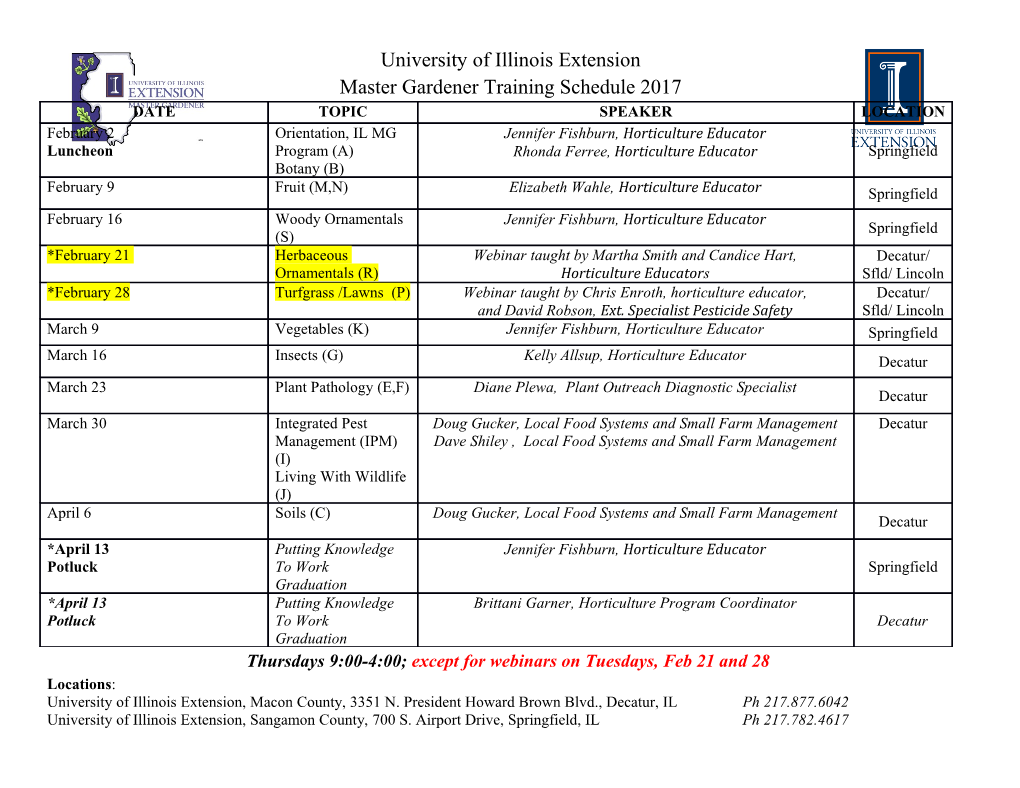
The Thirty-Third AAAI Conference on Artificial Intelligence (AAAI-19) Simulation-Based Approach to Efficient Commonsense Reasoning in Very Large Knowledge Bases Abhishek Sharma , Keith M. Goolsbey Cycorp Inc., 7718 Wood Hollow Drive, Suite 250, Austin, TX 78731 [email protected], [email protected] Abstract paths. Therefore, to make the search more efficient in such Cognitive systems must reason with large bodies of general a KBS, an inference engine is expected to assess the utility knowledge to perform complex tasks in the real world. of further expanding each incomplete path. A naïve order- However, due to the intractability of reasoning in large, ex- ing algorithm can cause unproductive backtracking. To pressive knowledge bases (KBs), many AI systems have solve this problem, researchers have used two types of limited reasoning capabilities. Successful cognitive systems have used a variety of machine learning and axiom selection search control knowledge: (i) Axiom/premise selection methods to improve inference. In this paper, we describe a heuristics: These heuristics attempt to find the small num- search heuristic that uses a Monte-Carlo simulation tech- ber of axioms that are the most relevant for answering a set nique to choose inference steps. We test the efficacy of this of queries, and (ii) Certain researchers have worked on approach on a very large and expressive KB, Cyc. Experi- ordering heuristics for improving the order of rule and mental results on hundreds of queries show that this method is highly effective in reducing inference time and improving node expansions. question-answering (Q/A) performance. In the current work, we describe a simulation-based ap- proach for learning an ordering heuristic for controlling search in large knowledge-based systems (KBS). The key Introduction idea is to simulate several thousand paths from a node. Deductive reasoning is an important component of many New nodes are added to a search tree, and each node con- cognitive systems. Modern cognitive systems need large tains a value that predicts the expected number of answers bodies of general knowledge to perform complex tasks from expanding the tree from that node. The search tree (Lenat & Feigenbaum 1991, Forbus et al 2007). However, expansion guides simulations along promising paths, by efficient reasoning systems can be built only for small-to- selecting the nodes that have the highest potential values. medium sized knowledge bases (KBs). Very large The algorithm produces a highly selective and asymmetric knowledge bases contain millions of rules and facts about search tree that quickly identifies good axiom sequences the world in highly expressive languages. Due to the in- for queries. Moreover, the evaluation function is not hand- tractability of reasoning in such systems, even simple que- crafted: It depends solely on the outcomes of the simulated ries are timed-out after several minutes. Therefore, re- paths. This approach has the characteristics of a statistical searchers believe that resolution-based theorem provers are anytime algorithm: The quality of evaluation function im- overwhelmed when they are expected to work on large proves with additional simulations. The evaluation func- expressive KBs (Hoder & Voronkov 2011). tion is used to order nodes in a search. Experimental results The goal query in knowledge-based systems (KBS) is show that: (i) this approach helps in significantly reducing typically provable from a small number of ground atomic inference time and, (ii) by guiding the search towards more formulas (GAFs) and rules. However, unoptimized infer- promising parts of the tree, this approach improves the ence engines can find it difficult to distinguish between a question-answering performance in time-constrained cog- small set of relevant rules and the millions of irrelevant nitive systems. ones. Hundreds of thousands of axioms that are irrelevant This paper is organized as follows: We start by discuss- for the query can inundate the reasoner with millions of ing relevant previous work. Our approach to using simula- tions to learn a search heuristic is explained next. We con- clude after analyzing the experimental results. Copyright © 2019, Association for the Advancement of Artificial Intelli- gence (www.aaai.org). All rights reserved. 1360 Related Work specific collections of which it is an instance. This set is referred to as MostSpecificCollections (e). Learning of search control knowledge plays an important Reasoning with Cyc representation language is difficult role in the optimization of KBS for at least two reasons: due to the size of the KB and the expressiveness of the First, the inference algorithms of KBS (e.g., backward language. The Cyc representation language uses full first- chaining, tableaux algorithms in description logic (DL)), order logic with higher-order extensions. Some examples typically represent their search space as a graph. Hundreds of highly expressive features of its language include: (a) of rules can apply to each node in a large KBS, and re- Cyc has more than 2000 rules with quantification over searchers have shown that the specific order of node and predicates, (b) it has 267 relations with variable arities, and rule expansion can have a significant effect on efficiency (c) Although first-order logic with three variables is unde- (Tsarkov & Horrocks 2005). Further still, first-order logic cidable (Tsarkov et al. 2004), Cyc ahs several thousand (FOL) theorem provers have been used as tools for such rules with more than three variables. The number of rules reasoning with very expressive languages (e.g., OWL DL, in Cyc with three, four and five variables are 48160, 23813 the Semantic Web Rule Language (SWRL)), where the and 14014 respectively. Moreover, 29716 rules have more language does not correspond to any decidable fragment of than five variables. In its default inference mode, the Cyc FOL, or where reasoning with the language is beyond the inference engine uses the following types of rules/facts scope of existing DL algorithms (Tsarkov et al. 2004, Hor- during inference: (i) 28,429 role inclusion axioms; (ii) rocks & Voronkov 2006). Researchers have also examined 3,623 inverse role axioms, (iii) 494,405 concepts and 1.1 the use of machine learning techniques to identify the best million concept inclusion axioms; (iv) 814 transitive roles; heuristics for problems (Bridge et al. 2014). There has (v) 120,547 complex role inclusion axioms; (vi) 77,170 been work as well on the premise selection algorithms other axioms; (vii) 35,528 binary roles and 10,508 roles (Hoder & Voronkov 2011, Sharma & Forbus 2013, Meng with arities greater than two. The KB has 27.3 million as- & Paulson 2009, Kaliszyk et al. 2015, Kaliszyk & Urban sertions and 1.14 million individuals. 2015, Alama et al. 2014). In contrast, we focus on design- To efficiency search in such a large KBS, inference en- ing ordering heuristics that will enable the system to work gines often use control strategies. They define: (i) set of with all axioms. In (Tsarkov & Horrocks 2005), the au- support, i.e. the set of important facts about the problem.; thors proposed certain rule-ordering heuristics, and expan- and (ii) the set of usable axioms, i.e. the set of all axioms sion ordering heuristics (e.g., a descending order of fre- outside the set of support. At every inference step, the in- quency of usage of symbols). In contrast, we believe that ference engine has to select an element from the set of usa- rule-ordering heuristics should be based on the search ble axioms and resolve it with an element of the set of sup- state. In other recent work (Sharma, Witbrock & Goolsbey port. To perform efficient search, a heuristic control strate- 2016, Sharma & Goolsbey 2017), researchers have used gy measures the “weight” of each clause in the set of sup- different machine learning techniques to improve the effi- port, picks the “best” clause, and adds to the set of support ciency of theorem provers, ore approach is different from the immediate consequences of resolving it with the ele- all aforementioned research because none have shown how ments of the usable list (Russell & Norvig 2003). Cyc uses Monte Carlo tree search/UCT-based approach can be used a set of heuristic modules to identify the best clause from to improve reasoning in a very large and expressive KBS. the set of support. If S is the set of all states, and A is the The work in other fields (Chaudhuri 1998, Hutter et al. set of actions (i.e. inference steps), then a heuristic module 2014, Brewka et al. 2011) is also less relevant because it is a tuple h : (w ., f ) , where f is a function f : S ×A R, does not address the complexity of reasoning needed for i i i i i which assesses the quality of an inference step, and w is large and expressive KBS. i the weight of h . The net score of an inference step is ∑ i Background wifi(s, a) and the inference step with the highest score is selected. Cyc uses several heuristics including the success rates of rules, decision trees (Sharma, Witbrock and We assume familiarity with the Cyc representation lan- Goolsbey 2016), regression-based models (Sharma, Wit- guage (Lenat & Guha 1990). In Cyc, concepts are repre- brock, and Goolsbey 2016) and a large database of useful sented as collections. For example, “Cat” is the set of cats rule sequences (Sharma & Goolsbey 2017). We can define and only cats. Concept hierarchies are represented by the a policy that uses these heuristics: “genls” relation. For example, (genls Telephone Artifact- Π (s)= argmax ∑ w f (s, a) …(1) Communication) holds. The “isa” relation is used to link BASELINE a i i things to any kind of collections of which they are instanc- In (1), we use all heuristics mentioned above to calculate es.
Details
-
File Typepdf
-
Upload Time-
-
Content LanguagesEnglish
-
Upload UserAnonymous/Not logged-in
-
File Pages8 Page
-
File Size-