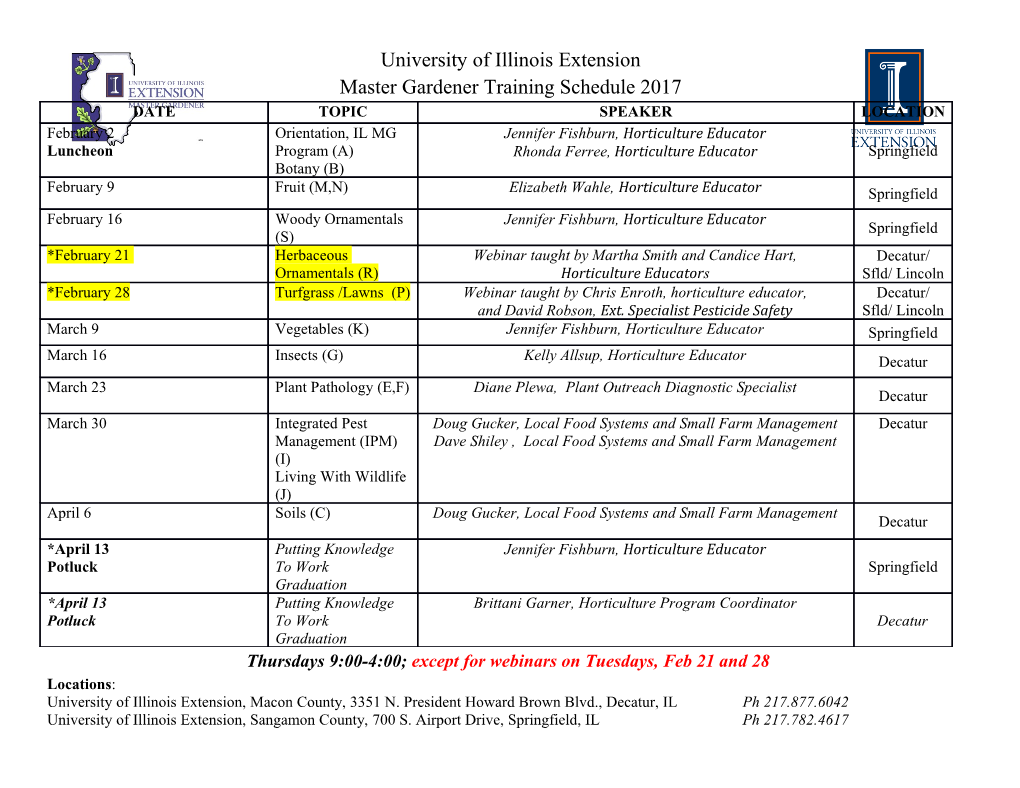
716 JOURNAL OF SOFTWARE, VOL. 6, NO. 4, APRIL 2011 Integration of Grey with Neural Network Model and Its Application in Data Mining Changjun Zhu College of Urban Construction, Hebei University of Engineering, Handan, 056038, China [email protected] Qinghua Luan College of Water Conservancy and Hydropower, Hebei University of Engineering, Handan, 056038, China Zhenchun Hao, Qin Ju State Key Laboratory of Hydrology-Water Resources and Hydraulic Engineering, Hohai University, Nanjing, China Abstract—Because of Boundary types and geologic which include advection, diffusion, dispersion, sorption, conditions, which possess random and obscure decay and biodegradation. In the courses,there is not characteristics, groundwater heads vary with the conditions. only the known information but also uncertain The prediction of groundwater level is one of the main work of hydraulic government, which is predicted based on the information, therefore, it can be seen as one gray system. history data and the relative influence factors. Therefore, Groundwater trends are related to atmosphere prediction precision depends on the accuracy of history precipitation. There is obvious seasonality variation of data. Data mining has provided a new method for analyzing atmosphere precipitation. Therefore, the groundwater massive, complex and noisy data. According to the level is changed by the seasons and periods. In this case, complexity and ambiguity of groundwater system, a new we needed to use the original data of the groundwater in integration of grey with neural network model is built to order to predict the groundwater level. In this paper, we forecast groundwater heads, which were used to judge calculated the cycle groundwater, trend groundwater, and whether future groundwater heads were extraordinarily random groundwater separately after isolating the three over the history range or not. This method overcomes the disadvantages which the grey method only predict the linear items. Traditionally, we analyzed and predicted trend. The methods were used to analyze the random groundwater using the determinacy and random statistical characteristics of groundwater heads in anyang city. The mathematics model (such as, finite element, finite results indicate that the method is reliable, and reasonable. difference, analysis, harmony wave analysis, time series analysis, probability statistic, etc) . These methods are Index Terms—grey degree, groundwater level, neural mainly based on the linear theory. Since the models are network, Anyang city simple, the precision is not high (Zhang et al., 2002; Luo et al., 2003) . I. I. INTRODUCTION The development and management of groundwater resources should include trends in groundwater head The Gray systematic theory is proposed by Chinese variation. In this study, the statistics estimation method professor Deng Jurong (1985) in 1982. In the theory, was used to build a wrapping band for groundwater level there is not only a large amount of known information variation based on groundwater observation data. called white system, but also much unknown and uncertain information called black system. The system II. PREDICTION METHOD including white system and black system is called gray system. Contaminant transport in an underground system A. Principle of GM (1,1) usually occurs in varied flow fields and in anisotropic and The essence of GM (1,1) [5] is to accumulate the original data in heterogenous media. Because the applicability of order to obtain regular data. By setting up the differential analytical solutions is extremely limited for such equation model, we obtain the fitted curve in order to predict the conditions, numerical techniques are essential for system. Assuming the observed original water quality is as underground pollution modeling. Mecarthy(1989) , Basha follows: (0) (0) (0) (0) & El-Habel(1993) , Li et al.(2004) ,Li & Wang(2004) {x ()tx= ( (1), x (2)," , xn ( )} made much work about the uncertain issues. Among the The data were treated with an accumulated generating numerical techniques, the gray numerical method has operation(AGO) : become very popular and is recognized as a powerful (1) (1) (1) (1) numerical tool. The distribution and transport of x ()tx= ( (1), x (2)," , xn ( ) (1) pollutants mentioned by Liu et al.(2003) ,Chen & { } Wagenet(1995) , Xu et al.(2002) in groundwater are controlled by physical chemistry and biology functions, © 2011 ACADEMY PUBLISHER doi:10.4304/jsw.6.4.716-723 JOURNAL OF SOFTWARE, VOL. 6, NO. 4, APRIL 2011 717 k σ σ (1) (0) interval of µ is , where Where: x ()kxi= ∑ (), kn=1, 2," ; [x − Z α , x + Z α ] t=1 2 n 2 n (1) Mean value of x x = ((2),(3),xx" xn ()) Z α is the accumulated probability corresponding to Where: 2 1 (1) (1) Data Process xt()=+− ( x () t x ( t 1))tn= 2,3," , 2 Corresponding differential equation: (1) dx (1) +=ax u (2) To build up differential equation with dt exponential form as Utilizing least square method to solve parameters au, . (1) dx (1) Where t is the time, a and u are the parameters to be determined. + ax = b ∧ dt ⎡⎤a TT−1 aBBBY==() (3) ⎢⎥u N ⎣⎦ To solve the differential equation −x(2) 1 ⎡⎤(0) ⎡⎤ x (2) (1) −a(t−1) (0) b b ⎢⎥⎢⎥(0) x (t) = e [x (1) − ] + −x(3) 1 x (3) a a where B = ⎢⎥ Y = ⎢⎥ ⎢⎥## N ⎢⎥# ⎢⎥⎢⎥ −xn() 1 ⎢⎥x(0) ()n ⎣⎦⎣⎦ To build up grey differential equation as (1) Grey predicting model of x : x (0) (t) + az (1) (t) = b,t = 2,3,4,"n ∧ (1) (0) uu−at xt(1)(+= x (1)) − e + (4) aa (0) Grey predicting model of x To find the a and b values by least square ∧ (0) method and build the grey prediction model aat(0) u − x (1)(1)((1))texe+=− − GM(1,1) a (5) Figure 1. Flow chart of grey method of groundwater level In order to differentiate models good or bad, the methods are adopted as follows: α ∧ (0) standard normal value at ( Y.-L.Yeh, T.-C.Chen,2004, (0) ek = x (k) − x (k) k = 1,2,", n) 2 (0) Hogg RV, Tanis EA 1988 ) − n − n 1 1 (0) e = e x = xt() In this study, the confidence interval of groundwater ∑ k ∑ level can be as the grey interval.. the grey degree can be n k =1 n t=1 defined as Eq. (6 ) n − n − 2 1 (0) 2 2 1 2 S = [x (k) − x] S = [e(k) − e] nout 1 n ∑ 2 n ∑ G = (6) k =1 k =1 d nall − S 2 While c = < 0.35 P = {}| ek − e |< 06745S1 > 0.95 , C. RBF Neural network S1 the precision of the models is very accurate indeed. A radial basis function (RBF) is a real-valued function whose value depends only on the distance from the B. Prediction of grey interval origin, so that φ(x) = φ(|| x ||) ; or alternatively on the 2 Assuming the mean value , x ,and variance, σ of distance from some other point c, called a center, so that the groundwater level in every month can be found. φ(x,c) = φ(|| x − c ||) . Any function ϕ that satisfies Accorinf to the large numbers principles, when the number of the data is enough , the mean of samples the property φ(x) = φ(|| x ||) is a radial function. The x can be expressed as a normal distribution, norm is usually Euclidean distance. σ (x − µ) Radial basis functions are typically used to build up N(µ, ) ,and satisfies normal distribution, function approximations of the form n σ N ( ) y(x) = w φ(|| x − c ||) (7) n ∑ i i i=1 where µ is the mean of population. If the confidence where the approximating function y(x) is represented level, (1−α) , of µ is given, then the confidence as a sum of N radial basis functions, each associated with © 2011 ACADEMY PUBLISHER 718 JOURNAL OF SOFTWARE, VOL. 6, NO. 4, APRIL 2011 a different center ci, and weighted by an appropriate establishment of the model. RBF neural network is one of coefficient wi. Approximation schemes of this kind have the most important network in ANN at present, and so been particularly used in time series prediction and far, it is widely used in hydrological science. The GM- control of nonlinear systems exhibiting sufficiently ANN coupling model is based on the GM model of simple chaotic behaviour, 3D reconstruction in computer groundwater, using the GM model of groundwater to graphics (for ex. hierarchical RBF) . simulate the groundwater level, then the groundwater level of different observation wells is as the input of the neural network to predict the groundwater level. III. APPLICATION A. Study area Spring area of small south China sea is located in linzhou city and anyang county in henan province. River water system belongs to Wei river water system in haihe basin, the main river is huan river, and there are a Xiaonanhai revervior and “artificial Tianhe” –red flag canal. The vents is in Huan river valley, the exposed elevation is between 131-135m. Overall look in spring, Figure 2. RBF neural network Structure western mountains and Lin basin aer recharge are, and the central low mountains is runoff area, and eastern There are three learning parameters:: center of RBF Xinan sea is discharge area, whose total area is 2 CK 、σ K WK . The process of algorithm as followings: 934.6Km . karst groundwater is buried deeply, the (1) choosing one series C from input matrix; minimum depth is more than 10m, the influence of K evaporation of groundwater on level is very small, and (2) Calculating variance the type of karst water discharge is mainly water d σ = max (8) drainage, mine drainage and artificial mining. The K precipitation and evaporation measured in 2003, Where d max is the maximum distance, K is the 2004 ,2005 at 6# station is shown in figure.5.
Details
-
File Typepdf
-
Upload Time-
-
Content LanguagesEnglish
-
Upload UserAnonymous/Not logged-in
-
File Pages8 Page
-
File Size-