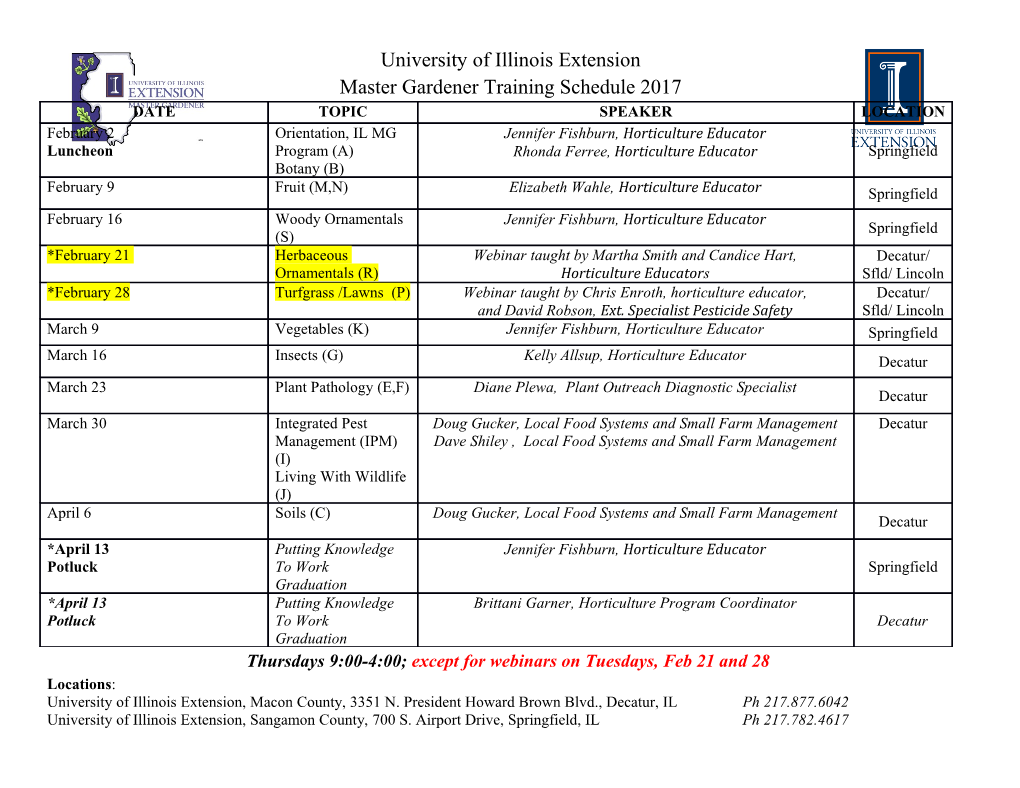
DISS. ETH NO. 25527 Development and Application of Bespoke Machine Learning Lipophilicity Models for Peptides A thesis submitted to attain the degree of DOCTOR OF SCIENCES of ETH ZURICH (Dr. sc. ETH Zürich) presented by JENS-ALEXANDER FUCHS Pharmacist (State Examination) University of Bonn born on September 12, 1988 Citizen of Germany accepted on the recommendation of Prof. Dr. Gisbert Schneider - examiner Prof. Dr. Stefanie Krämer - co-examiner 2018 ii c 2018 Jens A. Fuchs: Development and Application of Bespoke Machine Learning Lipophilicity Models for Peptides iii This work is dedicated to my wife Lisa, my parents Gabi and Wolfgang, and sister Jasmin. Your sympathy fosters my personality, well-being, and my thoughts about life and science. “Our species needs, and deserves, a citizenry with minds wide awake and a basic understanding of how the world works.” Carl Sagan iv Publications Parts of this thesis were published in: • J. A. Fuchs, F. Grisoni, M. Kossenjans, J. A. Hiss, G. Schneider, "Lipophilicity prediction of peptides and peptide derivatives by consensus machine learning", Medicinal Chemistry Communications 2018, 9, 1538-1546. The discussed concepts of peptide quantification and generative modelling by artificial neural networks are published in: • M. D. Allenspach*, J. A. Fuchs*, N. Doriot, J. A. Hiss, G. Schneider, C. Steuer, "Quantification of hydrolyzed peptides and proteins by amino acid fluorescence" Journal of Peptide Science 2018, e3113. • A. Gupta, A. T. Müller, B. J. H. Huisman, J. A. Fuchs, P. Schneider, G. Schneider, "Generative recurrent networks for de novo drug design", Molecular Informatics 2018, 37, 1-2. v Contents PUBLICATIONS iv LIST OF FIGURES AND TABLES viii LIST OF ABBREVIATIONS xi SUMMARY xiii ZUSAMMENFASSUNG xv 1 INTRODUCTION 1 1.1 Lipophilicity: A Fundamental Concept for Pharmacokinetic and Phar- macodynamic Assessment in Drug Discovery ................ 1 Partition and Distribution Coefficients . 4 Experimental Approaches to Determine Partition and Distribution Coef- ficients . 6 In Silico Calculation of Partition- and Distribution-Coefficients . 9 1.2 Peptides in Drug Discovery ........................... 13 Advantages and Drawbacks of Peptides . 14 Overcoming the Drawbacks of Peptides by Combining Biotechnology and Medicinal Chemistry . 15 Lipophilicity of Peptides and Peptide-Mimetics . 18 1.3 Machine Learning for the Prediction of Pharmaceutically Relevant Prop- erties ......................................... 20 Molecular Representation . 21 Unsupervised Algorithms . 26 Supervised Algorithms . 29 Model Evaluation . 35 Applicability Domain . 37 1.4 Evolutionary Algorithms ............................. 39 1.5 Protein-Protein Interactions in Drug Discovery . 41 The Chemokine System . 42 vi CCR7 and CCL19/CCL21 . 45 2 AIMS OF THIS THESIS 47 3 MATERIALS AND METHODS 49 3.1 Laboratory Methods ............................... 49 Peptide Synthesis . 49 Peptide Analytics and Purification . 51 Shake-Flask Method . 51 Microscale Thermophoresis . 53 3.2 Computational Methods ............................. 54 Software . 54 Molecular Representation and Descriptor Calculation . 55 Machine Learning . 55 Datasets . 56 De Novo Peptide Design . 57 4 RESULTS AND DISCUSSION 59 4.1 Baseline Models .................................. 59 Introduction . 59 Feature Selection and Dimensionality Reduction . 60 Results for Modelling with Lasso Features vs. PCA Scores . 63 Predictions from Baseline Models for Peptides up to a Length of Six AA . 64 Discussion . 66 4.2 Expanded Models ................................. 68 Introduction and Hypothesis . 68 Results for Modelling LIPOPEP vs. AZ . 68 Final Consensus Model based on the Pooled Data . 71 Domain of Applicability . 73 Discussion . 76 4.3 Benchmarking the Final Consensus Model . 80 Introduction and Hypothesis . 80 Methods . 80 Results . 81 Discussion . 85 4.4 Focussed De Novo Generated Peptide Libraries for Studying Chemokine- Receptor / Ligand Interactions .......................... 87 Introduction . 87 Fragmentation of CCR7_C24A . 89 vii De Novo Peptide Generation by Simulated Molecular Evolution and Ranking 90 Binding Affinities of Selected Offsprings . 92 Discussion . 93 5 CONCLUSIONS AND OUTLOOK 97 6 ACKNOWLEDGEMENTS 101 BIBLIOGRAPHY 103 ASUPPLEMENTARY INFORMATION 125 A.1 Supplementary Information to Chapter 1 . 125 A.2 Supplementary Information to Chapter 4.1 . 126 A.3 Supplementary Information to Chapter 4.2 . 149 A.4 Supplementary Information to Chapter 4.3 . 151 A.5 Supplementary Information to Chapter 4.4 . 152 viii List of Figures 1.1 The Drug Discovery Pathway . 3 1.2 LogD vs. pH Profile of Buprenorphine . 5 1.3 Molecular Structures of Cyclosporin A, Desmopressin, Daptomycin and Carbetocin . 17 1.4 Dataset Preparation and Machine Learning Workflow . 21 1.5 1D - 3D Molecular Representations . 23 1.6 Principal Component Analysis . 27 1.7 k-mean Clustering . 29 1.8 Support Vector Machines . 33 1.9 Ensemble Prediction by Cascaded Jury Networks . 35 1.10 Cross-Validation and y-Randomisation . 36 1.11 Covered Chemical Space and Applicability Domain . 38 1.12 NMR-Structures of CCL19 and CCL21 . 42 1.13 Sequence Alignment N-Termini of Homeostatic CC- Chemokine Receptors 43 1.14 Schematic Depiction of the CCR7/CCL19 Site 1 Interaction . 46 4.1 Feature Selection by Lasso. 60 4.2 Loadingplot of the Lasso-selected Features . 61 4.3 PCA Scree Plot. 62 4.4 Heatmaps of SVR hyper-parametrisation . 63 4.5 Baseline Model Predictions for the In-House Peptides . 65 4.6 Y-Randomisation . 67 4.7 Performances LIPOPEP vs. AZ . 69 4.8 Differences between LIPOPEP and AZ. 70 4.9 Consensus Results . 72 4.10 Williams Plots . 75 4.11 Retraining ACDlabs and Chemaxon. Flagging Molecules by ADMET- Predictor. 82 4.12 Benchmarking: Model Performances in Relation to Ionisability, Molecu- lar Weight and Liophilicity. 84 4.13 Overview of the CCR7 Project . 88 4.14 MST-Curves of CCR7_C24A, CCR7_10.1 and CCR7_6.4 . 89 4.15 Fragmentation of CCR7_C24A . 90 ix 4.16 Properties of Virtual Peptide Libraries. 92 List of Tables 1.1 Selected Experimental Methods for Direct and Indirect Lipophilicity De- termination. 8 1.2 Selected "Classic" Methods for logP Prediction. 10 1.3 Selected QSPR Methods for Lipophilicity Prediction. 12 1.4 LogD7.2 of some Peptide Drugs. 18 1.5 Prominent Kernel Functions . 32 3.1 Summary of the Synthesized Peptides. 50 3.2 SFM: Chromatographic Settings for Peptide-quantification. 52 3.3 Summary Datasets. 57 4.1 Performances of Baseline Models on the LIPOPEP Set. 64 4.2 Performances of Extended Models and the Consensus Model on the Pooled Data. 73 4.3 Structures and logD7.4 of the Test Compounds Predicted with an Abso- lute Error > 2 log Units. 77 4.4 Results of the Benchmark Analysis . 82 4.5 Summary of the Synthesised and Tested Offsprings . 94 xi List of Abbreviations 1D one-dimensional 2D two-dimensional 3D three-dimensional AA amino acid AAM arithmetic average model ACN acetonitrile ACP anticancer peptide AD applicability domain AE absolute error AMP antimicrobial peptide ANN(-E) artificial neural network (-ensemble) AZ AstraZeneca CCL19, CCL21 CC-chemokine ligand 19/21 CCR7 chemokine receptor 7 CHI chromatographic hydrophobicity index CPC centrifugal partition chromatography CPP cell penetrating peptide CR chemokine receptor CV cross validation DCM dichloromethane DMF dimethylformamide EA evolutionary algorithms ECFP extended connectivity fingerprints EV external validation FA formic acid FDA Food and Drug Administration Fmoc 9-fluorenylmethoxycarbonyl GAG glycosaminoglycans GP Gaussian process GPCR G-protein-coupled receptor HCTU 2-(6-chloro-1H-benzotriazol-1-yl)-1,1,3,3-tetramethylaminium- hexafluorophosphate HPLC high-performance liquid chromatography HTS high-throughput screening IUPAC International Union of Pure and Applied Chemistry Kd dissociation constant Lasso least absolute shrinkage and selection operator LLE llipophilic ligand efficiency logDpH logarithmic distribution coefficient at specific pH logP logarithmic partition coefficient MD molecular dynamics MHC-1 major histocompatibility complex 1 MOE molecular operating environment ML machine learning xii MLR multivariate linear regression MS mass spectrometry MST microscale thermophoresis MW molecular weight NCE new chemical entity NMM n-methyl-morpholine NMR nuclear magnetic resonance NN nearest neighbour OCHEM online chemical modelling environment PB phosphate buffer PBS phosphate-buffered saline PC principal component PCA principal component analysis PD pharmacodynamics PK pharmacokinetics pKa logarithmic acid dissociation constant PPI protein-protein interaction peptide-protein interaction PSGL-1 p-selectin glycoprotein ligand-1 QSAR quantitative structure-activity relationship QSPR quantitative structure-property relationship RF random forest RMSE root mean squared error Ro5 rule of five SFM shake-flask method SMILES simplified molecular input line entry specification S/N signal-to-noise ratio SPPS solid phase peptide synthesis std standard deviation SVM support vector machine SVR support vector regression TFA trifluoroacetic acid TRH thyrotropin-releasing hormone TIS triisopropylsilane UHPLC ultra-high-performance liquid chromatography USP United States Pharmacopeia vdW van der Waals xiii Summary Lipophilicity displays a key physicochemical property in drug design and discov- ery. In early stage scenarios, lipophilicity is employed to rationalise the selection of molecules from a large pool of compounds directing the development into preferred regions of the chemical space. The direct link between lipophilicity and the
Details
-
File Typepdf
-
Upload Time-
-
Content LanguagesEnglish
-
Upload UserAnonymous/Not logged-in
-
File Pages206 Page
-
File Size-