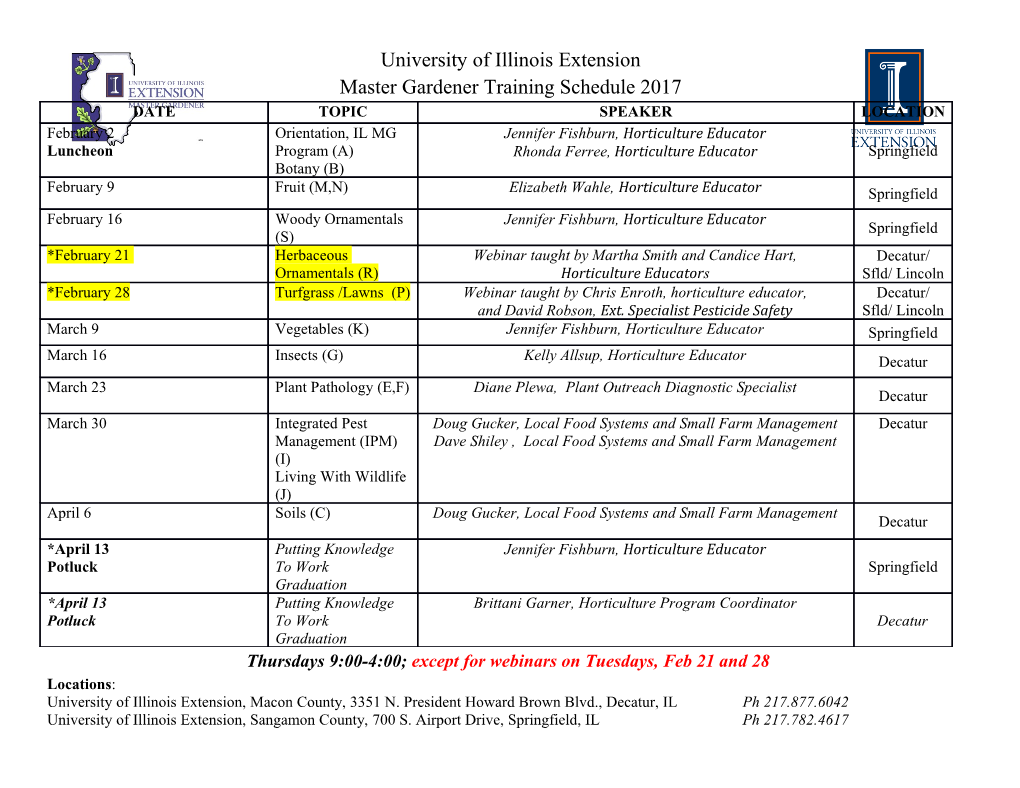
Modern Portfolio Theory Revisited: from Real Traders to New Methods THÈSE NO 5255 (2012) PRÉSENTÉE LE 20 JANVIER 2012 À LA FACULTÉ DES SCIENCES DE BASE LABORATOIRE DE BIOPHYSIQUE STATISTIQUE PROGRAMME DOCTORAL EN PHYSIQUE ÉCOLE POLYTECHNIQUE FÉDÉRALE DE LAUSANNE POUR L'OBTENTION DU GRADE DE DOCTEUR ÈS SCIENCES PAR David Morton DE LACHAPELLE acceptée sur proposition du jury: Prof. N. Grandjean, président du jury Prof. P. De Los Rios, Dr M. Marsili, directeurs de thèse Prof. J.-Ph. Bouchaud, rapporteur Dr F. Hashemi, rapporteur Prof. R. Mantegna, rapporteur Suisse 2012 2Modern Portfolio Theory Revisited Acknowledgement I am very much grateful to Swissquote Bank for the opportunity to carry out my thesis within the Quantitative Asset Management Dept. A warm thank you to the wonderful QAM team members for your constant support and all our fruitful discussions. I am truly indebted to my thesis Director Paolo de Los Rios and co-Director Matteo Marsili for their trust in me and my work, and to the three reviewers of my dissertation: Jean-Philippe Bouchaud, Rosario Mantegna, and Fariba Hashemi, for their involvement in reviewing the manuscript. I dedicate this thesis to my family and friends who all came along for the ride and without whom none of this would have been possible. Special thanks go to my Dad, my Bro, Mum, Isa, SQ Noyau Dur, NT4R, and Aitana & EPFL friends. Last but not least, very special thanks to you my dear and sweet Eva for your unflagging love and support. 3 Résumé Le comportement des traders en-ligne est tout d’abord analysé puis modélisé. On montre que l’investisseur moyen se comporte comme un minimiseur de mean-variance en finance. Sous cette description, on montre également que les frais de transaction des courtiers jouent un rôle pri- mordial dans l’explication des motifs d’investissement révélés par l’analyse, et en particulier dans celle d’une relation semi-empirique reliant l’investissement moyen à la valeur en porte- feuille. Puisque les investisseurs en-ligne tiennent compte des coûts de transaction dans leur stratégie d’investissement, il sont également sensibles aux coût élevés de rotation des positions de leur portefeuille. Des solutions pour éviter de trop importantes fluctuations du portefeuille sont étudiées : tout d’abord dans le cas unidimensionnel, où l’on montre que les estimateurs de dispersion minimale améliorent la précision ainsi que la variance des estimations de volatilité et de valeur-à-risque, puis dans un environnement multi-asset où la matrice de covariance idéale devrait avoir de bonnes propriétés de conditionnement afin de maintenir le turnover du porte- feuille à un niveau raisonnable. Plusieurs résultats théoriques sont obtenus en utilisant la théorie des matrices aléatoires, comme par exemple une équation pour la transformée de Stieltjes de la densité des valeurs propres de la matrices de corrélation de variables i.i.d., construite à l’aide d’un profile de poids décroisant dans le temps. Les résultats trouvés dans le cas unidimen- sionnel sont généralisés, menant à l’introduction des matrices de covariance à longue mémoire. Finalement, un actuel “fléau de la dimension” en finance est abordé par la généralisation de la méthode du Spectral Coarse Graining, une technique de réduction des réseaux complexes qui est étendue ici à la simplification du problème de l’optimisation de la mean-variance. Mots-clés : allocation de portefeuille ; optimisation de la mean-variance ; matrices aléatoires ; valeur-à-risque ; volatilité ; stabilité des estimateurs ; conditionnement des matrices ; spectral coarse- graining 4Modern Portfolio Theory Revisited 5 Summary In the first place the behavior of (online) traders on markets is analyzed and modeled, and it is shown that the average investor behaves as a mean-variance optimizer in finance. Within this description, transaction costs play a key role in explaining observed investment patterns and in particular an important uncovered relation between average investment and portfolio value. As online investors take into account transaction costs in their investment strategy, they are also sensitive to high portfolio rebalancing costs. Solutions to avoid high portfolio turnovers are investigated: first in the one-dimensional case, where it is shown that estimators with minimal- dispersion improve both the accuracy and the variance of volatility and Value-at-Risk forecasts; second, in a multi-asset environment where the ideal covariance matrix must have good con- ditioning properties to maintain reasonable portfolio turnover. Theoretical results are derived using Random Matrix Theory, as for instance an equation for the Stieltjes transform of the eigen- value density of i.i.d. correlation matrices with general time-decreasing weight profiles. Results found in the one-dimensional case are generalized leading to long-memory covariance matri- ces. Finally, a “curse of dimensionality” in portfolio allocation is tackled by generalizing the Spectral Coarse Graining, a method of reduction for complex networks, that is extended to the simplification of the mean-variance optimization problem. Keywords : portfolio allocation ; mean-variance optimization ; random matrices ; value-at-risk ; volatilty ; estimator stability ; matrix conditioning ; spectral coarse-graining 6Modern Portfolio Theory Revisited Contents 1 Motivations 11 1.1Atime-prooftheory.................................... 11 1.2Agentsbuildingtheirportfolios:whencostsandsizematter............. 12 2 Portfolio allocation: the perspective of online investors 17 2.1Motivations......................................... 17 2.2Descriptionofthedata................................... 19 2.3Results............................................ 20 2.3.1 Accountvalues................................... 20 2.3.2 Meanturnover................................... 22 2.3.3 Meanturnovervsaccountvalue......................... 24 2.3.4 Turnoverrescaledbyaccountvalue....................... 26 2.3.4.1 data.................................... 26 2.3.4.2 theory................................... 27 2.4 The influence of transaction costs on trading behavior: optimal mean-variance portfolios........................................... 29 2.4.1 Non-linear relationship between account value and number of assets . 32 2.5Turnover,numberofassetsandaccountvalue..................... 34 2.6Discussionandoutlook.................................. 39 8Modern Portfolio Theory Revisited 3 Volatility long-memory and min-dispersion profiles 41 3.1Weightingthepast:whyandhow............................ 41 3.2Varianceofweightedaveragesandautocorrelation.................. 43 3.2.1 Describing the whole volatility autocorrelation function . ........ 44 3.3Asymptoticbehaviorofthevariance........................... 49 3.4 Weight profilesinducingminimaldispersion...................... 52 3.4.1 Addinguprelevantconstraints.......................... 53 3.4.2 Solvingthemin-dispersionproblem....................... 54 3.5Exponentiallyautocorrelatedprocesses:explicitresults................ 54 3.6 Profiles of volatility with minimum dispersion . ................ 56 3.7 Forecasting Value-at-Risk with min-dispersion profiles................ 59 3.7.1 Historical estimation of VaR with non-uniform time-weighting . .... 60 3.7.2 Backtesting results: comparing the weight profiles.............. 61 3.8Concludingremarks.................................... 65 4 Long-memory covariance matrices 67 4.1Time-weightedcovariancematrices:theissueofconditioning............ 67 4.2Theory............................................ 69 4.2.1 Weightedcovarianceestimator.......................... 69 4.2.2 RandomMatrixTheoryinanutshell...................... 70 4.2.3 Mainresult..................................... 71 4.2.4 Computing the spectral density of i.i.d. weighted correlation matrices . 75 4.3Long-memorycovariancematrices............................ 75 4.3.1 Old and new weight profiles........................... 75 4.3.2 EigenvaluedensityandedgespectrumofPRECestimators......... 78 4.3.3 Simulationresults................................. 82 4.4 Turnover and long-memory profilesinportfolioallocation.............. 82 4.5Conclusion.......................................... 86 CONTENTS 9 5 Simplifying the Mean-Variance Optimization 89 5.1Exploitingstockcorrelationstoreducethecovariancematrixofreturns...... 89 5.2Differenttypesofcovarianceestimators......................... 90 5.3Coarse-grainingtheMean-VarianceOptimizationproblem.............. 91 5.4Buildingthecoarsegrainingoperator.......................... 93 5.5Simulationsandpreliminaryresults........................... 97 5.6Discussionandconclusion................................. 99 A Proofs of Chapter 4 101 A.1ProofofEq.4.9....................................... 101 T = < ∞ A.2 Spectrum of PREC i.i.d. correlation matrices for limN,T N c0 ........ 102 B The Spectral Coarse Graining of matrices: theory and examples from complex network theory 105 B.1Introduction......................................... 105 B.1.1 Overview...................................... 106 B.1.2 NotationsandStyle................................ 106 × B.2 A Note on Projectors in Cn n ............................... 106 B.3ExactCoarseGraining................................... 108 B.4ApproximateCoarseGraining.............................. 110 B.5OptimizingCoarseGrainingunderConstraints.................... 114 B.5.1 Partitioning..................................... 115 B.5.2 HomogeneousMixing............................... 116 ( ) B.5.3 Minimizing eP v :MethodsandAnalysis.................
Details
-
File Typepdf
-
Upload Time-
-
Content LanguagesEnglish
-
Upload UserAnonymous/Not logged-in
-
File Pages137 Page
-
File Size-