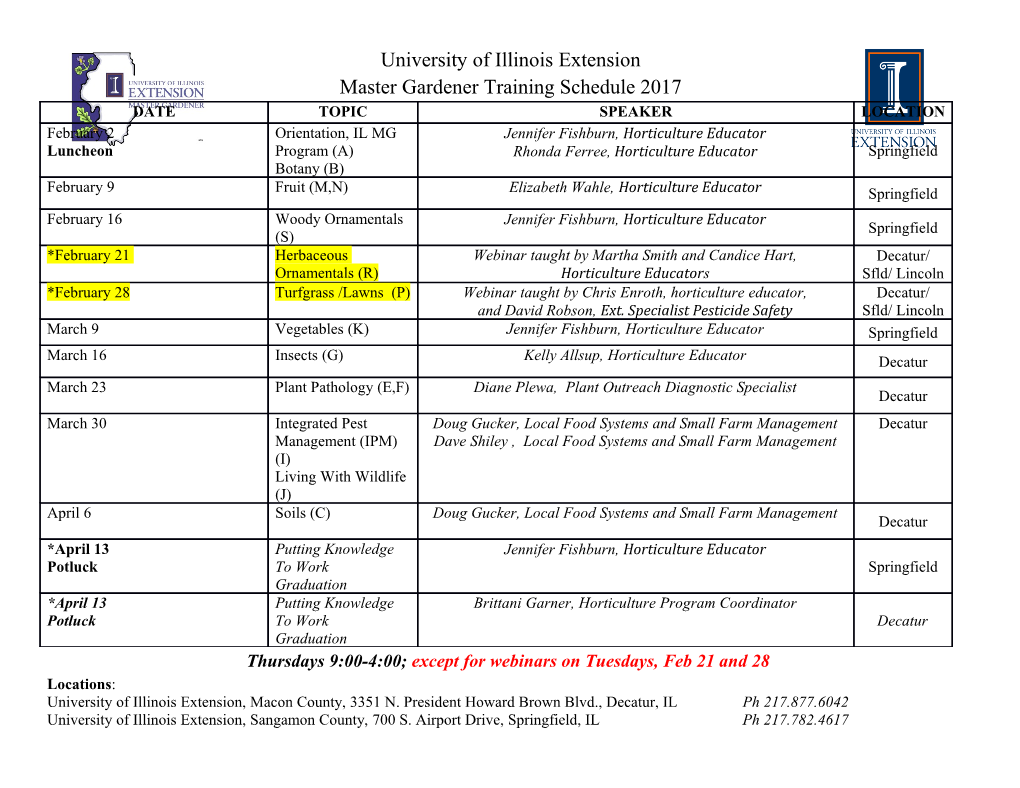
Citation for published version Escalera Guerrero, S., Fabian, J., Pardo, P., Baró Solé, X., Gonzàlez Sabaté, J., Escalante, H.J., Misevic, D., Steiner, U. & Guyon, I. (2016). ChaLearn Looking at People 2015: Apparent Age and Cultural Event Recognition Datasets and Results. IEEE International Conference on Computer Vision. Proceedings, 2016(), 243-251. doi: 10.1109/ICCVW.2015.40 DOI https://doi.org/10.1109/ICCVW.2015.40 Document Version This is the Accepted Manuscript version. The version in the Universitat Oberta de Catalunya institutional repository, O2 may differ from the final published version. Copyright and Reuse This manuscript version is made available under the terms of the Creative Commons Attribution Non Commercial No Derivatives licence (CC-BY-NC-ND) http://creativecommons.org/licenses/by-nc-nd/3.0/es, which permits others to download it and share it with others as long as they credit you, but they can’t change it in any way or use them commercially. Enquiries If you believe this document infringes copyright, please contact the Research Team at: [email protected] Universitat Oberta de Catalunya Research archive ChaLearn Looking at People 2015: Apparent Age and Cultural Event Recognition datasets and results Sergio Escalera Junior Fabian Pablo Pardo Universitat de Barcelona and CVC Computer Vision Center Universitat de Barcelona Xavier Baro´ Jordi Gonzalez` Hugo J. Escalante Universitat Oberta de Catalunya Universitat Autonoma` de Barcelona INAOE Mexico Computer Vision Center Computer Vision Center Dusan Misevic Ulrich Steiner Isabelle Guyon Center for Research and Interdisciplinarity Max-Planck Odense Center Clopinet, ChaLearn Abstract as well as technical limitations such as spatial and temporal resolution. Facial expressions analysis and age estimation Following previous series on Looking at People (LAP) are hot topics in Looking at People that serve as additional competitions [14, 13, 11, 12, 2], in 2015 ChaLearn ran two cues to determine human behavior and mood indicators. Fi- new competitions within the field of Looking at People: (1) nally, images of cultural events constitute a very challeng- age estimation, and (2) cultural event recognition, both in ing recognition problem due to a high variability of gar- still images. We developed a crowd-sourcing application to ments, objects, human poses and scene context. Therefore, collect and label data about the apparent age of people (as how to combine and exploit all this knowledge from pixels opposed to the real age). In terms of cultural event recog- constitutes an interesting problem. nition, one hundred categories had to be recognized. These These challenges motivated our choice to organize a new tasks involved scene understanding and human body anal- ICCV workshop and a competition on these topics to sus- ysis. This paper summarizes both challenges and data, as tain the effort of the computer vision community. These well as the results achieved by the participants of the com- new competitions come as a natural evolution from our pre- petition. Details of the ChaLearn LAP competitions can be vious workshops at CVPR2011, CVPR2012, ICPR2012, found at http://gesture.chalearn.org/. ICMI2013, ECCV2014 and CVPR2015. We continued us- ing our website http://gesture.chalearn.org for promotion and challenge entries in the quantitative competition were 1. Introduction scored on-line using the Codalab Microsoft-Stanford Uni- versity platforms (http://codalab.org/). The automatic analysis of the human body, also named In the rest of this paper, we describe in more detail both Looking at People, in still images and image sequences age estimation and cultural event recognition challenges, keeps making rapid progress with and the constant improve- their relevance in the context of the state of the art, and de- ment of new published methods that rapidly advance the scribe the results achieved by the participants in both chal- state-of-the-art. lenges. In 2015, ChaLearn organized new competitions and workshops on age estimation and cultural event recogni- 2. Age estimation challenge tion from still images. The recognition of continuous, nat- ural human signals and activities is very challenging due Age estimation is a difficult task which requires the au- to the multimodal nature of the visual cues (e.g., move- tomatic detection and interpretation of facial features. We ments of fingers and lips, facial expressions, body pose), have designed an application using the Facebook API for 17141 the collaborative harversting and labeling by the commu- nity in a gamified fashion (http://sunai.uoc.edu:8005/). Age estimation has historically been one of the most challenging problems within the field of facial analysis [32, 17, 21]. It can be very useful for several applications, such as advanced video surveillance, demographic statis- tics collection, business intelligence and customer profiling, and search optimization in large databases. Different ap- plication scenarios can benefit from learning systems that (a) Game Panel predict the apparent age, such as medical diagnosis (prema- ture aging due to environment, sickness, depression, stress, fatigue, etc.), effect of anti-aging treatment (hormone re- placement therapy, topical treatments), or effect of cosmet- ics, haircuts, accessories and plastic surgery, just to mention a few. Some of the reasons age estimation is still a chal- lenging problem are the uncontrollable nature of the aging process, the strong specificity to the personal traits of each individual, high variance of observations within the same (b) Gallery Panel age range, and the fact that it is very hard to gather com- plete and sufficient data to train accurate models. 2.1. Dataset Due to the nature of the age estimation problem, there is a restricted number of publicly available databases pro- viding a substantial number of face images labeled with ac- curate age information. Table 1 shows the summary of the existing databases with main reference, number of samples, (c) Ranking Panel number of subjects, age range, type of age and additional information. This field has experienced a renewed inter- Figure 1. Age Recognition Application. (a) User can see the im- est from 2006 on, since the availability of large databases ages of the rest of participants and vote for the apparent age. (b) User can upload images and see their uploads and the opinion of like MORPH-Album 2 [34], which increased by 55× the the users regarding the apparent age of people in their photos. (c) amount of real age-annotated data with respect to traditional User can see the points he/she achieves by uploading and voting age databases. Therefore, this database has extensively been photos and the ranking among his/her friends and all the partici- used in recent works by applying to it different descriptors pants of the application. and classification schemes. However, all existing datasets are based on real age estimation. In the present challenge, we propose the first dataset to recognize the apparent age of a global and friends leaderboard where the users could see people based on the opinion of many subjects using a new their position in the ranking. We asked the users to upload crowd-sourcing data collection and labeling application and images of a single person and we gave them tools to crop the 1 the data from AgeGuess platform . image if necessary, we also asked them to provide the real We developed a web application in order to collect and age for images they uploaded themselves (or a good approx- label an age estimation dataset online by the community. imation), allowing more analysis and comparisons between The application uses the Facebook API to facilitate the ac- real age and apparent age. cess hence reach more people with a broader background. In order to increase the number of images in the It also allows us to easily collect data from the participants, database, an exhaustive research was done to find similar such as gender, nationality and age. We show some panels applications which were collecting the same, or very close, of the application in the Figure 1(a), 1(b) and 1(c). type of data. The result of this search was AgeGuess, which The web application was developed in a gamified way, collects nearly the same information as we do. The line of i.e. the users or players get points for uploading and label- research of the AgeGuess team is focused on aging and age ing images, the closer the age guess was to the apparent age perception from a biologically demographical point of view. (average labeled age) the more points the player obtained. The AgeGuess team agreed to partner with the HuPBA re- In order to increase the engagement of the players, we added search group and the ChaLeran platform to joint effort in the 1AgeGuess: http://www.ageguess.org/ data collection. The Table 2 shows the main characteristics 17152 Table 1. Age-based Databases and its characteristics. Database #Faces #Subj. Range Type of age Controlled Balanced age Other annotation Enviroment Distrivution FG-NET [25, 24] 1,002 82 0 - 69 Real Age No No 68 Facial Landmarks GROUPS [19] 28,231 28,231 0 - 66+ Age group No No - PAL [27] 580 580 19 - 93 Age group No No - FRGC [31] 44,278 568 18 - 70 Real Age Partially No - MORPH2 [35] 55,134 13,618 16 - 77 Real Age Yes No - YGA [18] 8,000 1,600 0 - 93 Real Age No No - FERET[30] 14,126 1,199 - Real Age Partially No - Iranian face [3] 3,600 616 2 - 85 Real Age No No Kind of skin and cosmetic points PIE [37] 41,638 68 - Real Age Yes No - WIT-BD [42] 26,222 5,500 3 - 85 Age group No No - Caucasian Face 147 - 20 - 62 Real Age Yes No 208 Shape Landmarks Database [5] LHI [1] 8,000 8,000 9 - 89 Real Age Yes Yes - HOIP [16] 306,600 300 15 - 64 Age Group Yes No - Ni’s Web- 219,892 - 1 - 80 Real Age No No - Collected Database [28] OUI-Adience [9] 26.580 2.284 0 - 60+ Age Group No No Gender of the final database.
Details
-
File Typepdf
-
Upload Time-
-
Content LanguagesEnglish
-
Upload UserAnonymous/Not logged-in
-
File Pages10 Page
-
File Size-