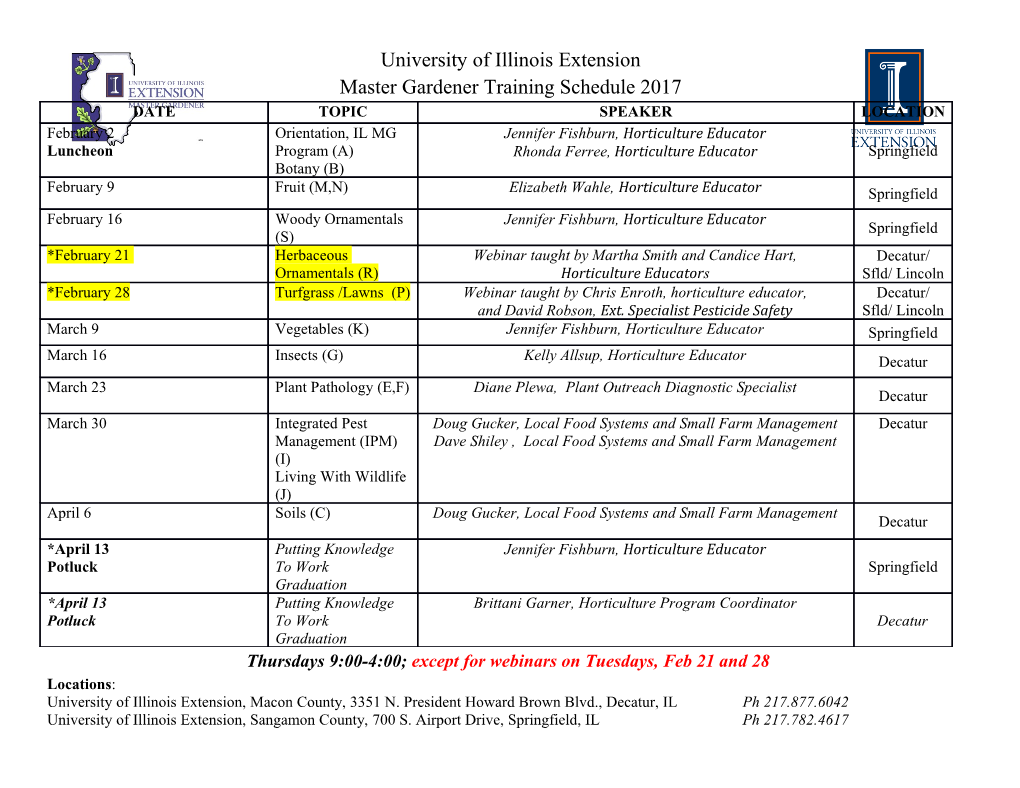
NOISY MARKETS & DEEP LEARNING Written by: Todd Moses imagin.ai Fischer Black, an American Economist, determined that markets move more from noise than by infor- mation. This noise as Black wrote, represents information that had not arrived yet. It reflects uncertainty about future supply and demand. He concluded that those that trade on noise will loose more often than those that trade on information. So what is the difference? Noise is the result of sentiment or opinion while information relates to the fundamental soundness of a security. For example, say a toothpaste company has proven large profits over the past 10 years. The stock price is at $100 per share due to the great performance. However, a television news show just ran a story on how toothpaste is a waste of money. Showing an interview with a dentist who believes everyone is better off brushing their teeth with salt. A few thousand people begin posting pictures of themselves brushing with salt on social media. There are Twitter posts, Facebook discussions, and YouTube videos on how great it is not to use toothpaste. As these events unfold, the stock price is moving downward to $99, $95, $90, and closing at $89 per share. The fundamentals of the company have not changed. They are still selling large amounts of toothpaste and even posted a press release on a new salt version of their toothpaste due out next month. However, the noise of the crowd is causing their stock to drop. Many investors short the stock while those that own it begin to sell. They do this not based on the fact that owning a percent of the company is unwise. Instead, they subscribe to a conclusion derived from the noise. In contrast, the information based investors are trying to buy all they can. 01 It is the noise the creates a liquid market. For a trader to buy a security, one must also be willing to sell. If all involved acted on information over noise, the stock price would remain allot more stable with little noticeable movement. Black’s hypothesis attempts to the answer the question of why people act on noise over information. He concludes that many traders act upon noise simply because they do not realize it as noise. Fundamental analysis takes effort — one has to look up SEC filings for the company in question, analyze financial data, and look for facts. Consider how long it takes Warren Buffett, the poster child of funda- mental investment, to make a buy decision. It is not within minutes or even days. Buffett travels onsite, meets with people, and does the research. Whereas noise is all around. Just read a Facebook feed, open Google News, or watch trading analysis programs on television. Therefore, noise takes far less effort. In 2011, the Journal of Computational Science published an article by Johan Bollen, Huina Moa, and Xiaojun Zeng titled, “Twitter mood predicts the stock market.” According to their findings, one could use sentiment analysis from Twitter feeds to predict the Dow Jones Industrial Average closing within 87.6% accuracy. 02 Perhaps not surprising, they concluded, “Daily variations in public mood states show statistically signifi- cant correlation to daily changes in Dow Jones Industrial Average closing values.” That is clearly the market responding to noise. As a side note, I was able to replicate their findings while working for a hedge fund. However, it may no longer be possible with just one social network. Regardless, it proves that markets move from noise over the short term. In this case, 24 hours. Or does it. Anyone using data has heard that correlation is not causation. Pradeep Mutalik, columnist for Quanta Magazine, concludes, “Complex, interdependent multifactorial causes arise often in nature and therefore in science.” Thus, there may be more to the Twitter experiment than crowd sentiment. Regard- less, this with the research of Fischer Black gives a strong argument that noise is a market driver. The core question now becomes, what drives markets over the long term? Based on the success of fundamental long term traders, this appears to be a combination of both noise and information. A short-term trade is probably better off listening to the noise while a long-term trade (over 1 year) seems to do better when based on information. However, using noise to determine opportunities of discount or inflation is good to have. Now, how can this be used for a Deep Learning trading strategy? Based upon the shocking perfor- mance of AlphaGo, the Go game winning system by Google, perhaps we should let the machine deter- mine it’s own strategy. Allowing it to select the right mix of noise and information for the most profitable course of action. 03 The data source for this Deep Learning system should be a combination of three data-sets: equity pricing data, SEC filings, and Twitter feeds. What we want to accomplish is a system capable of building it’s own successful trading strategy using noise, information, or a combination of both. Like AlphaGo, the machine will determine it’s own best strategy. To begin, we will load the training set arranged by time from 10 to 5 years in the past as the input. Afterwards, the machine will trade over a years worth of time per iteration with the results compared to the actual known results from that year. The known results for that year will be included in the next training set and so on until we reach current day. If successful, the system will trade equities using the API for a demonstration brokerage account. Once satisfied that it has a good strategy — may take several months, we will give it access to a small account using real money. [email protected] (888) 723-8643 www.imaginovation.net 04.
Details
-
File Typepdf
-
Upload Time-
-
Content LanguagesEnglish
-
Upload UserAnonymous/Not logged-in
-
File Pages5 Page
-
File Size-