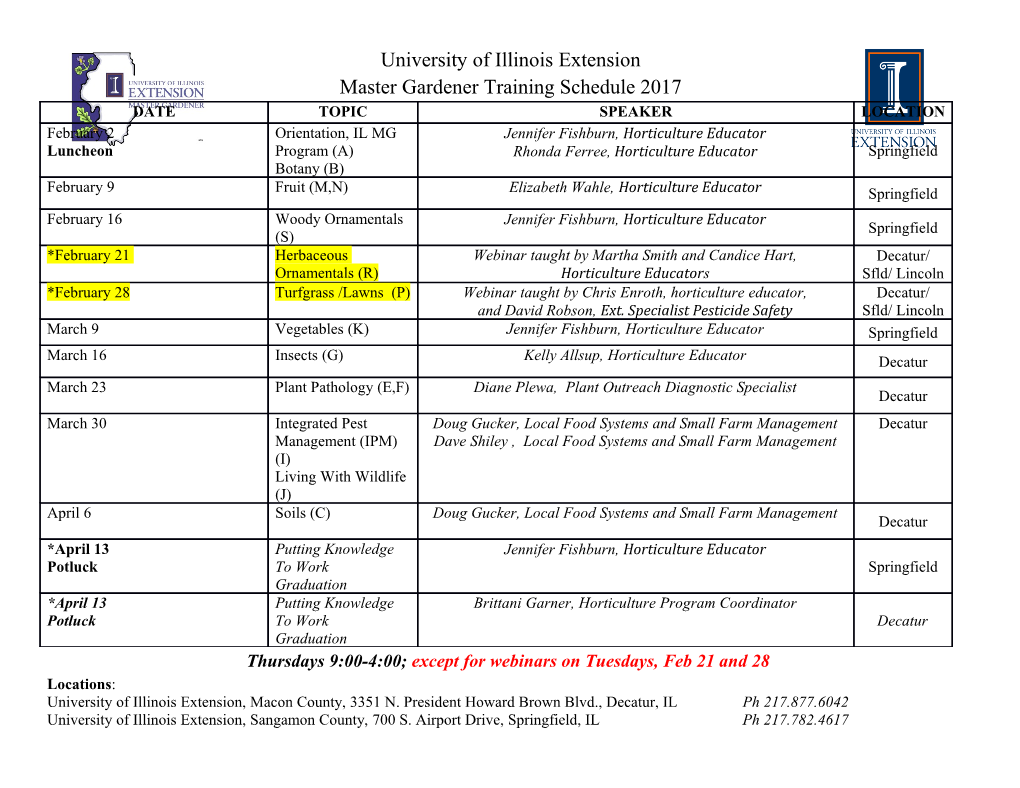
GPUs: Hype, Reality, and Future 30/07/2012 David Black-Schaffer Uppsala University / Department of Informaon Technology 30/07/2012 | 2 Uppsala Programming for Multicore Architectures Research Center Today 1. The hype GPUs: The Hype, The Reality, 2. What makes a GPU a GPU? and The Future 3. Why are GPUs scaling so well? 4. What are the problems? David Black-Schaffer 5. What’s the Future? Assistant Professor, Department of Informaon Technology Uppsala University David Black-Schaffer Uppsala University / Department of Informaon Technology 30/07/2012 | 3 David Black-Schaffer Uppsala University / Department of Informaon Technology 30/07/2012 | 4 How Good are GPUs? THE HYPE 100x 3x David Black-Schaffer Uppsala University / Department of Informaon Technology 30/07/2012 | 5 David Black-Schaffer Uppsala University / Department of Informaon Technology 30/07/2012 | 6 Real World SoXware GPUs for Linear Algebra • Press release 10 Nov 2011: 5 CPUs = – “NVIDIA today announced that four leading applicaons… 75% of 1 GPU have added support for mulKple GPU acceleraon, enabling them to cut simulaon mes from days to hours.” • GROMACS – 2-3x overall – Implicit solvers 10x, PME simulaons 1x • LAMPS – 2-8x for double precision – Up to 15x for mixed • QMCPACK – 3x 2x is AWESOME! Most research claims 5-10%. StarPU for MAGMA © 2012 David Black-Schaffer 1 GPUs: Hype, Reality, and Future 30/07/2012 David Black-Schaffer Uppsala University / Department of Informaon Technology 30/07/2012 | 7 David Black-Schaffer Uppsala University / Department of Informaon Technology 30/07/2012 | 8 GPUs by the Numbers Efficiency (Peak and TDP) (DP, peak) But how close to peak GPUs are enormously 5 4 do you really get? more efficient designs 400 791 for doing FLOPs 675 4 192 3 300 176 3 Intel 32nm vs. 40nm 36% smaller per transistor 2 200 250 2 244 GFLOP/W 3.0 1 100 Normalized to Intel 3960X 130 172 51 3.3 2.3 2.4 1 FLOP/cycle/B Transistors 1.5 0.9 0 0 0 WaEs GFLOP Bandwidth GHz Transistors Intel 3960X GTX 580 AMD 6970 Intel 3960X Nvidia GTX 580 AMD 6970 Energy Efficiency Design Efficiency David Black-Schaffer Uppsala University / Department of Informaon Technology 30/07/2012 | 9 David Black-Schaffer Uppsala University / Department of Informaon Technology 30/07/2012 | 10 Efficiency in PerspecKve Intel’s Response Energy Efficiency • Larabee Nvidia4 says that – Manycore x86-“light” to compete with Nvidia/AMD in graphics and compute ARM + Laptop GPU LINPACK: Green 500 #1 – Didn’t work out so well (despite huge fab advantages — graphics is hard) 7.5GFLOPS/W Blue Gene/Q LINPACK: • Reposi/oned it as an HPC co-processor 3 Green 500 #6 – Knight’s Corner GPU/CPU – 1TF double precision in a huge (expensive) single 22nm chip – At 300W this would beat Nvidia’s peak efficiency today (40nm) 2 GFLOP/W 1 Peak Peak Peak Actual Actual 0 Intel 3960X GTX 580 AMD 6970 Blue Gene/Q DEGIMA Cluster David Black-Schaffer Uppsala University / Department of Informaon Technology 30/07/2012 | 11 David Black-Schaffer Uppsala University / Department of Informaon Technology 30/07/2012 | 12 Show Me the Money 4 9.4 3 2 BTW — Intel’s Ivy Bridge integrated graphics is about to eat most of the GPU market. 1 Revenue in Billion USD WHAT MAKES A GPU A GPU? <50% of this is 0 GPU in HPC AMD Q3 Nvidia Q3 Intel Q3 Professional Graphics Embedded Processors © 2012 David Black-Schaffer 2 GPUs: Hype, Reality, and Future 30/07/2012 David Black-Schaffer Uppsala University / Department of Informaon Technology 30/07/2012 | 13 David Black-Schaffer Uppsala University / Department of Informaon Technology 30/07/2012 | 14 GPU CharacterisKcs GPU Innovaons • Architecture • Programming/Interface • – Data parallel processing – Data parallel kernels SMT (for latency hiding) – Hardware thread scheduling – Throughput-focused – Massive numbers of threads – High memory bandwidth – Limited synchronizaon – Programming SMT is far easier than SIMD – Graphics rasterizaon units – Limited OS interacKon • SIMT (thread groups) – Limited caches – AmorKze scheduling/control/data access (with texture filtering hardware) – Warps, wavefronts, work-groups, gangs • Memory systems op/mized for graphics – Special storage formats – Texture filtering in the memory system – Bank opKmizaons across threads • Limited synchronizaon – Improves hardware scalability (They didn’t invent any of these, but they made them successful.) David Black-Schaffer Uppsala University / Department of Informaon Technology 30/07/2012 | 15 David Black-Schaffer Uppsala University / Department of Informaon Technology 30/07/2012 | 16 Lots of Room to Grow the Hardware Simple cores Simple control But, they do have a lot… – Short pipelines – Limited context switching – TLBs for VM – No branch predicKon – Limited processes – I-caches – In-order – (But do have to schedule – Complex hardware thread Simple memory system 1000s of threads…) schedulers – Limited caches* Lack of OS-level issues – No coherence – Memory allocaon Trading off simplicity – Split address space* – PreempKon for features and programmability. 1600 200 1200 400 WHY ARE GPUS SCALING SO WELL? 1200 150 900 300 800 100 600 200 FLOP/cycle GFLOPs (SP) 400 50 Bandwidth (GB/s) 300 100 FLOP/cycle/B Transistors 0 0 0 0 G80 GT200 GF100 G80 GT200 GF100 GFLOP (SP) Bandwidth Architecture Efficiency Design Efficiency David Black-Schaffer Uppsala University / Department of Informaon Technology 30/07/2012 | 17 David Black-Schaffer Uppsala University / Department of Informaon Technology 30/07/2012 | 18 “Nice” Programming Model Why are GPUs Scaling So Well? void kernel calcSin(global float *data) { • All GPU programs have: int id = get_global_id(0); • Room in the hardware design data[id] = sin(data[id]); – Explicit parallelism } • Scalable soXware – Hierarchical structure Synchronization OK.! – Restricted synchronizaon – Data locality They’re not burdened with 30 years of cru6 and No Synchronization.! • Inherent in graphics legacy code… • Enforced in compute by performance …lucky them. – Latency tolerance Expensive • Easy to scale! Cheap © 2012 David Black-Schaffer 3 GPUs: Hype, Reality, and Future 30/07/2012 David Black-Schaffer Uppsala University / Department of Informaon Technology 30/07/2012 | 19 David Black-Schaffer Uppsala University / Department of Informaon Technology 30/07/2012 | 20 Amdahl’s Law • Always have serial code – GPU single threaded performance is terrible • Soluon: Heterogeneity – A few “fat” latency-opmized cores (CPUs) – Many “thin” throughput-opmized cores (GPUs) – Plus hard-coded accelerators • Nvidia Project Denver • ARM for latency 100 99% Parallel (91x) WHERE ARE THE PROBLEMS? • GPU for throughput • AMD Fusion 10 90% Parallel (10x) • x86 for latency 75% Parallel (4x) • GPU for throughput Maximum Speedup • 1 Intel MIC 1 2 4 8 16 32 64 128 256 512 1024 • x86 for latency Number of Cores • X86-“light” for throughput Limits of Amdahl’s Law David Black-Schaffer Uppsala University / Department of Informaon Technology 30/07/2012 | 21 David Black-Schaffer Uppsala University / Department of Informaon Technology 30/07/2012 | 22 It’s an Accelerator… Legacy Code • Moving data to the GPU is slow… • Code lives forever • Moving data from the GPU is slow… – Amdahl: Even opKmizing the 90% of hot code limits speedup to 10x – • Moving data to/from the GPU is really slow. Many won’t invest in proprietary technology • Programming models are immature – CUDA mature low-level Nvidia, PGI • Limited data storage – OpenCL immature low-level Nvidia, AMD, Intel, ARM, Altera, Apple • Limited interacKon with OS – OpenHMPP mature high-level CAPS, PathScale – OpenACC immature high-level CAPS, Nvidia, Cray, PGI 192GB/s GPU GB/s CPU 51GB/s GRAM 5 DRAM Nvidia GTX 580 Intel 3960X 1.5GB 16GB 529mm2 PCIe 3.0 435mm2 10GB/s David Black-Schaffer Uppsala University / Department of Informaon Technology 30/07/2012 | 23 David Black-Schaffer Uppsala University / Department of Informaon Technology 30/07/2012 | 24 Making PorKng Easier Code that Doesn’t Look Like Graphics • If it’s not painfully data-parallel you have to redesign your algorithm – Example: scan-based techniques for zero counKng in JPEG – Why? It’s only 64 entries! High(er)-level Libraries • Single-threaded performance is terrible. Need to parallelize. languages are great are easiest • Overhead of transferring data to CPU is too high. • If it’s not accessing memory well you have to re-order your algorithm – Example: DCT in JPEG • Need to make sure your access to local memory has no bank conflicts across threads. • Libraries star/ng to help – Lack of composability – Example: Combining linear algebra operaons to keep data on the device • Most code is not purely data-parallel – Very expensive to synchronize with the CPU (data transfer) – No effecKve support for task-based parallelism – No ability to launch kernels from within kernels © 2012 David Black-Schaffer 4 GPUs: Hype, Reality, and Future 30/07/2012 David Black-Schaffer Uppsala University / Department of Informaon Technology 30/07/2012 | 25 David Black-Schaffer Uppsala University / Department of Informaon Technology 30/07/2012 | 26 Corollary: What are GPUs Good For? • Data parallel code – Lots of threads – Easy to express parallelism (no SIMD nasKness) • High arithme/c intensity and simple control flow – Lots of FPUs – No branch predictors • High reuse of limited data sets – Very high bandwidth to memory (1-6GB) – Extremely high bandwidth to local memory (16-64kB) • Code with predictable access paerns WHAT’S THE FUTURE OF GPUS? – Small (or no) caches – User-controlled local memories ! big.LITTLE Task Migration Use Model In the big.LITTLE task migration use model the OS and applications only ever execute on Cortex-A15 or Cortex-A7 and never both processors at the same time. This use-model is a natural extension to the Dynamic Voltage and Frequency Scaling (DVFS), operating points provided by current mobile platforms with a single application processor to allow the OS to match the performance30/07/2012 | 27 of the platform to the 30/07/2012 | 28 David Black-Schaffer Uppsala University / Department of Informaon Technology performance required by the application.
Details
-
File Typepdf
-
Upload Time-
-
Content LanguagesEnglish
-
Upload UserAnonymous/Not logged-in
-
File Pages6 Page
-
File Size-