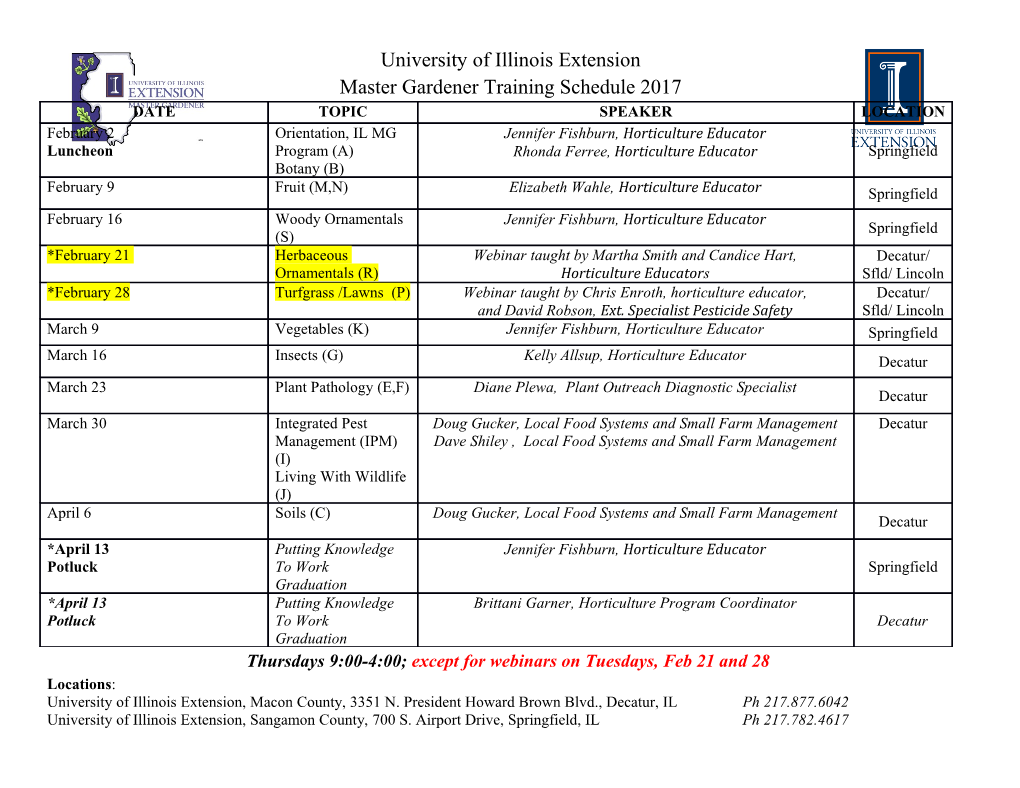
University of Kentucky UKnowledge Theses and Dissertations--Electrical and Computer Engineering Electrical and Computer Engineering 2020 FAULT IDENTIFICATION ON ELECTRICAL TRANSMISSION LINES USING ARTIFICIAL NEURAL NETWORKS Christopher W. Asbery University of Kentucky, [email protected] Author ORCID Identifier: https://orcid.org/0000-0002-1594-7294 Digital Object Identifier: https://doi.org/10.13023/etd.2020.167 Right click to open a feedback form in a new tab to let us know how this document benefits ou.y Recommended Citation Asbery, Christopher W., "FAULT IDENTIFICATION ON ELECTRICAL TRANSMISSION LINES USING ARTIFICIAL NEURAL NETWORKS" (2020). Theses and Dissertations--Electrical and Computer Engineering. 152. https://uknowledge.uky.edu/ece_etds/152 This Doctoral Dissertation is brought to you for free and open access by the Electrical and Computer Engineering at UKnowledge. It has been accepted for inclusion in Theses and Dissertations--Electrical and Computer Engineering by an authorized administrator of UKnowledge. For more information, please contact [email protected]. STUDENT AGREEMENT: I represent that my thesis or dissertation and abstract are my original work. Proper attribution has been given to all outside sources. I understand that I am solely responsible for obtaining any needed copyright permissions. I have obtained needed written permission statement(s) from the owner(s) of each third-party copyrighted matter to be included in my work, allowing electronic distribution (if such use is not permitted by the fair use doctrine) which will be submitted to UKnowledge as Additional File. I hereby grant to The University of Kentucky and its agents the irrevocable, non-exclusive, and royalty-free license to archive and make accessible my work in whole or in part in all forms of media, now or hereafter known. I agree that the document mentioned above may be made available immediately for worldwide access unless an embargo applies. I retain all other ownership rights to the copyright of my work. I also retain the right to use in future works (such as articles or books) all or part of my work. I understand that I am free to register the copyright to my work. REVIEW, APPROVAL AND ACCEPTANCE The document mentioned above has been reviewed and accepted by the student’s advisor, on behalf of the advisory committee, and by the Director of Graduate Studies (DGS), on behalf of the program; we verify that this is the final, approved version of the student’s thesis including all changes required by the advisory committee. The undersigned agree to abide by the statements above. Christopher W. Asbery, Student Dr. Yuan Liao, Major Professor Dr. Aaron Cramer, Director of Graduate Studies FAULT IDENTIFICATION ON ELECTRICAL TRANSMISSION LINES USING ARTIFICIAL NEURAL NETWORKS ________________________________________________________________________ DISSERTATION ________________________________________________________________________ A dissertation submitted in partial fulfillment of the requirements for the degree of Doctor of Philosophy in the College of Engineering at the University of Kentucky By Christopher Wayne Asbery Lexington, Kentucky Director: Dr. Yuan Liao, Professor of Electrical and Computer Engineering Lexington, Kentucky 2020 Copyright © Christopher Wayne Asbery 2020 https://orcid.org/0000-0002-1594-7294 ABSTRACT OF DISSERTATION FAULT IDENTIFICATION ON ELECTRICAL TRANSMISSION LINES USING ARTIFICIAL NEURAL NETWORKS Transmission lines are designed to transport large amounts of electrical power from the point of generation to the point of consumption. Since transmission lines are built to span over long distances, they are frequently exposed to many different situations that can cause abnormal conditions known as electrical faults. Electrical faults, when isolated, can cripple the transmission system as power flows are directed around these faults therefore leading to other numerous potential issues such as thermal and voltage violations, customer interruptions, or cascading events. When faults occur, protection systems installed near the faulted transmission lines will isolate these faults from the transmission system as quickly as possible. Accurate fault location is essential in reducing outage times and enhancing system reliability. Repairing these faulted elements and restoring the transmission lines to service quickly is highly important since outages can create congestion in other parts of the transmission grid, therefore making them more vulnerable to additional outages. Therefore, identifying the classification and location of these faults as quickly and accurately as possible is crucial. Diverse fault location methods exist and have different strengths and weaknesses. This research aims to investigate the use of an intelligent technique based on artificial neural networks. The neural networks will attempt to determine the fault classification and precise fault location. Different fault cases are analyzed on multiple transmission line configurations using various phasor measurement arrangements from the two substations connecting the transmission line. These phasor measurements will be used as inputs into the artificial neural network. The transmission system configurations studied in this research are the two- terminal single and parallel transmission lines. Power flows studied in this work are left static, but multiple sets of fault resistances will be tested at many points along the transmission line. Since any fault that occurs on the transmission system may never experience the same fault resistance or fault location, fault data was collected that relates to different scenarios of fault resistances and fault locations. In order to analyze how many different fault resistance and fault location scenarios need to be collected to allow accurate neural network predictions, multiple sets of fault data were collected. The multiple sets of fault data contain phasor measurements with different sets of fault resistance and fault location combinations. Having the multiple sets of fault data help determine how well the neural networks can predict the fault identification based on more training data. There has been a lack of guidelines on designing the architecture for artificial neural network structures including the number of hidden layers and the number of neurons in each hidden layer. This research will fill this gap by providing insights on choosing effective neural network structures for fault classification and location applications. KEY WORDS: Artificial neural networks, feed forward neural networks, electrical transmission faults, single transmission line, parallel transmission line Christopher Wayne Asbery May 11, 2020 Date FAULT IDENTIFICATION ON ELECTRICAL TRANSMISSION LINES USING ARTIFICIAL NEURAL NETWORKS By Christopher Wayne Asbery Dr. Yuan Liao Director of Dissertation Dr. Aaron Cramer Director of Graduate Studies May 11, 2020 Date DEDICATION First, this dissertation and related PhD research has been dedicated to my beautiful wife and son, Kirsten and Peyton Asbery. Working a full-time job while working towards the completion of my PhD degree has been extremely stressful and rewarding for my entire family and myself. I want to thank them both for their continued support for my career and educational goals that help fulfill my aspirations in life. Having my PhD degree in Electrical and Computer Engineering has been a goal of mine since I began my Electrical and Computer Engineering academic career in May of 2004. Secondly, this dissertation has been dedicated to my loving parents, Jerry and Martha Asbery. My parents have always been extremely supportive of my education and career goals by always encouraging me and providing the support that was needed. Without their continued appreciation of my career development starting at a young age, I’m not sure I would be as successful as I am. Coming from a first-generation college family, my family and I are very proud of my accomplishments and are excited to see how my future unfolds. ACKNOWLEDGEMENTS I would like to express my deepest appreciation and gratitude to the people who helped me complete this PhD dissertation. To begin with, I would like to thank my academic advisor, Dr. Yuan Liao, for his support in taking me under his wing and guiding me through the process of completing this PhD academic research. I want to express my gratitude for my PhD committee members: Dr. Aaron Cramer, Dr. Dan Ionel, and Dr. Jinze Liu. A special thank you to each one of you for taking the time to sit on my committee and helping me complete this academic dissertation. Also, a special thank you goes out to Dr. Nick Jewell for his guidance and time that he has provided throughout this research. Lastly, I would like to thank my employer, Louisville Gas & Electric and Kentucky Utilities (LG&E/KU), and the management staff within the Transmission Strategy and Planning department for encouraging me to come back to school to obtain the PhD degree. Since I have been employed with LG&E/KU, the management team has been extremely supportive for continuing my education ambitions that has helped me meet one of my greatest accomplishments. iii TABLE OF CONTENTS ACKNOWLEDGEMENTS .............................................................................................. III TABLE OF CONTENTS .................................................................................................. IV LIST OF TABLES ........................................................................................................
Details
-
File Typepdf
-
Upload Time-
-
Content LanguagesEnglish
-
Upload UserAnonymous/Not logged-in
-
File Pages218 Page
-
File Size-