensions Illustrations Fisher Information n n X X @ri (β) J (β) = E[S 2(β)] = δ E[r 2(β)] = − δ E i i i @(β) i=1 i=1 residual sum of squares (SSres): estimate of Fisher Info SSres is used to study predictability: ! explained variation linear model: n P 2 (Yi −Y^i ) 2 i=1 SSres R = 1 − n = 1 − P 2 SStotal (Yi −Y¯ ) i=1 ! based on a measure of information, interpretation as explained randomness Lukas Butikofer¨ 10/ 30 Introduction Expl. Variation Expl. Randomness Based on Fisher Info Properties/Interpretation Extensions Illustrations Explained Variation versus Explained Randomness explained variation Ω2/R2: proportion to which a model accounts for the variation of a given data set proportion of total variation explained by conditioning on a model (or a random variable) explained randomness ρ2: information gain of one model over the other distance between distributions interpretable as explained variation in some cases Lukas Butikofer¨ 11/ 30 Introduction Expl. Variation Expl. Randomness Based on Fisher Info Properties/Interpretation Extensions Illustrations Explained Variation consider the random pair (T ; Z ): Var(T ) = E[Var(T jZ )] + Var[E(T jZ )] Var[E(T jZ )]: signal, explained by conditioning on Z E[Var(T jZ )]: noise, residual variance explained variation: amount of variation in T explained by conditioning on Z Var[E(T jZ )] E[Var(T jZ )] Ω2 (Z ) = = 1 − T Var(T ) Var(T ) Lukas Butikofer¨ 12/ 30 Introduction Expl. Variation Expl. Randomness Based on Fisher Info Properties/Interpretation Extensions Illustrations Explained Variation Idea: the more the variance can be reduced by the model, the greater is the model's predictability Problem: more complicated if the variables are linked by a regression model ! no practical interpretation Cox: 2 - upper bound on ΩT (Z ) that depends heavily on distribution of covariate Z - e.g. binary covariates with βA = 2 and βB = 20 2 2 ! ΩT (A) = 0:25 and ΩT (B) = 0:16 2 - consider ΩZ (T ) Lukas Butikofer¨ 13/ 30 Introduction Expl. Variation Expl. Randomness Based on Fisher Info Properties/Interpretation Extensions Illustrations Explained Randomness Quantifies how far away the null model is from the model under consideration by measuring the distance between the distributions measures of distanced between distributions: Fisher Information or Kullback-Leibler Information more general than explained variation coincides with explained variation in special cases: 2 multivariate normal, Cox regression (if ΩZ (T ) is used) Lukas Butikofer¨ 14/ 30 Introduction Expl. Variation Expl. Randomness Based on Fisher Info Properties/Interpretation Extensions Illustrations Explained Randomness based on Kullback-Leibler Info (1) Kullback-Leibler discrepancy: pX (x); pY (y): probability density functions D(pX jjpY ) = E[log(pX (X )] − E[log(pY (X )] distance between null model (β = 0, no regression effect) and model of interest: Γ(β) = 2[I (β) − I (0)] explained randomness: ρ2(β) = 1 − exp(−Γ(β)) ! measure for predictability, not depending on scale Lukas Butikofer¨ 15/ 30 Introduction Expl. Variation Expl. Randomness Based on Fisher Info Properties/Interpretation Extensions Illustrations Explained Randomness based on Kullback-Leibler Info (2) I1(θ) = x log[f (tjz; θ)]f (tjz; β)dt dG(z) ZT I2(θ) = x log[g(zjt; θ)]g(zjt; β)dz dF (t) TZ rank invariant to monotonic transformations on time estimation of g(zjt; β) by πi (β;^ t) estimation of F (t) by Kaplan-Meier ! W (Xi ) k n ^ ! X X πi (β; Xj ) Γ^ (β^) = 2 W (X ) π (β;^ X ) log 2 j i j π (0; X ) j =1 i=1 i j j = 1,...,k: failures !T ; i = 1,...,n: subjects, !Z Lukas Butikofer¨ 16/ 30 Introduction Expl. Variation Expl. Randomness Based on Fisher Info Properties/Interpretation Extensions Illustrations Explained Variation/Randomness based on Fisher Info (I) Schoenfeld residuals: ri (β) = Zi (Xi ) − Eβ(Z jXi ) for δi = 1 n P 2 residual sum of squares: I(β) = δi ri (β) i=1 n P 2 total sum of squares: I(0) = δi ri (0) i=1 2 I(β) explained variation: R (β) = 1 − I(0) Normal model: coincides with coefficient of correlation, percentage of explained variation Lukas Butikofer¨ 17/ 30 Introduction Expl. Variation Expl. Randomness Based on Fisher Info Properties/Interpretation Extensions Illustrations Explained Variation/Randomness based on Fisher Info (II) multivariate case: - prognostic index: η(t) = βZ (t) - individuals with different Z but same η have same survival probability - univariate: Z equivalent to η ! residuals of Z can be used - multivariate: residuals of η instead of Z n n X 2 X 2 I(β) = δi [βri (β)] and I(0) = δi [βri (0)] i=1 i=1 Lukas Butikofer¨ 18/ 30 Introduction Expl. Variation Expl. Randomness Based on Fisher Info Properties/Interpretation Extensions Illustrations Correction for Censoring R2 asymptotically dependent on censoring ! correction weight squared residuals by estimate of F (t) ! Kaplan-Meier weights: S^(t) W (t) = n relative jumps of the KM-curve P Yi (t) i=1 weighted R2: n P 2 δi W (Xi )ri (β) 2 I(β) i=1 R (β) = 1 − = 1 − n I(0) P 2 δi W (Xi )ri (0) i=1 unaffected by independent censoring, coincides with previous definition in the absence of censoring Lukas Butikofer¨ 19/ 30 Introduction Expl. Variation Expl. Randomness Based on Fisher Info Properties/Interpretation Extensions Illustrations Population Parameter in practice: R2(β^) instead of R2(β) with β^: partial likelihood estimate population parameter of R2(β^): R 2 2 Eβf[Z (t) − Eβ(Z (t)jt)] jtg dF (t) Ω (β) = 1 − R 2 Eβf[Z (t) − E0(Z (t)jt)] jtg dF (t) F : failure time distribution function; F = 1 − S if Z time-invariant: Ω2(β) = explained variation Ω2(β) depends only weakly on covariate distribution Lukas Butikofer¨ 20/ 30 Introduction Expl. Variation Expl. Randomness Based on Fisher Info Properties/Interpretation Extensions Illustrations Properties of R2 R2(0) = 0 R2(β^) ≤ 1 R2(β^) is invariant under linear transformation of Z and monotonically increasing transformations of T R2(β^) consistently estimates Ω2(β) R2(β^) is asymptotically normal R2(β^) can be negative (best fitting model provides poorer fit than null model) Lukas Butikofer¨ 21/ 30 Introduction Expl.
Details
-
File Typepdf
-
Upload Time-
-
Content LanguagesEnglish
-
Upload UserAnonymous/Not logged-in
-
File Pages30 Page
-
File Size-