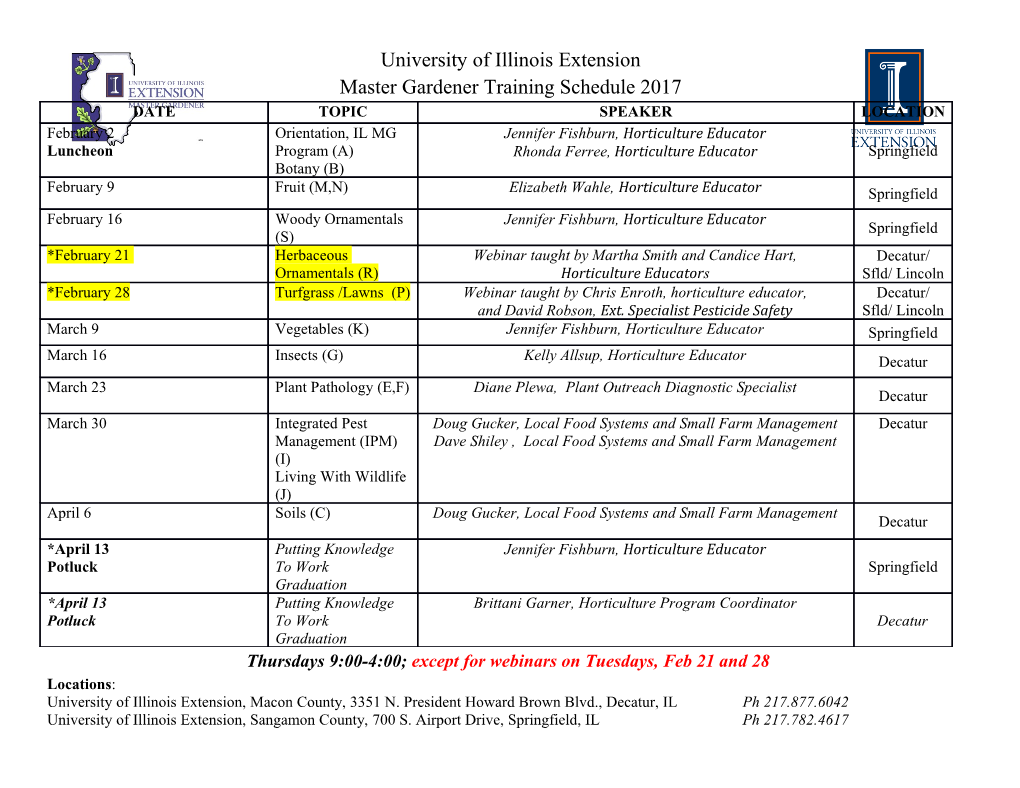
Revista Colombiana de Estadística Diciembre 2013, volumen 36, no. 2, pp. 319 a 336 Bayesian Inference for Two-Parameter Gamma Distribution Assuming Different Noninformative Priors Inferencia Bayesiana para la distribución Gamma de dos parámetros asumiendo diferentes a prioris no informativas Fernando Antonio Moala1;a, Pedro Luiz Ramos1;b, Jorge Alberto Achcar2;c 1Departamento de Estadística, Facultad de Ciencia y Tecnología, Universidade Estadual Paulista, Presidente Prudente, Brasil 2Departamento de Medicina Social, Facultad de Medicina de Ribeirão Preto, Universidade de São Paulo, Ribeirão Preto, Brasil Abstract In this paper distinct prior distributions are derived in a Bayesian in- ference of the two-parameters Gamma distribution. Noniformative priors, such as Jeffreys, reference, MDIP, Tibshirani and an innovative prior based on the copula approach are investigated. We show that the maximal data information prior provides in an improper posterior density and that the different choices of the parameter of interest lead to different reference pri- ors in this case. Based on the simulated data sets, the Bayesian estimates and credible intervals for the unknown parameters are computed and the performance of the prior distributions are evaluated. The Bayesian analysis is conducted using the Markov Chain Monte Carlo (MCMC) methods to generate samples from the posterior distributions under the above priors. Key words: Gamma distribution, noninformative prior, copula, conjugate, Jeffreys prior, reference, MDIP, orthogonal, MCMC. Resumen En este artículo diferentes distribuciones a priori son derivadas en una in- ferencia Bayesiana de la distribución Gamma de dos parámetros. A prioris no informativas tales como las de Jeffrey, de referencia, MDIP, Tibshirani y una priori innovativa basada en la alternativa por cópulas son investigadas. Se muestra que una a priori de información de datos maximales conlleva a una a aProfessor. E-mail: [email protected] bStudent. E-mail: [email protected] cProfessor. E-mail: [email protected] 319 320 Fernando Antonio Moala, Pedro Luiz Ramos & Jorge Alberto Achcar posteriori impropia y que las diferentes escogencias del parámetro de interés permiten diferentes a prioris de referencia en este caso. Datos simulados per- miten calcular las estimaciones Bayesianas e intervalos de credibilidad para los parámetros desconocidos así como la evaluación del desempeño de las distribuciones a priori evaluadas. El análisis Bayesiano se desarrolla usando métodos MCMC (Markov Chain Monte Carlo) para generar las muestras de la distribución a posteriori bajo las a priori consideradas. Palabras clave: a prioris de Jeffrey, a prioris no informativas, conjugada, cópulas, distribución Gamma, MCMC, MDIP, ortogonal, referencia. 1. Introduction The Gamma distribution is widely used in reliability analysis and life testing (see for example, Lawless 1982) and it is a good alternative to the popular Weibull distribution. It is a flexible distribution that commonly offers a good fit to any variable such as in environmental, meteorology, climatology, and other physical situations. Let X be representing the lifetime of a component with a Gamma distribution, denoted by Γ(α, β) and given by βα f(x j α; β) = xα−1 exp{−βxg; for all x > 0 (1) Γ(α) where α > 0 and β > 0 are unknown shape and scale parameters, respectively. There are many papers considering Bayesian inference for the estimation of the Gamma parameters. Son & Oh (2006) assume vague priors for the param- eters to the estimation of parameters using Gibbs sampling. Apolloni & Bassis (2099) compute the joint probability distribution of the parameters without as- suming any prior. They propose a numerical algorithm based on an approximate analytical expression of the probability distribution. Pradhan & Kundu (2011) assume that the scale parameter has a Gamma prior and the shape parameter has any log-concave prior and they are independently distributed. However, most of these papers have in common the use of proper priors and the assumption of independence a priori of the parameters. Although this is not a problem and have been much used in the literature we, would like to propose a noninformative prior for the Gamma parameters which incorporates the dependence structure of parameters. Some of priors proposed in the literature are Jeffreys (1967), MDIP (Zellner 1977, Zellner 1984, Zellner 1990, Tibshirani 1989), and reference prior (Bernardo 1979). Moala (2010) provides a comparison of these priors to estimate the Weibull parameters. Therefore, the main aim of this paper is to present different noninformative priors for a Bayesian estimation of the two-parameter Gamma distribution. We also propose a bivariate prior distribution derived from copula functions (see for example, Nelsen 1999, Trivedi & Zimmer 2005a, Trivedi & Zimmer 2005b) in order to construct a prior distribution to capture the dependence structure between the parameters α and β. Revista Colombiana de Estadística 36 (2013) 319–336 Bayesian Inference for Two-Parameter Gamma Distribution 321 We investigate the performance of the prior distributions through a simulation study using a small data set. Accurate inference for the parameters of the Gamma is obtained using MCMC (Markov Chain Monte Carlo) methods. 2. Maximum Likelihood Estimation Let X1, :::, Xn be a complete sample from (1) then the likelihood function is n n βnα Y n X o L(α; β j x) = xα−1 exp −β x (2) [Γ(α)]n i i i=1 i=1 for α > 0 and β > 0. @ @ Considering @α log L and @β log L equal to 0 and after some algebric manip- ulations we get the likelihood equations given by ! αb X βb = and log αb − (αb) = log ∼ (3) X X 0 @ Γ (k) where (k) = @k log Γ(k)= Γ(k) (see Lawless 1982) is the diGamma function, Pn 1=n i=1 xi Qn X = n and X = i=1 xi . The solutions for these equations provide the maximum likelihood estimators αb and βb for the parameters of the Gamma distribution (1). As closed form solution is not possible to evaluate (3), numerical techniques must used. The Fisher information matrix is given by " 0 1 # (α) − β I(α; β) = 1 α (4) − β β2 where 0(α) is the derivative of (α) called as triGamma function. For large samples, approximated confidence intervals can be constructed for the parameters α and β through normal marginal distributions given by 2 2 αb ∼ N(α; σ1) and βb ∼ N(0; σ2); for n ! 1 (5) β2 ’(α) 2 αb 2 b b where σ1 = var(α) = and σ2 = var(βb) = . In this case, the b b αψb ’(αb)−1 b αψb ’(αb)−1 approximated 100(1−Γ)% confidence intervals for each parameter α and β are given by α − z Γ σ1 < α < α + z Γ σ1 and βb − z Γ σ2 < β < βb + z Γ σ2 (6) b 2 b 2 2 2 respectively. Revista Colombiana de Estadística 36 (2013) 319–336 322 Fernando Antonio Moala, Pedro Luiz Ramos & Jorge Alberto Achcar 3. Jeffrey’s Prior A well-known weak prior to represent a situation with little information about the parameters was proposed by Jeffreys (1967). This prior denoted by πJ (α, β) is derived from the Fisher information matrix I(α, λ) given in (4) as p πJ (α; β) / det I(α, β) (7) Jeffrey’s prior is widely used due to its invariance property under one-to-one transformations of parameters although there has been an ongoing discussion about whether the multivariate form prior is appropriate. Thus, from (4) and (7) the Jeffreys prior for (α, β) parameters is given by: pα 0(α) − 1 π (α; β) / (8) J β 4. Maximal Data Information Prior (MDIP) It is of interest that the data gives more information about the parameter than the information on the prior density; otherwise, there would not be justification for the realization of the experiment. Thus, we wish a prior distribution π(φ) that provides a gain in the information supplied by data in which the largest possible relative to the prior information of the parameter, that is, which maximize the information on the data. With this idea Zellner (1977), Zellner (1984), Zellner (1990) and Min & Zellner (1993) derived a prior which maximize the average information in the data density relative to that one in the prior. Let Z H(φ) = f(x j φ)lnf(x j φ)dx; x 2 Rx (9) Rx be the negative entropy of f(x j φ), the measure of the information in f(x j φ) and Rx the range of density f(x j φ). Thus, the following functional criterion is employed in the MDIP approach: Z b Z b G[π(φ)] = H(φ)π(φ)dφ − π(φ) ln π(φ)dφ (10) a a which is the prior average information in the data density minus the information in R b the prior density. G[π(φ)] is maximized by selection of π(φ) subject to a π(φ)dφ = 1. The solution is then a proper prior given by n o π(φ) = k exp H(φ) a ≤ φ ≤ b (11) −1 R b n o where k = a exp H(φ) dφ is the normalizing constant. Therefore, the MDIP is a prior that leads to an emphasis on the information in the data density or likelihood function. That is, its information is weak in comparison with data information. Revista Colombiana de Estadística 36 (2013) 319–336 Bayesian Inference for Two-Parameter Gamma Distribution 323 Zellner (1977), Zellner (1984), Zellner (1990) shows several interesting proper- ties of MDIP and additional conditions that can also be imposed to the approach reflection given initial information. However, the MDIP has restrictive invariance properties. Theorem 1. Suppose that we do not have much prior information available about α and β. Under this condition, the prior distribution MDIP, denoted by πZ (α, β), for the parameters (α, β) of the Gamma density (1) is given by: β n o π (α; β) / exp (α − 1) (α) − α (12) Z Γ(α) Proof .
Details
-
File Typepdf
-
Upload Time-
-
Content LanguagesEnglish
-
Upload UserAnonymous/Not logged-in
-
File Pages18 Page
-
File Size-