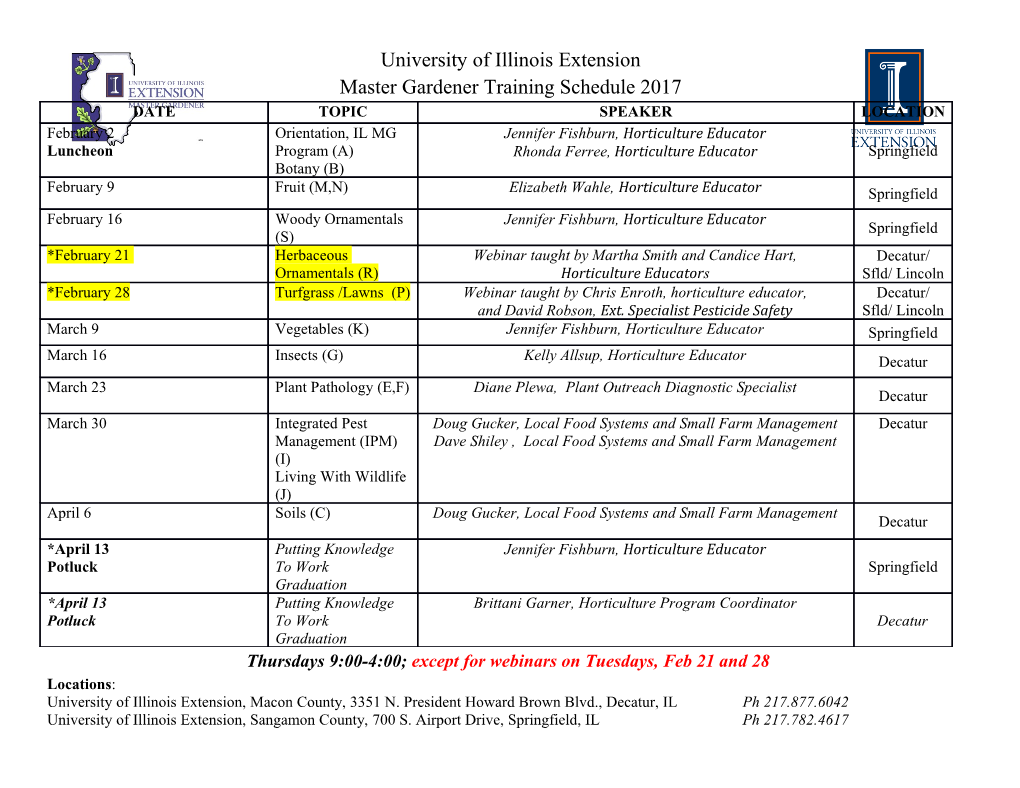
In-Datacenter Performance Analysis of a Tensor Processing Unit By NP Jouppi et al. Presented by Alex Appel Note: Some slides adapted from Dave Patterson’s talk at EECS Colloqium with the same title Agenda - Introduction/Motivation - Architecture - Performance Comparisons - Main highlights/Summary - Questions Origin of Tensor Processing Unit - Projection: if people searched by voice for 3 minutes a day it would double Google’s computation demands - Domain-specific architecture is the solution - Goal: Make the inference phase 10X of GPUs - Very short development cycle: ~15 months Key Neural Net Concepts - Training (learning) in development vs Inference (prediction) in production - Batch size - Amortize weight-fetch time by inferring (or training) many input examples at a time - Quantization - Floating point is useful, but uses a lot more energy and takes more time - Do the training in floating point on GPUs, inference in integers 3 Types of NNs Represent 95% of Google Inference Workload - Multi-Layer Perceptrons (MLP) - Each new layer is a set of nonlinear functions of a weighted sum of all outputs from prior layer ("fully connected") - Convolutional Neural Network (CNN) - Popular for vision, each layer is a set of nonlinear functions of weighted sums at different coordinates of spatially nearby subsets of outputs from the prior layer, which allows the weights to be reused - Recurrent Neural Networks (RNN) / “Long Short-Term Memory” - Each subsequent layer is a collection of nonlinear functions of weighted sums of outputs and the previous state. Inference Datacenter Workload (95%) TPU Architecture - Matrix Unit has 65,536 (256x256) 8-bit multiply-accumulate units - 700 MHz clock rate - Peak: 92 trillion operations/second - >25X multiply-accumulate units vs GPU - >100X multiply-accumulate units vs CPU - 4 MiB of on-chip Accumulator memory - 24 MiB of on-chip Unified Buffer (activation memory) TPU Chip - Unified Buffer: 29% - Matrix Multiply Unit: 24% - Control: 2% Main CISC Instructions - Read_Host_Memory - Reads data from the CPU host memory into the Unified Buffer (UB) - Write_Host_Memory - Writes data from the Unified Buffer into the CPU host memory - Read_Weights - Reads weights from Weight Memory into the Weight FIFO as input to the Matrix Unit - MatrixMultiply/Convolve - Causes the Matrix Unit to perform a matrix multiply or a convolution from the Unified Buffer into the Accumulators - Activate - Performs the nonlinear function of the artificial neuron, with options for ReLU, Sigmoid, and so on Circuit Board Performance Comparisons Roofline Model - Y-axis: - FLOPs - X-axis: - Arithmetic Intensity - How many operations per byte fetched? TPU Roofline - Very high peak performance - Bottlenecked by memory bandwidth Haswell (CPU) Die Roofline - Lower peak performance - More memory bandwidth - The neural nets are not as close to the top as with the TPU K80 (GPU) Die Roofline - Higher memory bandwidth than CPU - The neural nets are far from their Roofline Relative Performance Table Performance/Watt Comparisons - GPU vs CPU: 1.2X-2.1X total performance/Watt - GPU vs CPU: 1.7X-2.9X incremental performance/Watt - TPU vs CPU: 17X-34X total performance/Watt - TPU vs GPU: 14X-16X total performance/Watt - TPU vs CPU: 41X-83X incremental performance/Watt - TPU vs GPU: 25X-29X incremental performance/Watt Energy Proportionality - TPU has lowest power - 40W per die - Poor energy proportionality - At 10% load, the TPU uses 88% of the power it uses at 100% Summary - Inference apps usually emphasize response-time over throughput since they are often user facing. - As a result of latency limits, the K80 GPU is just a little faster for inference than the Haswell CPU, despite it having much higher peak performance and memory bandwidth. - While most architects are accelerating CNNs, they are just 5% of Google's datacenter workload. - The TPU is about 15X – 30X faster at inference than the K80 GPU and the Haswell CPU. Summary (contd.) - Four of the six NN apps that were tested are memory bound; if the TPU were revised to have the same memory as the K80 GPU, it would be about 30 – 50X faster than the GPU and CPU. - Despite having a much smaller and lower power chip, the TPU has 25 times as many multiply accumulators and 3.5 times as much on-chip memory as the K80 GPU. - The performance per Watt of the TPU is 30X – 80X that of its contemporary CPUs and GPUs; a revised TPU with K80 memory would be 70X – 200X better. Resources Link to paper: https://www.cse.wustl.edu/~roger/566S.s21/P1-Norman-1.pdf Link to Dave Patterson talk: Dave Patterson Evaluation of the Tensor Processing Unit Thank you for listening! Questions?.
Details
-
File Typepdf
-
Upload Time-
-
Content LanguagesEnglish
-
Upload UserAnonymous/Not logged-in
-
File Pages23 Page
-
File Size-