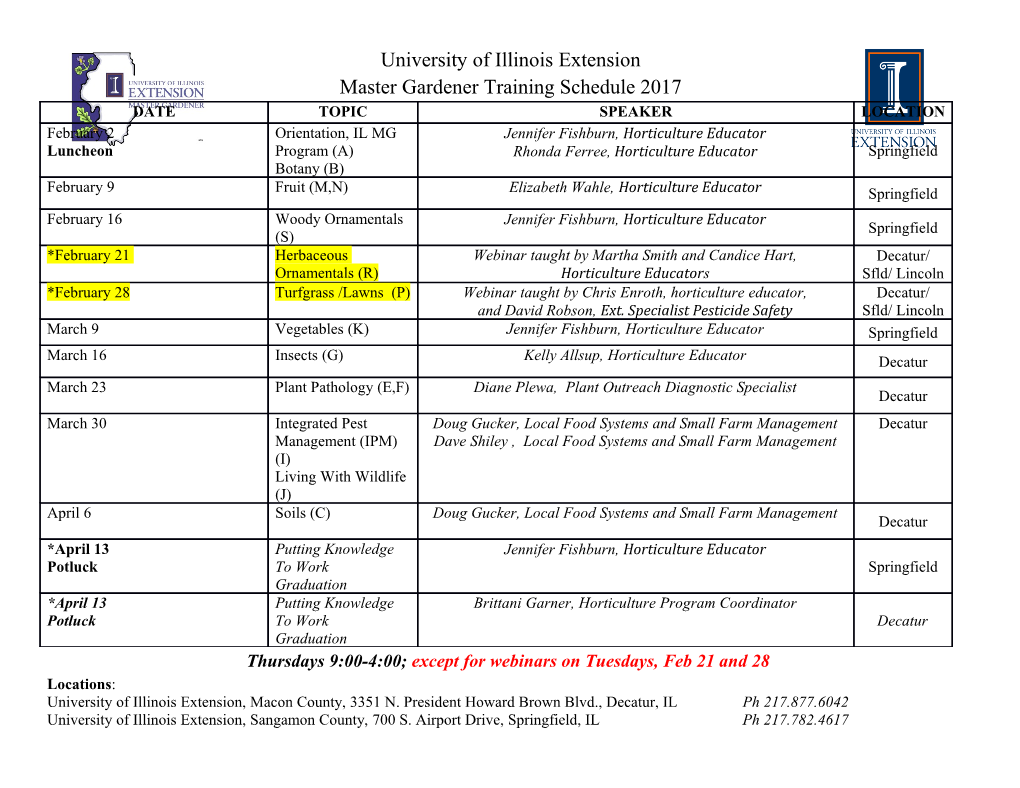
Flattening the Curve: Pandemic-Induced Revaluation of Urban Real Estate Arpit Gupta‡ Vrinda Mittal† Jonas Peeters§ Stijn Van Nieuwerburgh† ‡NYU Stern †Columbia GSB §NYU Data Science Bank of England June 14, 2021 1 / 17 1. Urban Flight Document Population Moves in the time of Covid 2 / 17 Mobility Gradient Shows Urban Flight NYC SF vs. Rent vs. Prices Covid gradient I Covid-19 prompted a large urban flight to suburbs over Feb 2020–Mar 2020 I Venpath cell phone data 0.4 0.2 0.0 0.2 Log Change in Population 0.4 0 1 2 3 4 5 6 Log(1 + Distance) from City Center 3 / 17 2. Change in Bid-Rent Functions Flattening the Curve 4 / 17 NYC Area SF Chicago Log Change Price Log Change Rent Log Change Price 0.10 0.05 0.00 0.05 0.10 0.15 0.20 5 / 17 Rent Changes New York NY Rent Reversal Pre vs. Post I Rents declined drastically in city centers. Grew strongly in suburbs. Dec 2020 8.4 0.15 Dec 2019 0.10 8.2 0.05 8.0 0.00 7.8 0.05 Log Rent 7.6 Change in Log Rent 0.10 7.4 0.15 7.2 0.20 0 1 2 3 4 5 0 1 2 3 4 Log(1 + Distance) from New York Center Log(1 + Distance) from New York City Center 6 / 17 Rent Changes San Francisco Dec 2020 8.6 Dec 2019 0.05 0.00 8.4 0.05 8.2 0.10 Log Rent 8.0 Change in Log Rent 0.15 7.8 0.20 7.6 0 1 2 3 4 0 1 2 3 4 Log(1 + Distance) from San Francisco Center Log(1 + Distance) from San Francisco Center 7 / 17 Complete Reversal of Rent Gradient Top 30 MSAs Top 49 MSAs NYC SF LA z m ln Rentijt = αjt + δjt ln(1 + D(zij ; zj )) + βXij + eijt 0.000 -0.010 -0.020 -0.030 Gradient elasticity w.r.t. zori Gradient elasticity w.r.t. -0.040 -0.050 2018m1 2018m7 2019m1 2019m7 2020m1 2020m7 Month Estimates Bounds 8 / 17 Complete Reversal of Rent Gradient Top 30 MSAs Top 49 MSAs NYC SF LA z m ln Rentijt = αjt + δjt ln(1 + D(zij ; zj )) + βXij + eijt 0.000 -0.010 -0.020 -0.030 Gradient elasticity w.r.t. zori Gradient elasticity w.r.t. -0.040 -0.050 2018m1 2018m7 2019m1 2019m7 2020m1 2020m7 Month Estimates Bounds 8 / 17 Price Changes New York and San Francisco NY Price Reversal I Prices also saw stronger growth in suburbs as seen in New York (left) and San Francisco (right). 0.20 0.15 0.15 0.10 0.10 0.05 0.05 0.00 0.00 Change in Log Price Change in Log Price 0.05 0.05 0.10 0.10 0 1 2 3 4 5 0 1 2 3 4 Log(1 + Distance) from New York City Center Log(1 + Distance) from San Francisco Center 9 / 17 Partial Reversal of Price Gradient Top 30 MSAs Top 49 MSAs NYC SF LA z m ln Priceijt = αjt + δjt ln(1 + D(zij ; zj )) + βXij + eijt -0.100 -0.110 -0.120 -0.130 Gradient elasticity w.r.t. zhvi Gradient elasticity w.r.t. -0.140 2018m1 2018m7 2019m1 2019m7 2020m1 2020m7 Month Estimates Bounds 10 / 17 4. Explaining Changes in Real Estate Gradients Urban Premia Reverses in Presence of Remote Work and Land Scarcity 11 / 17 National Change in Price Gradients 12 / 17 Correlation with Working from Home Rent Price WA NY-NJ-PA (SF)-CA (SF)-CA .04 .05 (Pittsburgh)-PAIL-IN-WI NY-NJ-PA AZ WA MN-WI MA-NH NC-SC DC-VA-MD-WV GA (Sacramento)-CA(Austin)-TX (Houston)-TX(Dallas)-TX(LA)-CACO .02 (Portland)-OR-WA (Miami)-FL MA-NH (Riverside)-CA (Orlando)-FL MO-IL(Tampa)-FL(Portland)-OR-WA (Miami)-FLMO-IL (Sacramento)-CACO DC-VA-MD-WV (San (Baltimore)-MDDiego)-CA (Las Vegas)-NV (Tampa)-FL(Baltimore)-MD (Las Vegas)-NV GAIL-IN-WI(LA)-CAMN-WI PA-NJ-DE-MD NC-SC 0 0 (San Antonio)-TX (Dallas)-TXPA-NJ-DE-MD (Riverside)-CA (Orlando)-FL (San Diego)-CA (Houston)-TX (San Antonio)-TX (Austin)-TX (Cincinnati)-OH-KY-IN MI AZ Gradient Change (ZHVI) Gradient Change(ZORI) -.02 (Pittsburgh)-PA (Cincinnati)-OH-KY-IN -.05 MI -.04 .3 .35 .4 .45 .3 .35 .4 .45 Dingel Neiman WFH Dingel Neiman WFH MSAs Fitted Values MSAs Fitted Values WFH = Dingle and Neiman (2020) measure of ability to work from home, based on detailed job descriptions 13 / 17 Explaining the Variation in Rent Gradient Changes MSA level ∆δj = α + β1WFH + β2Policy Stringency + β3Supply Inelasticity + "j |{z} Change in Rent Gradient ∗∗∗ ∗∗ ∗∗∗ Work from Home 0.326 0.267 0.326 (0.101) (0.112) (0.102) ∗∗ Stringency Measure 0.145 0.0623 (0.0651) (0.0766) ∗ Supply Inelasticity Index 0.0300 0.00862 (0.0172) (0.0188) Orthogonalized Stringency Index 0.0797 (0.0664) Orthogonalized Supply Inelasticity 0.00862 (0.0188) Observations 30 30 30 30 30 2 R 0.270 0.151 0.098 0.314 0.314 14 / 17 j Backing out Expected Rent Growth, Case 1: ∆x = 0 Full Table 4.00 3.00 2.00 1.00 Urban Minus Suburban Rent Growth 0.00 0 5 10 15 20 Time Transitory Permanent Combined (p=0.64) 15 / 17 Concluding Thoughts I Pandemic-triggered urban flight has benefited the suburban real estate sector, hurt the urban core. I Work from home opportunities explain much of the disappearance of urban rent premium. I House prices and rents suggest that much, but not all, of the WFH phenomenon is expected to be transitory. Urban rent revival predicted and already underway. I How this plays out will affect housing affordability debate and fiscal health of (superstar) cities. I Follow-up work: Implications for urban office. 16 / 17 Urban Office Leases: Net Effective Rent I Study new office leases in New York City using Compstak data I Net effective rent in $/sf (combines asking rent, free rent, TI) l_log_renteff - Model 1 70 65 60 55 50 Fixed Effect 45 40 35 2016-01 2016-07 2017-01 2017-07 2018-01 2018-07 2019-01 2019-07 2020-01 2020-07 Month 17 / 17 Are Cities Dead, or is this a Temporary Blip for Urban Growth? Ed Glaeser: “We’ll eventually figure out how to deal with COVID-19. And the forces that gave rise to superstar cities aren’t going to just go away. Zoom calls remain a lackluster substitute for face-to- face chats, and people will still want all the awesome stuff that density creates.” 18 / 17 Are Cities Dead, or is this a Temporary Blip for Urban Growth? David Autor: “The COVID crisis appears poised to reshape labor markets along at least four axes: telepresence, urban de- densification, employment concentration in large firms, and general automation... The pandemic will permanently alter the texture of urban life.” 18 / 17 1. Document disappearance of rent gradient, partial decline in price gradient I Associated with large urban flight 2. Evaluate associations for changing price and rent gradients I Working from home is the key driver I Strong results for rent gradients implying shock is transitory 3. Extract housing market expectations of future urban rent premia I Use Campbell-Shiller decomposition of prices I Use survey expectations data from Pulsenomics to calibrate persistence I Price gradient falls less than rent gradient ! future urban rent growth Pandemic was a massive temporary shock, but does not appear to herald the permanent demise of the superstar city Large, But Temporary, Decline in (Superstar) City Premia I Focus on bid-rent function (or price and rent gradients) I Changes in house prices/rents with respect to distance from center of the city I Urban premia reflects agglomeration effects, commuting, and amenities 19 / 17 2. Evaluate associations for changing price and rent gradients I Working from home is the key driver I Strong results for rent gradients implying shock is transitory 3. Extract housing market expectations of future urban rent premia I Use Campbell-Shiller decomposition of prices I Use survey expectations data from Pulsenomics to calibrate persistence I Price gradient falls less than rent gradient ! future urban rent growth Pandemic was a massive temporary shock, but does not appear to herald the permanent demise of the superstar city Large, But Temporary, Decline in (Superstar) City Premia I Focus on bid-rent function (or price and rent gradients) I Changes in house prices/rents with respect to distance from center of the city I Urban premia reflects agglomeration effects, commuting, and amenities 1. Document disappearance of rent gradient, partial decline in price gradient I Associated with large urban flight 19 / 17 3. Extract housing market expectations of future urban rent premia I Use Campbell-Shiller decomposition of prices I Use survey expectations data from Pulsenomics to calibrate persistence I Price gradient falls less than rent gradient ! future urban rent growth Pandemic was a massive temporary shock, but does not appear to herald the permanent demise of the superstar city Large, But Temporary, Decline in (Superstar) City Premia I Focus on bid-rent function (or price and rent gradients) I Changes in house prices/rents with respect to distance from center of the city I Urban premia reflects agglomeration effects, commuting, and amenities 1. Document disappearance of rent gradient, partial decline in price gradient I Associated with large urban flight 2.
Details
-
File Typepdf
-
Upload Time-
-
Content LanguagesEnglish
-
Upload UserAnonymous/Not logged-in
-
File Pages80 Page
-
File Size-