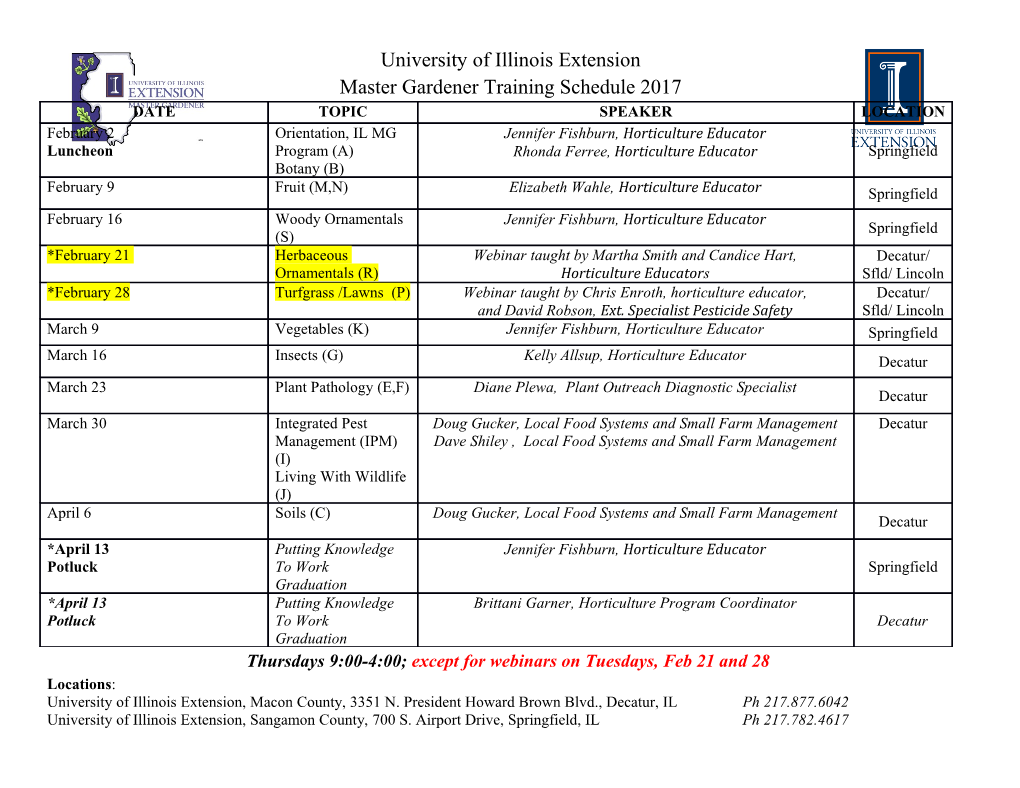
ARTICLE https://doi.org/10.1038/s41467-021-21194-4 OPEN RNA secondary structure prediction using deep learning with thermodynamic integration ✉ Kengo Sato 1 , Manato Akiyama1 & Yasubumi Sakakibara1 Accurate predictions of RNA secondary structures can help uncover the roles of functional non-coding RNAs. Although machine learning-based models have achieved high performance in terms of prediction accuracy, overfitting is a common risk for such highly parameterized fi 1234567890():,; models. Here we show that over tting can be minimized when RNA folding scores learnt using a deep neural network are integrated together with Turner’s nearest-neighbor free energy parameters. Training the model with thermodynamic regularization ensures that folding scores and the calculated free energy are as close as possible. In computational experiments designed for newly discovered non-coding RNAs, our algorithm (MXfold2) achieves the most robust and accurate predictions of RNA secondary structures without sacrificing computational efficiency compared to several other algorithms. The results sug- gest that integrating thermodynamic information could help improve the robustness of deep learning-based predictions of RNA secondary structure. 1 Department of Biosciences and Informatics, Keio University, 3–14–1 Hiyoshi, Kohoku-ku, Yokohama, Japan. ✉email: [email protected] NATURE COMMUNICATIONS | (2021) 12:941 | https://doi.org/10.1038/s41467-021-21194-4 | www.nature.com/naturecommunications 1 ARTICLE NATURE COMMUNICATIONS | https://doi.org/10.1038/s41467-021-21194-4 ecent studies have revealed that functional non-coding ContextFold14, and other methods, and has enabled us to more RNAs (ncRNAs) play essential roles, including transcrip- accurately predict RNA secondary structures. However, rich para- R fi fi tional regulation and guiding modi cation, participating in meterization can easily cause over tting to the training data, thus various biological processes ranging from development to cell preventing robust predictions for a wide range of RNA sequences15. differentiation, with defective functionality being involved in Probabilistic generative models such as stochastic context-free various diseases1. Because it is well-known that the functions of grammars (SCFGs) have also been applied to predicting RNA ncRNAs are deeply related to their structures rather than their secondary structures. Recently, TORNADO15 implemented an primary sequences, discovering the structures of ncRNAs can application of SCFGs to the nearest-neighbor model, thus achieving elucidate the functions of ncRNAs. However, there are major performance comparable with that of other machine learning-based difficulties in determining RNA tertiary structures through methods. experimental assays such as nuclear magnetic resonance and X- Hybrid methods that combine thermodynamic and machine ray crystal structure analysis, because of the high experimental learning-based approaches to compensate for each other’s costs and resolution limits on measurements of RNA. Although shortcomings have been developed. SimFold16,17 more accurately considerable advances in cryo-electron microscopy research on estimates thermodynamic parameters from training data, RNA tertiary structure determination have been achieved in including RNA sequences and their known secondary structures, recent years2, these limitations have not yet been completely as well as the free energy of their known secondary structures. We overcome. Therefore, instead of conducting such experimental previously proposed MXfold18, which combines thermodynamic assays, we frequently perform computational prediction of RNA energy parameters and rich-parameterized weight parameters. secondary structures, defined as sets of base-pairs with hydrogen The model learns more precise parameters for substructures bonds between the nucleotides. observed in training data and avoids overfitting rich- The most popular approach for predicting RNA secondary parameterized weight parameters to the training data by resort- structures is based on thermodynamic models, such as Turner’s ing to the thermodynamic parameters for assessing previously nearest-neighbor model3,4, in which a secondary structure is unobserved substructures. decomposed into several characteristic substructures, called In recent years, deep learning has made remarkable progress in nearest-neighbor loops, such as hairpin loops, internal loops, a wide range of fields, including bioinformatics and life sciences. bulge loops, base-pair stackings, multi-branch loops, and external SPOT-RNA19 and E2Efold20 have been developed for RNA sec- loops, as shown in Fig. 1. The free energy of each nearest- ondary structure prediction using deep neural networks (DNNs). neighbor loop can be calculated by summing the free energy Both algorithms formulate RNA secondary structure prediction parameters that characterize the loop. The free energy parameters as multiple binary classification problems that predict whether have been determined in advance by experimental methods such each pair of nucleotides forms a base pair or not, using a deep as optical melting experiments3. The free energy of an entire RNA neural network trained with a large amount of training data. As secondary structure is calculated by summing the free energy of with the other machine learning-based methods described above, the decomposed nearest-neighbor loops. We can efficiently cal- concerns about overfitting caused by rich parameterization culate an optimal secondary structure that has the minimum free remain. energy using a dynamic programming (DP) technique, the well- Inspired by MXfold and the DNN-based RNA secondary known Zuker algorithm5. A number of tools, including Mfold/ structure prediction methods, in this paper, we propose an UNAfold6,7, RNAfold8,9, and RNAstructure10,11, have adopted algorithm for predicting RNA secondary structures using deep this approach. learning. Similar to MXfold, we integrate folding scores, which An alternative approach utilizes machine learning techniques, are calculated by a deep neural network, with Turner’s nearest- which train scoring parameters for decomposed substructures from neighbor free energy parameters. The deep neural network is reference structures, rather than experimental techniques. This trained using the max-margin framework with thermodynamic approach has successfully been adopted by CONTRAfold12,13, regularization, which is proposed in this paper to make our model robust by maintaining that the folding score calculated by our method and the free energy calculated by the thermodynamic A 40 fi G G multi-branch loop parameters are as close as possible. To con rm the robustness of U U A U C our algorithm, we conducted computational experiments with C 50 U U G U C two types of cross-validation: sequence-wise cross-validation, G G U U U U U G G U bulge loop with test datasets structurally similar to training datasets, and A C 30 C G family-wise cross-validation, with test datasets structurally dis- A C U G A U U U C similar to training datasets. The resulting method, called 60 G G G MXfold2, achieved the best prediction accuracy based not only on internal loop A G U A C stacking U the sequence-wise cross-validation, but also on the family-wise 20 A G U U cross-validation, indicating the robustness of our algorithm. In 10 A C G addition, the computational experiment conducted on a dataset A U hairpin loop A A U C C with sequence-structure-energy triplets shows a high correlation C G C C 1 66 between folding scores predicted by MXfold2 and the free energy A U derived from optical melting experiments. external loop Fig. 1 Decomposition of an RNA secondary structure into nearest- Results neighbor loops. An RNA secondary structure can be decomposed into Overview of our algorithm. Our algorithm computes four types several types of nearest-neighbor loops, including hairpin loops (e.g., bases of folding scores for each pair of nucleotides by using a deep 11–19), internal loops (e.g., bases 25–29 and 43–47), bulge loops (e.g., neural network, as shown in Fig. 2. The folding scores are used to bases 4–5 and 57–62), base-pair stackings (e.g., bases 23–24 and 48–49), calculate scores of the nearest-neighbor loops. Similar to MXfold, multi-branch loops (e.g., bases 7–9, 21–23, and 49–55), and external loops we integrate the folding scores, which are calculated using a deep (e.g., bases 1–2 and 64–66). This diagram was drawn using VARNA45. neural network, with Turner’s nearest-neighbor free energy 2 NATURE COMMUNICATIONS | (2021) 12:941 | https://doi.org/10.1038/s41467-021-21194-4 | www.nature.com/naturecommunications NATURE COMMUNICATIONS | https://doi.org/10.1038/s41467-021-21194-4 ARTICLE RNA sequence 3-layer MLP L x d L x d Act. / norm. / dropout L Concat 2D d/2 d/2 L L x L x 4 1D conv@5 Act. / norm. / dropout Helix Stacking Unpaired Region Block 1 i+1 j-1 1D conv@3 L x L x d Act. / norm. / dropout i j ij 2D conv@5x5 Helix Opening Helix Closing Act. / norm. / dropout Block 1 x (N1-1) i+1 j-1 i+1 j-1 i j i j Block 3 BiLSTM 2D conv@3x3 Act. / norm. / dropout Act. / norm. / dropout Block 2 Folding scores for (i, j) Block 2 x (N2-1) Block 3 x (N3-1) Zuker-style Dynamic Programming Fig. 2 The network structure of our algorithm. It calculates four types of folding scores for each pair of nucleotides (i, j) from a given RNA sequence of length L. See the “Methods” section for more details. Act.: the activation function, Norm.: the normalization function, Conv: the convolution layer, d: the dimension of trainable embeddings, N1, N2, and N3: the numbers of times Blocks 1, 2, and 3 repeat, respectively, BiLSTM: bidirectional long short-term memory, MLP: multilayer perceptron, and Concat 2D: concatenation of 1D sequences to 2D matrices. parameters. Then, our algorithm predicts an optimal secondary Table 1 Comparison of the accuracy of the secondary structure that maximizes the sum of the scores of the nearest- 5 structure prediction between the base model and the use of neighbor loops using Zuker-style dynamic programming (DP) .
Details
-
File Typepdf
-
Upload Time-
-
Content LanguagesEnglish
-
Upload UserAnonymous/Not logged-in
-
File Pages9 Page
-
File Size-