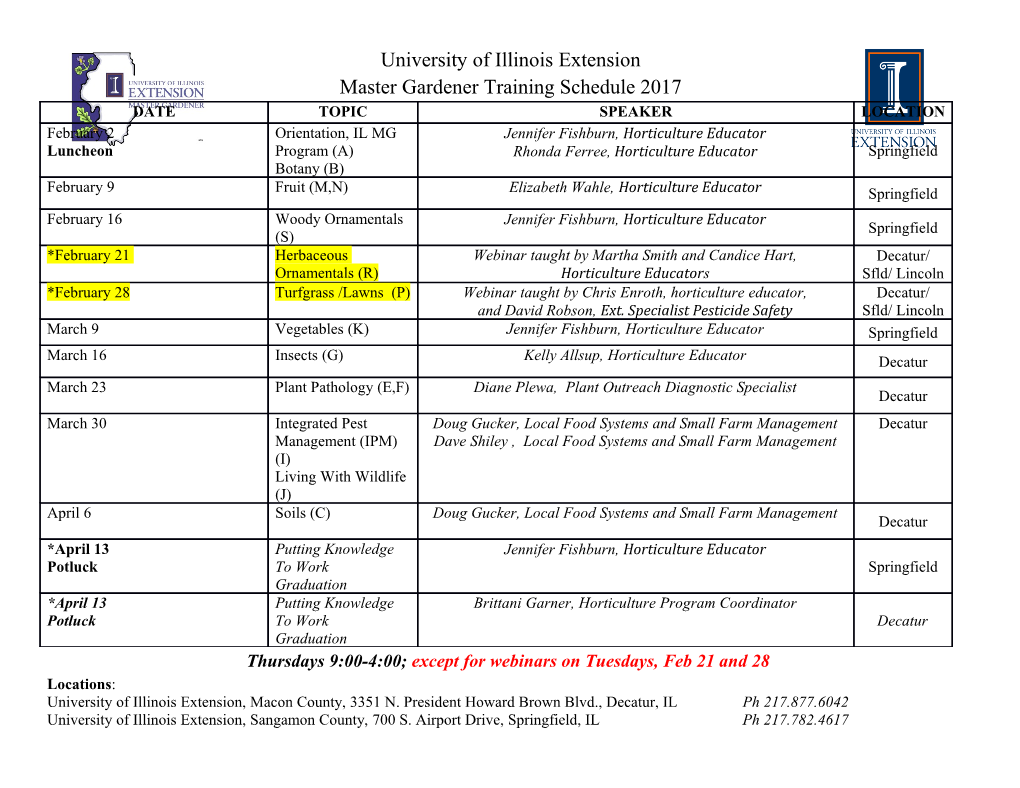
Binders Full Of Women: Convergence of Independently-Generated Hashtags on Twitter CS 224W Diego Pontoriero Evelyn Gillie Group 48 [email protected] [email protected] Abstract world events actually transform into specific Twitter-wide memes in the first place remains an open question. In In social networks, topic propagation is an area of this paper we investigate this mechanism by aligning large obvious interest and frequent study. However, the samples of Twitter data with instances of significant ex- methods by which topics arise in the first place is ternal events and searching for criteria that cause certain less understood. Though some topics may arise memes to arise and, in some cases, subsequently flourish. from purely internal memes, many are derived in real-time from events affecting users in the real Analyzing and modeling convergence of world. independently-generated hashtags on Twitter. We analyze a large sample of Twitter data with Our analysis is concerned with Twitter topics from the a focus on topic (hashtag) creation as a response time of their inception to the time that they become to external stimuli. We present several examples trending, i.e. are being utilized by a relatively large in which disparate topic instances converge into a number of Twitter users. Intuitively, in order for a small prevailing set. We also propose a simple sim- new topic to flourish it must be relatable by a large ulation model that reasonably matches observed number of people. Accordingly, we have selected several behavior. examples of widely-watched events (Olympic events, Presidential Debates) where notable external stimula, 1 Introduction such as memorable phrases or occurrences, were directly responsible for topics that became trending on Twitter. Twitter and other social media sites provide a unique view Using a large sample of Twitter data we are then able into the real-time zeitgeist: by being constantly connected to identify topics that were created as a response to this to social networks via laptops and smartphones, users have stimulus and track them to see which flourish and which effectively transformed news consumption into news par- do not. ticipation. Accordingly, social media sources are now a fixture in media reporting, since they provide an instanta- A drawback to our approach is that for financial and lo- neous and interactive perspective of what millions of inter- gistical reasons we do not have access to the entire Twitter net users are interested in and passionate about. The com- social graph or full stream of Tweets. Therefore, in addi- bined effect of the 2012 United States Presidential election, tion to a qualitative and quantitative analysis of Twitter 2012 Summer Olympics, and other significant, globally- data, we also present a simulation model that provides ad- visible news events has resulted in a landmark year for ditional insight into the observed results. This model uti- Twitter activity. Twitter records were broken [1] and re- lizes a simple scale-free network [8] to simulate the prop- broken [2], [3] with each successive event, and some topics agation competing meme instances. even took on internet lives of their own. For example, the The remainder of this paper is organized as follows. phrase \binders full of women," fatefully uttered by Gov- First, we discuss relevant prior work in the area of so- ernor Mitt Romney during the second Presidential Debate cial network analysis and meme propagation. Second, we resulted in a flurry of responses on Twitter, Facebook, and outline the method by which we assembled our data set Tumblr [4]. and provide some high-level descriptive statistics. Third, In order to detect trends from thousands of Twit- we describe our analytical approach of the data and our ter posts, Twitter algorithmically extracts trending top- initial findings. Fourth, we introduce a simulation model ics based on the content of the tweets. Additionally, an for meme creation and propagation. Finally, we conclude organically-developed but de facto-standardized mecha- and discuss areas for further work. nism called a hashtag|a word or phrase preceded by a # symbol, such as #bieberfever or #yolo|is employed 2 Related Work by Twitter users to explicitly mark a tweet as belonging Due to its size, data availability, and certain interesting to a certain topic. properties, Twitter is a prevalent subject of study in the Several previous works [5], [6], [7] address the question literature. Kwak et al [10] present an empirical overview of how topics propagate throughout Twitter, i.e. using of the Twitter social graph structure and user behavior. contagion models. However, the mechanism by which real- The authors observe that the graph generally follows a 1 power law degree distribution with a few small deviations 3 Real-World Meme Creation due to initially suggested people to follow, a restriction on the maximum number of people to follow, and an un- 3.1 Data Collection explained long tail which the authors believe is due to a In August, we started collected Twitter data using Twit- preponderance of celebrities. ter's streaming API on an EC2 instance. This collected Several prior works directly address the topic of Twit- around 55-60 tweets per second during peak hours, and ter hashtag propagation. Romero et al [6] present a broad 25-30 during non-peak hours. For the third Presidential empirical study of hashtag propagation on Twitter, pa- debate and election day, we filtered the stream on the key- rameterize a model based on their observations, and then words 'obama' and 'romney' in order to get a more pre- run a simulation based on these parameters. By hand- cise stream on memes related to the debate and election, classifying hashtags into a number of qualitative categories and after the election, we returns to collecting the random the authors uncover several differences in the propagation stream. of hashtags as a function of their categories. However, the 3.2 Data Processing authors are primarily concerned with measures of sticki- ness and persistence of already-established hashtags and Since we were only interested in hashtags, we parsed the do not address their formation. tweets and indexed the hashtags by 10-minute intervals. Myers and Leskovec [7] propose a model that extends We did not think it necessary to perform any stemming, the Independent Cascade Model to account for the effect but did lower-case all tags. of prior exposures to infections on a the probability of ac- 3.3 Analysis quiring new infection. The motivation for the model is based on the insight that information does not spread in We focus on notable events with significant twitter activ- isolation, but rather many contagions propagate through a ity, and measure the popularity of unique hashtags rep- network simultaneously and either cooperating or compet- resenting a common idea over time. Three events that ing with one other for survival. They formulate a statisti- we inspect are 1) the anniversary of the 9/11 attack, 2) cal model that uses three factors|inherent content viral- the third presidential debate, and 3) the November 6 gen- ity, inherent user bias to share, and a content interaction eral election. Graphs of hash tag frequencies by hour term that captures the cross-content effect of exposure to are shown in Figure 3 and Figure 2. These graphs il- one piece of information has on the adoption of others| lustrate two examples of hashtag convergence over time; to compute the probability that a user will adopt a new observe the set of 9/11 hashtags: at 9am PST there piece of information. However, whereas the authors are are several competing hashtags describing 9/11, of which only concerned with users analyzing their Twitter feeds none has more than sixty occurrences, and only five have and deciding which items to re-share, we are interested more than twenty occurrences: #neverforget, #9/11, in the origins of these memes as well as their subsequent #11september, #september11th, and #911. By noon adoption. PST, #neverforget is by far the most popular tag with Leskovec et al [5] examine the effect of external sources 468 uses per hour in our stream. The next most frequent on information propagation through Twitter by measuring hashtag is #remember911 with 188 occurrences; note that the lag in peaks of attention from news outlets to blogs and #remember911 was not in our top five at 9am. By 4pm online media. Their work is primarily concerned with de- #remember911 is outperforming #neverforget, and ends veloping an algorithm for generating variants of phrases so the day at the top. that they might increase recall of discussion about a given Similarly, election day starts with many varia- phrase. Compared to our work, their approach only han- tions of 'vote' hashtags: #votedem, #voteobama2012, dles information flowing from a single authoritative source, #vote4obama, #voteobama12p, etc., but users e.g. the person quoted. Our work, on the other hand, con- flock to #voteobama. As an interesting side note: siders the interpretation of real-time primary sources, e.g. #voteforromney was the most popular romney-related live television, by many internet users who then go on to hashtag until #voteobama became popular, at which share and consume these interpretations online via social point #voteromney became more popular, perhaps as a media. response. Ratkiewicz et al [11] present a framework for detecting We look at a few measures to detect predictors of hash- and tracking memes in relatively real-time as they arise tag success: the influence (in terms of total number of in Twitter. This system uses a hand-curated list of 2500 followers) of users using a particular hashtag (we call keywords to filter and extract politically-oriented tweets. this "impressions", and what proportion of hashtag us- The purpose of this system is to perform diffusion and ages were in retweets.
Details
-
File Typepdf
-
Upload Time-
-
Content LanguagesEnglish
-
Upload UserAnonymous/Not logged-in
-
File Pages8 Page
-
File Size-