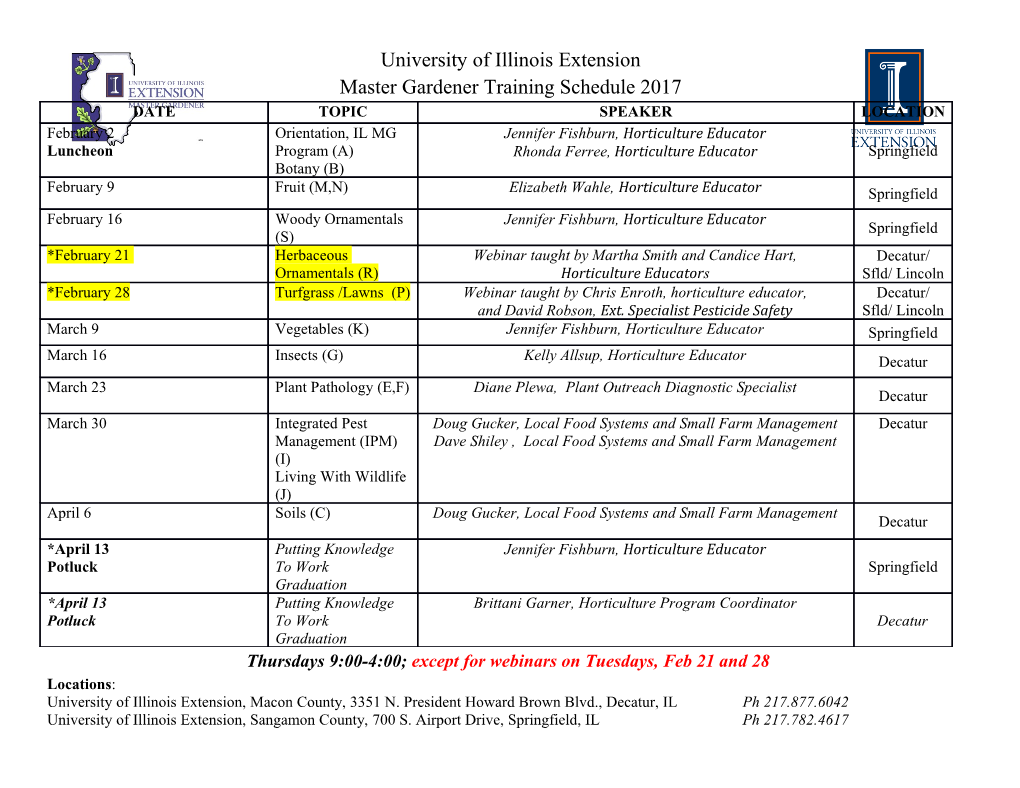
Lecture 12: Generative Models Fei-Fei Li & Justin Johnson & Serena Yeung Lecture 12 - 1 May 15, 2018 Administrative Project Milestone due tomorrow (Wed 5/16) A3 due next Wed, 5/23 Fei-Fei Li & Justin Johnson & Serena Yeung Lecture 12 - 2 May 15, 2018 Overview ● Unsupervised Learning ● Generative Models ○ PixelRNN and PixelCNN ○ Variational Autoencoders (VAE) ○ Generative Adversarial Networks (GAN) Fei-Fei Li & Justin Johnson & Serena Yeung Lecture 12 - 3 May 15, 2018 Supervised vs Unsupervised Learning Supervised Learning Data: (x, y) x is data, y is label Goal: Learn a function to map x -> y Examples: Classification, regression, object detection, semantic segmentation, image captioning, etc. Fei-Fei Li & Justin Johnson & Serena Yeung Lecture 12 - 4 May 15, 2018 Supervised vs Unsupervised Learning Supervised Learning Data: (x, y) x is data, y is label Cat Goal: Learn a function to map x -> y Examples: Classification, regression, object detection, Classification semantic segmentation, image captioning, etc. This image is CC0 public domain Fei-Fei Li & Justin Johnson & Serena Yeung Lecture 12 - 5 May 15, 2018 Supervised vs Unsupervised Learning Supervised Learning Data: (x, y) x is data, y is label Goal: Learn a function to map x -> y Examples: Classification, DOG, DOG, CAT regression, object detection, semantic segmentation, image Object Detection captioning, etc. This image is CC0 public domain Fei-Fei Li & Justin Johnson & Serena Yeung Lecture 12 - 6 May 15, 2018 Supervised vs Unsupervised Learning Supervised Learning Data: (x, y) x is data, y is label Goal: Learn a function to map x -> y Examples: Classification, GRASS, CAT, TREE, SKY regression, object detection, semantic segmentation, image Semantic Segmentation captioning, etc. Fei-Fei Li & Justin Johnson & Serena Yeung Lecture 12 - 7 May 15, 2018 Supervised vs Unsupervised Learning Supervised Learning Data: (x, y) x is data, y is label Goal: Learn a function to map x -> y Examples: Classification, A cat sitting on a suitcase on the floor regression, object detection, semantic segmentation, image Image captioning captioning, etc. Caption generated using neuraltalk2 Image is CC0 Public domain. Fei-Fei Li & Justin Johnson & Serena Yeung Lecture 12 - 8 May 15, 2018 Supervised vs Unsupervised Learning Unsupervised Learning Data: x Just data, no labels! Goal: Learn some underlying hidden structure of the data Examples: Clustering, dimensionality reduction, feature learning, density estimation, etc. Fei-Fei Li & Justin Johnson & Serena Yeung Lecture 12 - 9 May 15, 2018 Supervised vs Unsupervised Learning Unsupervised Learning Data: x Just data, no labels! Goal: Learn some underlying hidden structure of the data Examples: Clustering, K-means clustering dimensionality reduction, feature learning, density estimation, etc. This image is CC0 public domain Fei-Fei Li & Justin Johnson & Serena Yeung Lecture 12 - 10 May 15, 2018 Supervised vs Unsupervised Learning Unsupervised Learning Data: x Just data, no labels! Goal: Learn some underlying hidden structure of the data 3-d 2-d Examples: Clustering, Principal Component Analysis dimensionality reduction, feature (Dimensionality reduction) learning, density estimation, etc. This image from Matthias Scholz is CC0 public domain Fei-Fei Li & Justin Johnson & Serena Yeung Lecture 12 - 11 May 15, 2018 Supervised vs Unsupervised Learning Unsupervised Learning Data: x Just data, no labels! Goal: Learn some underlying hidden structure of the data Examples: Clustering, dimensionality reduction, feature Autoencoders learning, density estimation, etc. (Feature learning) Fei-Fei Li & Justin Johnson & Serena Yeung Lecture 12 - 12 May 15, 2018 Supervised vs Unsupervised Learning Unsupervised Learning Data: x Figure copyright Ian Goodfellow, 2016. Reproduced with permission. Just data, no labels! 1-d density estimation Goal: Learn some underlying hidden structure of the data Examples: Clustering, dimensionality reduction, feature 2-d density estimation learning, density estimation, etc. 2-d density images left and right are CC0 public domain Fei-Fei Li & Justin Johnson & Serena Yeung Lecture 12 - 13 May 15, 2018 Supervised vs Unsupervised Learning Supervised Learning Unsupervised Learning Data: (x, y) Data: x x is data, y is label Just data, no labels! Goal: Learn a function to map x -> y Goal: Learn some underlying hidden structure of the data Examples: Classification, regression, object detection, Examples: Clustering, semantic segmentation, image dimensionality reduction, feature captioning, etc. learning, density estimation, etc. Fei-Fei Li & Justin Johnson & Serena Yeung Lecture 12 - 14 May 15, 2018 Supervised vs Unsupervised Learning Supervised Learning Unsupervised Learning Training data is cheap Data: (x, y) Data: x Holy grail: Solve x is data, y is label Just data, no labels! unsupervised learning => understand structure of visual world Goal: Learn a function to map x -> y Goal: Learn some underlying hidden structure of the data Examples: Classification, regression, object detection, Examples: Clustering, semantic segmentation, image dimensionality reduction, feature captioning, etc. learning, density estimation, etc. Fei-Fei Li & Justin Johnson & Serena Yeung Lecture 12 - 15 May 15, 2018 Generative Models Given training data, generate new samples from same distribution Training data ~ pdata(x) Generated samples ~ pmodel(x) Want to learn pmodel(x) similar to pdata(x) Fei-Fei Li & Justin Johnson & Serena Yeung Lecture 12 - 16 May 15, 2018 Generative Models Given training data, generate new samples from same distribution Training data ~ pdata(x) Generated samples ~ pmodel(x) Want to learn pmodel(x) similar to pdata(x) Addresses density estimation, a core problem in unsupervised learning Several flavors: - Explicit density estimation: explicitly define and solve for pmodel(x) - Implicit density estimation: learn model that can sample from pmodel(x) w/o explicitly defining it Fei-Fei Li & Justin Johnson & Serena Yeung Lecture 12 - 17 May 15, 2018 Why Generative Models? - Realistic samples for artwork, super-resolution, colorization, etc. - Generative models of time-series data can be used for simulation and planning (reinforcement learning applications!) - Training generative models can also enable inference of latent representations that can be useful as general features FIgures from L-R are copyright: (1) Alec Radford et al. 2016; (2) David Berthelot et al. 2017; Phillip Isola et al. 2017. Reproduced with authors permission. Fei-Fei Li & Justin Johnson & Serena Yeung Lecture 12 - 18 May 15, 2018 Taxonomy of Generative Models Direct GAN Generative models Explicit density Implicit density Markov Chain Tractable density Approximate density GSN Fully Visible Belief Nets - NADE - MADE Variational Markov Chain - PixelRNN/CNN Variational Autoencoder Boltzmann Machine Change of variables models (nonlinear ICA) Figure copyright and adapted from Ian Goodfellow, Tutorial on Generative Adversarial Networks, 2017. Fei-Fei Li & Justin Johnson & Serena Yeung Lecture 12 - 19 May 15, 2018 Taxonomy of Generative Models Direct Today: discuss 3 most GAN popular types of generative Generative models models today Explicit density Implicit density Markov Chain Tractable density Approximate density GSN Fully Visible Belief Nets - NADE - MADE Variational Markov Chain - PixelRNN/CNN Variational Autoencoder Boltzmann Machine Change of variables models (nonlinear ICA) Figure copyright and adapted from Ian Goodfellow, Tutorial on Generative Adversarial Networks, 2017. Fei-Fei Li & Justin Johnson & Serena Yeung Lecture 12 - 20 May 15, 2018 PixelRNN and PixelCNN Fei-Fei Li & Justin Johnson & Serena Yeung Lecture 12 - 21 May 15, 2018 Fully visible belief network Explicit density model Use chain rule to decompose likelihood of an image x into product of 1-d distributions: Likelihood of Probability of i’th pixel value image x given all previous pixels Then maximize likelihood of training data Fei-Fei Li & Justin Johnson & Serena Yeung Lecture 12 - 22 May 15, 2018 Fully visible belief network Explicit density model Use chain rule to decompose likelihood of an image x into product of 1-d distributions: Likelihood of Probability of i’th pixel value image x given all previous pixels Complex distribution over pixel values => Express using a neural Then maximize likelihood of training data network! Fei-Fei Li & Justin Johnson & Serena Yeung Lecture 12 - 23 May 15, 2018 Fully visible belief network Explicit density model Use chain rule to decompose likelihood of an image x into product of 1-d distributions: Will need to define ordering of “previous Likelihood of Probability of i’th pixel value pixels” image x given all previous pixels Complex distribution over pixel values => Express using a neural Then maximize likelihood of training data network! Fei-Fei Li & Justin Johnson & Serena Yeung Lecture 12 - 24 May 15, 2018 PixelRNN [van der Oord et al. 2016] Generate image pixels starting from corner Dependency on previous pixels modeled using an RNN (LSTM) Fei-Fei Li & Justin Johnson & Serena Yeung Lecture 12 - 25 May 15, 2018 PixelRNN [van der Oord et al. 2016] Generate image pixels starting from corner Dependency on previous pixels modeled using an RNN (LSTM) Fei-Fei Li & Justin Johnson & Serena Yeung Lecture 12 - 26 May 15, 2018 PixelRNN [van der Oord et al. 2016] Generate image pixels starting from corner Dependency on previous pixels modeled using an RNN (LSTM) Fei-Fei Li & Justin Johnson & Serena Yeung Lecture 12 - 27 May 15, 2018 PixelRNN [van der Oord et al. 2016] Generate
Details
-
File Typepdf
-
Upload Time-
-
Content LanguagesEnglish
-
Upload UserAnonymous/Not logged-in
-
File Pages134 Page
-
File Size-