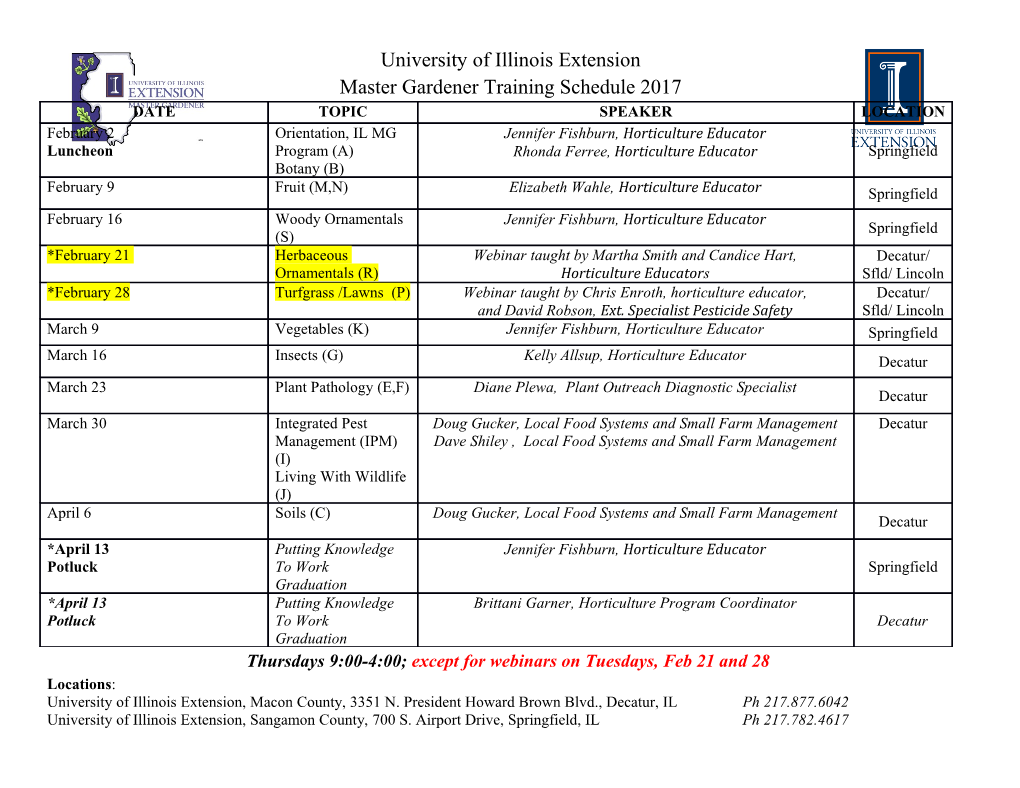
Appendix A Stable Distributions Revisited This appendix is meant to complement the discussions of the central limit theorem (Sect. 2.1) and of stable distributions (Sect. 4.1). Its aim is two-fold. On the one hand, further criteria are presented for testing whether the probability density p() belongs to the domain of attraction of the stable distribution Lα,β (x). On the other hand, useful asymptotic expansions of Lα,β (x) and (most of) the known closed-form expressions for specific α and β are compiled. A.1 Testing for Domains of Attraction Let p() be the probability density of the independent and identically distributed random variables n (n = 1,...,N). Its distribution function F()and characteristic function p(k) are given by +∞ F()= d p ,p(k)= dp() exp(ik). (A.1) −∞ −∞ The density p() is said to belong to the ‘domain of attraction’ of the limiting prob- ability density Lα,β (x) if the distributions of the normalized sums N ˆ 1 SN = n − AN BN n=1 converge to Lα,β (x) in the limit N →∞. In Sect. 4.1, we quoted a theorem that all possible limiting distributions must be stable, i.e., invariant under convolution (see Theorem 4.1). This stability criterion leads to the class of Lévy (stable) distributions Lα,β (x) which are characterized by two parameters, α and β (see Theorem 4.2). The Gaussian is a specific example of Lα,β (x) with α = 2. W. Paul, J. Baschnagel, Stochastic Processes, DOI 10.1007/978-3-319-00327-6, 237 © Springer International Publishing Switzerland 2013 238 A Stable Distributions Revisited Theorem A.1 A probability density p() belongs to the domain of attraction of the Gaussian if and only if 2 y ||>y dp() lim = 0, (A.2) y→∞ 2 ||<y d p() and to the domain of Lα,β (x) with 0 <α<2 if and only if 1. F(−) c− lim = , →∞ 1 − F() c+ 2. 1 − F()+ F(−) lim = rα ∀r>0, →∞ 1 − F(r)+ F(−r) where c+ and c− are proportional to the amplitudes of the asymptotic behavior of p() for large ± (see (4.16)). Alternatively, one can also test for the domains of attraction by means of the characteristic function due to the following theorem: Theorem A.2 The density p() belongs to the domain of attraction of the Gaussian if and only if Re[ln p(rk)] lim = k2 (any k ≥ 0) (A.3) r→0 Re[ln p(r)] converges uniformly with respect to k, and to the domain of Lα,β (x) with 0 <α<2 if and only if the following conditions are satisfied for k ≥ 0 and r → 0: 1. 0 <α<2, α = 1: Re[ln p(rk) ] lim = k (A.4) r→0 Re[ln p(r) ] and Im[ln p(rk) ] π lim = βrα tan α . (A.5) r→0 Re[ln p(r) ] 2 2. α = 1: Re[ln p(rk) ] lim = k (A.6) r→0 Re[ln p(r) ] and Im[ln p(rk) − r ln p(k) ] 2β lim = k ln k. (A.7) r→0 Re[ln p(r) ] π In these equations, pstands for p(k) = p(k) for α ≤ 1, − p(k) = e i x kp(k) for α>1. Both theorems are taken from [62]. A.2 Closed-Form Expressions and Asymptotic Behavior 239 A.2 Closed-Form Expressions and Asymptotic Behavior Although the canonical representation completely defines the expression of all pos- sible stable distributions by (see (4.11)) k ln L (k) = iγk− c|k|α 1 + iβ ω(k,α) , α,β |k| where γ is an arbitrary real constant, c ≥ 0, 0 <α≤ 2, −1 ≤ β ≤ 1, and the function ω(k,α) is given by tan(πα/2) for α = 1, ω(k,α) = (2/π) ln |k| for α = 1, the inverse Fourier transform of Lα,β (k) can only be carried out in a limited number of cases. In the following, we compile (most of) these cases and present several useful asymptotic expansions. Our presentation is based on the excellent discussions in [17, 144, 147], where further details can also be found. The Gaussian: α = 2 If α = 2, ω(k,2) = 0. Then L2,β (x) becomes independent of β and is given by +∞ − 2 1 −ik(x−γ)−ck2 1 (x γ) L2(x) = dke = √ exp − . (A.8) 2π −∞ 4πc 4c This shows that γ merely shifts the origin and c is only a scale factor if the imag- inary term of (4.11) vanishes. Therefore, we set γ = 0 and c = 1 for the following discussion of the symmetric cases, where β = 0. The Symmetric Cases: β = 0 If β = 0, Lα,0(x) is an even function of x. Explicit expressions are known for α = 1/2, 2/3, 1. α = 1/2: +∞ 1 1/2 L1/2,0(x) = dk exp −ikx −|k| 2π −∞ 1 ∞ = dk cos(kx) exp −k1/2 π 0 ∞ 1 − = dkk 1/2 sin(kx) exp −k1/2 2πx 0 √ ∞ y= kx 1 y = y y2 − , 3/2 d sin exp 1/2 (A.9) πx 0 x 240 A Stable Distributions Revisited where we used an integration by parts to obtain the penultimate expression. The integral in (A.9) is given by (see [1], p. 303, Eq. (7.4.23)) 2 1/2 ∞ y y y2 − d sin exp 1/2 π 0 x 1 1 1 1 1 1 = − C cos + − S sin , 2 2πx 4x 2 2πx 4x (A.10) where x>0 and C(x) and S(x) are Fresnel integrals x 1 x 1 C(x) = dy cos πy2 ,S(x)= dy sin πy2 . (A.11) 0 2 0 2 The solution of the integral in (A.10) is tabulated in [1], where it is abbreviated by g(x) (see Table 7.8, p. 323 and Eq. (7.3.6), p. 300). The function g(x) starts at 1/2forx = 0 and decreases monotonously towards zero if x tends to infinity. Therefore, the full solution for the stable distribution reads 1 1/2 L / , (x) = g [1/2πx] . (A.12) 1 2 0 (2π)1/2x3/2 α = 2/3: The stable law was found by Zolotarev and can be related to the Whittaker function Wu,v(x) ([1], p. 505) by [147] ∞ 1 2/3 L2/3,0(x) = dk cos(kx) exp −k π 0 3 1/2 1 2 4 = exp − W / , / . (A.13) π x 27x2 1 2 1 6 27x2 α = 1: This yields the Cauchy distribution +∞ 1 −ikx−|k| 1 1 L1,0(x) = dke = . (A.14) 2π −∞ π 1 + x2 In addition to these explicit formulas, the asymptotic expansions for the general case of α are known if x → 0 and x →∞. A series expansion for small x can be obtained from +∞ ∞ 1 α 1 α Lα,0(x) = dk exp −ikx −|k| = dk cos(kx) exp −k 2π −∞ π 0 ∞ 1 (−1)n ∞ = x2n dkk2n exp −kα π (2n)! n=0 0 ∞ n y=kα 1 (−1) 1 + 2n = Γ x2n. (A.15) πα (2n)! α n=0 A.2 Closed-Form Expressions and Asymptotic Behavior 241 This series diverges for all x if 0 <α<1 and converges for all x if 1 <α<2. In the divergent range, it may further be considered as an asymptotic expansion around x = 0. If x = 0, only the n = 0termof(A.15) survives and one obtains 1 1 L (0) = Γ . (A.16) α,0 πα α On the other hand, Wintner found the following large-x expansion [144, 147]: ∞ α (−1)n Γ([1 + n]α) 1 Lα, (x) = sin π(n+ 1)α (A.17) 0 π n! xα(n+1)+1 2 n=0 ∞ 1 (−1)n+1 Γ(1 + nα) 1 = sin πnα , (A.18) π n! xαn+1 2 n=1 in which we used Γ 1 + (n + 1)α = (n + 1)αΓ (n + 1)α in the last step. The series (A.17) and (A.18) converge for x>0 when 0 <α<1, but diverge for all x if 1 <α<2. Even in the first case, the convergence is very slow if x is small. The leading term of this expansion is Γ(1 + α)sin(πα/2) 1 L (x) ∼ (x →∞). (A.19) α,0 π xα+1 The Asymmetric Cases: β =±1 If 0 <α<1, the stable distributions are con- centrated in the intervals (−∞, 0] and [0, ∞) (choosing γ = 0) for β = 1 and β =−1, respectively. We want to illustrate this general property by two examples where closed-form expressions can be obtained, α = 1/3 and α = 1/2: α = 1/3: Let K1/3(x) be the modified Bessel function of order 1/3. This function is real and positive for x>0 and tends to zero for x →∞[1]. If 0 <x<∞, Zolotarev found that Lα,β (x) with α = 1/3, β =−1 and c = 1 can be related to K1/3(x) by the following expression [17, 147]: 1 2 3/2 31/44 2 1/2 L / ,− (x) = K / . (A.20) 1 3 1 31/4π 3x 1 3 9 3x α = 1/2: For this choice, tan(πα/2) = 1. Choose, again, c = 1. Then the stable distribution reads +∞ 1 1/2 k L1/2,−1(x) = dk exp −ikx −|k| 1 − i π −∞ |k| 2 1 ∞ = dk cos kx − k1/2 exp −k1/2 π 0 ∞ y=k1/2 2 = dyy cos y2x − y exp(−y).
Details
-
File Typepdf
-
Upload Time-
-
Content LanguagesEnglish
-
Upload UserAnonymous/Not logged-in
-
File Pages41 Page
-
File Size-