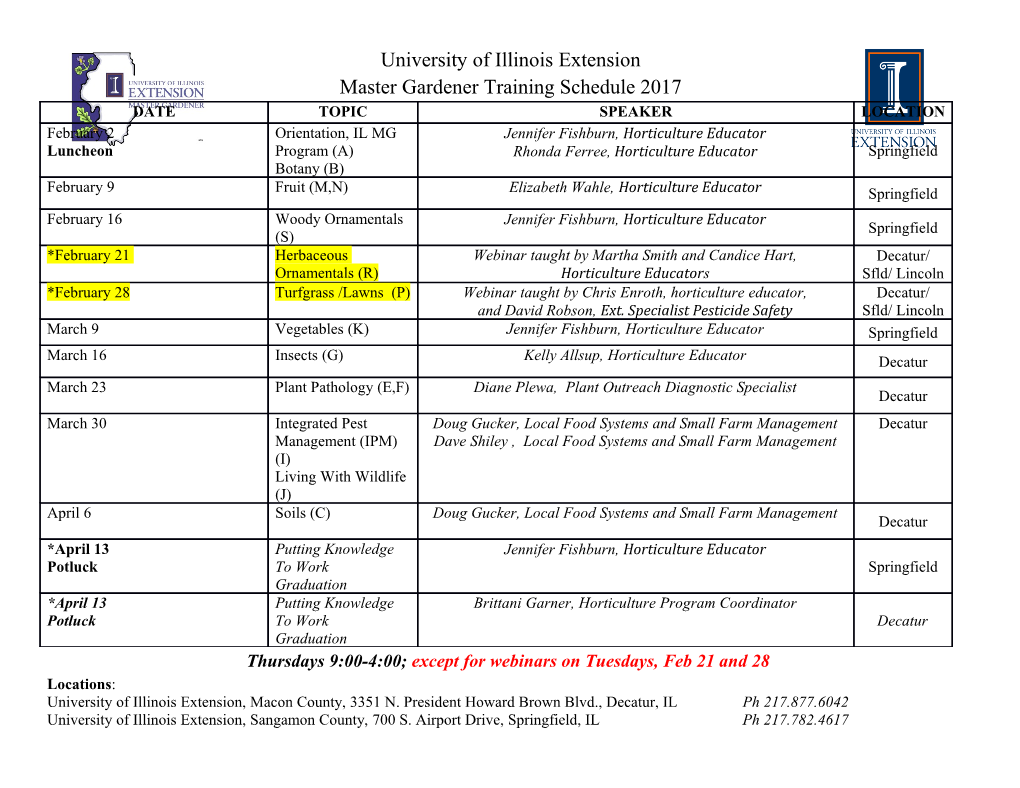
Word Segmentation and Ambiguity in English and Chinese NLP & IR by ± Jin Hu Huang, B.Eng.(Comp.Sc)Grad.Dip.(Soft.Eng.) School of Computer, Engineering and Mathematics, Faculty of Science and Engineering August 10, 2011 A thesis presented to the Flinders University of South Australia in total fulfillment of the requirements for the degree of Doctor of Philosophy in Computer Science Adelaide, South Australia, 2012 c (Jin Hu Huang, 2012) CONTENTS Abstract ::::::::::::::::::::::::::::::::::::: ix Certification ::::::::::::::::::::::::::::::::::: xii Acknowledgements ::::::::::::::::::::::::::::::: xiii Preface ::::::::::::::::::::::::::::::::::::: xv 1. Introduction ::::::::::::::::::::::::::::::::: 1 1.1 Context Sensitive Spelling Correction . 1 1.2 Chinese Pinyin Input . 2 1.3 Chinese Segmentation . 6 1.4 Chinese Information Retrieval (IR) . 9 1.5 Thesis Contribution . 10 1.6 Thesis Organization . 13 Part I Word Disambiguation for English Spelling Checking and Chinese Pinyin Input 15 2. Machine Learning for Context Sensitive Spelling Checking ::::::: 16 2.1 Introduction . 16 2.2 Confused Words . 17 2.3 Context-sensitive Spelling Correction . 18 2.4 Experiment and Result . 21 2.5 Interface . 29 2.6 Conclusion and Future Work . 30 2.7 Reflections . 31 Contents iii 3. Statistical N-gram Language Modeling :::::::::::::::::: 33 3.1 Statistical Language Modeling . 33 3.2 N-Gram Markov Language Models . 36 3.3 Smoothing Methods . 37 3.3.1 Add One Smoothing . 37 3.3.2 Interpolation Smoothing . 38 3.3.3 Absolute Discounting . 38 3.3.4 Good-Turing Discounting . 38 3.3.5 Katz Back-off Smoothing . 39 3.3.6 Witten-Bell Smoothing . 39 3.3.7 Kneser-Ney Smoothing . 40 3.3.8 Modified Kneser-Ney Smoothing . 40 3.4 Discussion . 41 3.5 Conclusion . 41 4. Compression-based Adaptive Approach for Chinese Pinyin Input :::: 42 4.1 Introduction . 42 4.2 Statistical Language Modelling . 44 4.2.1 Pinyin-to-Character Conversion . 45 4.2.2 SLM Evaluation . 45 4.3 Compression Theory . 46 4.4 Adaptive Modelling . 47 4.5 Prediction by Partial Matching . 48 4.6 Experiment and Result . 52 4.7 Conclusion . 54 5. Error-driven Adaptive Language Modeling for Pinyin-to-Character Con- version :::::::::::::::::::::::::::::::::::: 55 5.1 Introduction . 55 5.2 LM Adaption Methods . 56 5.2.1 MAP Methods . 56 5.2.2 Discriminative Training Methods . 57 5.3 Error-driven Adaption . 58 5.4 Experiment and Result . 61 5.5 Conclusion . 65 Contents iv Part II Chinese Word Segmentation and Classification 66 6. Chinese Words and Chinese Word Segmentation ::::::::::::: 67 6.1 Introduction . 67 6.2 The Definition of Chinese Word . 67 6.3 Chinese Word Segmentation . 70 6.3.1 Segmentation Ambiguity . 70 6.3.2 Unknown Words . 71 6.4 Segmentation Standards . 72 6.5 Current Research Work . 73 6.6 Conclusion . 76 7. Chinese Word Segmentation Based on Contextual Entropy ::::::: 78 7.1 Introduction . 78 7.2 Contextual Entropy . 79 7.3 Algorithm . 80 7.3.1 Contextual Entropy . 80 7.3.2 Mutual Information . 82 7.4 Experiment Results . 83 7.5 Conclusion . 90 7.6 Reflections . 90 8. Unsupervised Chinese Word Segmentation and Classification :::::: 92 8.1 Introduction . 92 8.2 Word Classification . 93 8.3 Experiments and Future Work . 96 8.4 Conclusion . 99 8.5 Reflections . 100 Part III Chinese Information Retrieval 101 9. Using Suffix Arrays to Compute Statistical Information ::::::::: 102 9.1 Suffix Trees . 102 9.2 Suffix Arrays . 104 9.3 Computing Term Frequency and Document Frequency . 109 9.4 Conclusion . 112 Contents v 10. N-gram based Approach for Chinese Information Retrieval ::::::: 113 10.1 Introduction . 113 10.2 Chinese Information Retrieval . 114 10.2.1 Single-character-based (Uni-gram) Indexing . 114 10.2.2 Multi-character-based (N-grams) Indexing . 115 10.2.3 Word-based Indexing . 115 10.2.4 Previous Works . 116 10.3 Retrieval Models . 121 10.3.1 Vector Space Model . 121 10.3.2 Term Weighing . 122 10.3.3 Query and Document Similarity . 123 10.3.4 Evaluation . 123 10.4 Experimental Setup . 126 10.4.1 TREC Data . 126 10.4.2 Measuring Retrieval Performance . 127 10.5 Experiments and Discussion . 127 10.5.1 Using Dictionary-based Approach . 127 10.5.2 Statistical Segmentation Approach . 128 10.5.3 Using Different N-grams . 131 10.5.4 Word Extraction . 136 10.5.5 Removing Stop Words . 142 10.6 Discussion . 145 10.7 Conclusion . 147 11. Conclusions ::::::::::::::::::::::::::::::::: 149 11.1 Thesis Review . 149 11.2 Future Work . 151 Appendix 153 A. The Appendix: Tables for TREC 5 & 6 Chinese Information Retrieval Results ::::::::::::::::::::::::::::::::::: 154 B. The Appendix: Examples of TREC 5 & 6 Chinese Queries ::::::: 162 Bibliography :::::::::::::::::::::::::::::::::: 188 LIST OF FIGURES 1.1 Number of Homonyms for Each Pinyin . 4 5.1 Perplexity compare between static and adaptive model on Modern Novel.................................. 62 5.2 Perplexity compare between static and adaptive model on Martial Arts Novel . 63 5.3 Perplexity compare between static and adaptive model on People's Daily 96 . 64 7.1 Contextual Entropy and Mutual Information for \The two world wars happened this century had brought great disasters to human being including China." . 80 10.1 Average Precision At Different Recall For Dictionary-based and Statistical Segmentation Approaches . 129 10.2 Average Precision At X Documents Retrieved For Dictionary-based and Statistical Segmentation Approaches . 130 10.3 Average Precision for 54 Queries Using 1-gram, 2-grams, 3-grams and 4-grams . 134 10.4 The Impact of Extracted Words on 54 Queries . 139 10.5 The Impact of Stop Words on 54 Queries . 143 LIST OF TABLES 2.1 Diameter with occurrences, significance and probability - number of contexts (WSJ 87-89,91-92) . 22 2.2 Relationship between probability and significance - number of con- texts (WSJ 87-89,91-92) . 23 2.3 False errors and coverage testing on test and validation corpora with no errors seeded but two real errors found . 24 2.4 True errors detected (recall) and corrected when errors seeded ran- domly . 25 2.5 Seeded errors of the confusion set of \from" and \form" (S,P≥95%) 25 2.6 False positive rate (FPR), true positive rate (TPR) and informed- ness when errors seeded . 27 2.7 Spelling Errors Found in WSJ0801 and WSJ1231 . 28 4.1 Compression models for the string \dealornodeal" . 47 4.2 PPM model after processing the string dealornodeal . 50 4.3 Compression results for different compression methods . 52 4.4 Character Error Rates for Kneser-Ney, Static and Adaptive PPM 53 5.1 Witten-Bell smoothing model after processing the string dealornodeal 60 5.2 Comparing perplexity and CER using different smoothing methods on testing corpus . 63 5.3 CER and percentage of data used for adaption . 64 5.4 Testing on Xinhua 96 with different mixed models with adaption . 65 6.1 Some differences between the segmentation standards . 73 7.1 Validation results based on Recall, Precision and F-Measure for Eq. 7.1 7.2 7.3 7.4 . 84 7.2 Validation results based on Recall, Precision and F-Measure for Eq. 7.5 7.6 7.7 7.8 . 84 7.3 Validation results on Recall, Precision and F-measure according to Eq. 7.9 7.10 7.11 . 85 List of Tables viii 7.4.
Details
-
File Typepdf
-
Upload Time-
-
Content LanguagesEnglish
-
Upload UserAnonymous/Not logged-in
-
File Pages227 Page
-
File Size-